We, humans, are very perfect at applying the transfer of knowledge between tasks. This means that whenever we encounter a new problem or a task, we recognize it and apply our relevant knowledge from our previous learning experiences. This makes our work easy and fast to finish. For instance, if you know how to ride a bicycle and if you are asked to ride a motorbike which you have never done before. In such a case, our experience with a bicycle will come into play and handle tasks like balancing the bike, steering, etc. This will make things easier compared to a complete beginner. Such learnings are very useful in real life as they make us more perfect and allow us to earn more experience. Following the same approach, a term was introduced Transfer Learning in the field of machine learning. This approach involves the use of knowledge that was learned in some tasks and applying it to solve the problem in the related target task. While most machine learning is designed to address a single task, the development of algorithms that facilitate transfer learning is a topic of ongoing interest in the machine-learning community.
What is Transfer Learning?
Transfer learning is a technique in machine learning where a model trained on one task is used as the starting point for a model on a second task. This can be useful when the second task is similar to the first task, or when there is limited data available for the second task. By using the learned features from the first task as a starting point, the model can learn more quickly and effectively on the second task. This can also help to prevent overfitting, as the model will have already learned general features that are likely to be useful in the second task.
Why do we need Transfer Learning?
Many deep neural networks trained on images have a curious phenomenon in common: in the early layers of the network, a deep learning model tries to learn a low level of features, like detecting edges, colours, variations of intensities, etc. Such kind of features appear not to be specific to a particular dataset or a task because no matter what type of image we are processing either for detecting a lion or car. In both cases, we have to detect these low-level features. All these features occur regardless of the exact cost function or image dataset. Thus, learning these features in one task of detecting lions can be used in other tasks like detecting humans.
How does Transfer Learning work?
This is a general summary of how transfer learning works:
- Pre-trained Model: Start with a model that has previously been trained for a certain task using a large set of data. Frequently trained on extensive datasets, this model has identified general features and patterns relevant to numerous related jobs.
- Base Model: The model that has been pre-trained is known as the base model. It is made up of layers that have utilized the incoming data to learn hierarchical feature representations.
- Transfer Layers: In the pre-trained model, find a set of layers that capture generic information relevant to the new task as well as the previous one. Because they are prone to learning low-level information, these layers are frequently found near the top of the network.
- Fine-tuning: Using the dataset from the new challenge to retrain the chosen layers. We define this procedure as fine-tuning. The goal is to preserve the knowledge from the pre-training while enabling the model to modify its parameters to better suit the demands of the current assignment.
The Block diagram is shown below as follows:
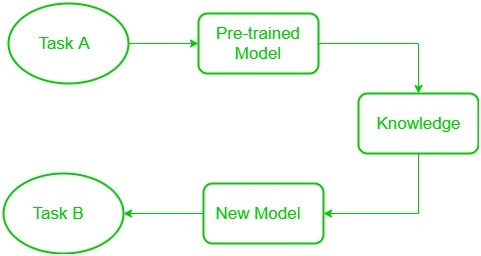
Transfer Learning
Low-level features learned for task A should be beneficial for learning of model for task B.
This is what transfer learning is. Nowadays, it is very hard to see people training whole convolutional neural networks from scratch, and it is common to use a pre-trained model trained on a variety of images in a similar task, e.g models trained on ImageNet (1.2 million images with 1000 categories) and use features from them to solve a new task. When dealing with transfer learning, we come across a phenomenon called the freezing of layers. A layer, it can be a CNN layer, hidden layer, a block of layers, or any subset of a set of all layers, is said to be fixed when it is no longer available to train. Hence, the weights of freeze layers will not be updated during training. While layers that are not frozen follows regular training procedure. When we use transfer learning in solving a problem, we select a pre-trained model as our base model. Now, there are two possible approaches to using knowledge from the pre-trained model. The first way is to freeze a few layers of the pre-trained model and train other layers on our new dataset for the new task. The second way is to make a new model, but also take out some features from the layers in the pre-trained model and use them in a newly created model. In both cases, we take out some of the learned features and try to train the rest of the model. This makes sure that the only feature that may be the same in both of the tasks is taken out from the pre-trained model, and the rest of the model is changed to fit the new dataset by training.
Freezed and Trainable Layers:
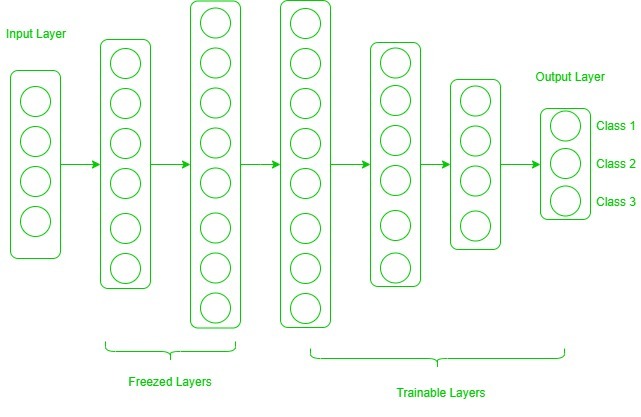
Now, one may ask how to determine which layers we need to freeze, and which layers need to train. The answer is simple, the more you want to inherit features from a pre-trained model, the more you have to freeze layers. For instance, if the pre-trained model detects some flower species and we need to detect some new species. In such a case, a new dataset with new species contains a lot of features similar to the pre-trained model. Thus, we freeze less number of layers so that we can use most of its knowledge in a new model. Now, consider another case, if there is a pre-trained model which detects humans in images, and we want to use that knowledge to detect cars, in such a case where the dataset is entirely different, it is not good to freeze lots of layers because freezing a large number of layers will not only give low level features but also give high-level features like nose, eyes, etc which are useless for new dataset (car detection). Thus, we only copy low-level features from the base network and train the entire network on a new dataset.
Let’s consider all situations where the size and dataset of the target task vary from the base network.
- The target dataset is small and similar to the base network dataset: Since the target dataset is small, that means we can fine-tune the pre-trained network with the target dataset. But this may lead to a problem of overfitting. Also, there may be some changes in the number of classes in the target task. So, in such a case we remove the fully connected layers from the end, maybe one or two, and add a new fully connected layer satisfying the number of new classes. Now, we freeze the rest of the model and only train newly added layers.
- The target dataset is large and similar to the base training dataset: In such cases when the dataset is large, and it can hold a pre-trained model there will be no chance of overfitting. Here, also the last full-connected layer is removed, and a new fully-connected layer is added with the proper number of classes. Now, the entire model is trained on a new dataset. This makes sure to tune the model on a new large dataset keeping the model architecture the same.
- The target dataset is small and different from the base network dataset: Since the target dataset is different, using high-level features of the pre-trained model will not be useful. In such a case, remove most of the layers from the end in a pre-trained model, and add new layers a satisfying number of classes in a new dataset. This way we can use low-level features from the pre-trained model and train the rest of the layers to fit a new dataset. Sometimes, it is beneficial to train the entire network after adding a new layer at the end.
- The target dataset is large and different from the base network dataset: Since the target network is large and different, the best way is to remove the last layers from the pre-trained network and add layers with a satisfying number of classes, then train the entire network without freezing any layer.
Transfer learning is a very effective and fast way, to begin with, a problem. It gives the direction to move, and most of the time best results are also obtained by transfer learning.
Below is the sample code using Keras for Transfer learning & fine-tuning with a custom training loop.
Transfer Learning Implementations
Pre-requisites for implementing the code:
Before going for the implementing the code you have to install some libraries given below:
TensorFlow is an open-source framework that is used for Machine Learning. It provides a range of functions to achieve complex functionalities with single lines of code.
!pip install tensorflow
Import the necessary libraries and functions
Import required libraries and the MNIST dataset, a dataset of handwritten digits often used for training and testing machine learning models.
Python3
import tensorflow as tf
from tensorflow.keras import layers, models, optimizers
from tensorflow.keras.datasets import mnist
from tensorflow.keras.utils import to_categorical
|
Load and unpack the MNIST dataset into training and testing sets for images (x) and labels (y).
Python3
(x_train, y_train), (x_test, y_test) = mnist.load_data()
x_train = x_train.astype( 'float32' ) / 255.0
x_test = x_test.astype( 'float32' ) / 255.0
|
Convert class labels to one-hot encoded vectors for both training and testing sets.
Python3
y_train = to_categorical(y_train, 10 )
y_test = to_categorical(y_test, 10 )
|
Load a pretrained MobileNetV2 model without the classification layer
The code initializes a MobileNetV2 model using TensorFlow/Keras. It has an input shape of (224, 224, 3), excludes the top layers for feature extraction, and uses pre-trained ImageNet weights. This makes it suitable for tasks like transfer learning in image classification.
Python3
base_model = tf.keras.applications.MobileNetV2(input_shape = ( 224 , 224 , 3 ),
include_top = False ,
weights = 'imagenet' )
|
Add custom layers on top of the pre-trained model
Using TensorFlow’s Keras API, this code builds a convolutional neural network (CNN). Layers for reshaping, convolution, pooling, flattening, and fully connected operations are included. Dropout is used to achieve regularisation. Using softmax activation, the model, which is ideal for image classification like MNIST, generates class probabilities. The design achieves a compromise between feature extraction and categorization, allowing for successful learning and generalization.
Python3
model = models.Sequential()
model.add(layers.Reshape(( 28 , 28 , 1 ), input_shape = ( 28 , 28 )))
model.add(layers.Conv2D( 32 , ( 3 , 3 ), activation = 'relu' ))
model.add(layers.MaxPooling2D(( 2 , 2 )))
model.add(layers.Flatten())
model.add(layers.Dense( 256 , activation = 'relu' ))
model.add(layers.Dropout( 0.5 ))
model.add(layers.Dense( 10 , activation = 'softmax' ))
|
Freeze the pretrained layers
It is essential that the convolutional base be frozen prior to model compilation and training. Freezing ( through layer setting).trainable = False) stops a layer’s weights from changing while it is being trained. Since there are numerous layers in MobileNet V2, all of them will be frozen if the trainable flag for the model is set to False.
Python3
for layer in base_model.layers:
layer.trainable = False
|
Compile the model
It is essential to utilize a lower learning rate at this point because you are training a much larger model and want to readjust the pretrained weights. If not, your model may rapidly become overfit.
Python3
model. compile (optimizer = optimizers.Adam(learning_rate = 0.001 ),
loss = 'categorical_crossentropy' ,
metrics = [ 'accuracy' ])
|
Custom training loop
This TensorFlow script trains a neural network over multiple epochs. It uses a training dataset (train_dataset
) and a validation dataset (val_dataset
). The training loop computes gradients of categorical cross-entropy loss and updates the model’s weights using the Adam optimizer. After each epoch, a validation loop calculates and prints the validation accuracy. There’s a minor correction: the optimizer is initialized outside the training loop for proper functionality.
Python3
epochs = 10
batch_size = 32
train_dataset = tf.data.Dataset.from_tensor_slices((x_train, y_train)).shuffle( 60000 ).batch(batch_size)
val_dataset = tf.data.Dataset.from_tensor_slices((x_test, y_test)).batch(batch_size)
for epoch in range (epochs):
print (f "Epoch {epoch + 1}/{epochs}" )
for images, labels in train_dataset:
with tf.GradientTape() as tape:
predictions = model(images)
loss = tf.keras.losses.categorical_crossentropy(labels, predictions)
gradients = tape.gradient(loss, model.trainable_variables)
optimizer = optimizers.Adam(learning_rate = 0.001 )
optimizer.apply_gradients( zip (gradients, model.trainable_variables))
accuracy = tf.metrics.CategoricalAccuracy()
for images, labels in val_dataset:
predictions = model(images)
accuracy.update_state(labels, predictions)
val_acc = accuracy.result().numpy()
print (f "Validation Accuracy: {val_acc}" )
|
Output:
Epoch 1/10
Validation Accuracy: 0.972000002861023
Epoch 2/10
Validation Accuracy: 0.9700999855995178
Epoch 3/10
Validation Accuracy: 0.951200008392334
Epoch 4/10
Validation Accuracy: 0.9736999869346619
Epoch 5/10
Validation Accuracy: 0.9782000184059143
Epoch 6/10
Validation Accuracy: 0.9800000190734863
Epoch 7/10
Validation Accuracy: 0.9787999987602234
Epoch 8/10
Validation Accuracy: 0.9740999937057495
Epoch 9/10
Validation Accuracy: 0.9782999753952026
Epoch 10/10
Validation Accuracy: 0.9786999821662903
Evaluate the model performance on test set
Python3
test_loss, test_accuracy = model.evaluate(x_test, y_test)
print (f "Test Accuracy: {test_accuracy}" )
|
Output:
313/313 [==============================] - 5s 13ms/step - loss: 0.4465 - accuracy: 0.9787
Test Accuracy: 0.9786999821662903
Advantages of transfer learning:
- Speed up the training process: By using a pre-trained model, the model can learn more quickly and effectively on the second task, as it already has a good understanding of the features and patterns in the data.
- Better performance: Transfer learning can lead to better performance on the second task, as the model can leverage the knowledge it has gained from the first task.
- Handling small datasets: When there is limited data available for the second task, transfer learning can help to prevent overfitting, as the model will have already learned general features that are likely to be useful in the second task.
Disadvantages of transfer learning:
- Domain mismatch: The pre-trained model may not be well-suited to the second task if the two tasks are vastly different or the data distribution between the two tasks is very different.
- Overfitting: Transfer learning can lead to overfitting if the model is fine-tuned too much on the second task, as it may learn task-specific features that do not generalize well to new data.
- Complexity: The pre-trained model and the fine-tuning process can be computationally expensive and may require specialized hardware.
Frequently Asked Questions:
1. What is transfer learning?
In simple terms, transfer learning is a technique where we use a pre-trained model which are trained on a sufficient amount of dataset to perform on a similar task that is related to what it was trained on initially.
2. What is the role of transfer learning in natural language processing?
In Natural Language Processing (NLP), transfer learning refers to using huge text corpora with pre-trained language models. Subsequent NLP tasks benefit from these acquired characteristics by employing models such as BERT or GPT, which learn contextual representations. This method speeds up model training, decreases the requirement for large task-specific datasets, and enhances performance. In NLP, transfer learning has emerged as a key component that supports advances in sentiment analysis, text categorization, and language understanding, among other language-related tasks.
3. Define the transfer learning in layman terms?
Transfer learning in layman terms can be defined like if you learn to ride a bicycle, you can apply some of that skill when learning to ride a new type of bike. Similarly, in transfer learning, a pre-trained model is used as a starting point for a new task that shares similarities with the original task it was trained on.
4. What is fine-tuning in Transfer Learning?
Fine-tuning in transfer learning refers to the process of taking a pre-trained model on one task and further training it on a new, specific task. Initially, the model is trained on a large dataset for a general task, learning useful features. In fine-tuning, some layers of the pre-trained model may be kept frozen (not updated) to retain previously learned knowledge, while others are adjusted to adapt to the nuances of the new task. This approach is particularly beneficial when the new task has a smaller dataset, allowing the model to specialize without requiring extensive training from scratch.
5. What is freezed and trainable layers in Transfer learning?
In transfer learning, “frozen” layers refer to pre-trained layers whose weights remain fixed during subsequent training, preserving learned features. These layers are not updated to prevent loss of valuable knowledge. In contrast, “trainable” layers are those modified and fine-tuned on the new task, allowing the model to adapt to task-specific patterns. Freezing early layers, where generic features are learned, is common, while later layers may be fine-tuned. This strategy balances leveraging pre-existing knowledge and tailoring the model for specific tasks, optimizing performance when labeled data for the new task is limited.
Share your thoughts in the comments
Please Login to comment...