Emotion Based Music Player – Python Project
Last Updated :
03 Aug, 2023
In this article, we will be discussing how can we recommend music based on expressions or say dominant expressions on someone’s face. This is a basic project in which we will be using OpenCV, Matplotlib, DeepFace, and Spotify API.
Import Packages and Modules
- OpenCV: OpenCV is a Python open-source package used for computer vision, machine learning, and image processing.
- Matplotlib: Python’s Matplotlib module is a complete tool for building static, animated, and interactive visualizations.
- Deepface: A Facebook research team specializing in artificial intelligence developed Deepface. Python provides a framework for analyzing properties and recognizing faces. Keras and TensorFlow both utilize key library elements from Deepface.
- Requests: Sending HTTP/1.1 requests is incredibly simple with Requests. These days, all you have to do is use the JSON method instead of manually adding query strings to your URLs or form-encoding your PUT and POST data.
Though there are many other ways to recommend music, this implementation is a basic approach. Let’s move forward to the implementation. Install the deepface library in the Python environment.
Python3
!pip install - q deepface
|
Import the necessary packages.
Python3
import cv2
import requests
import matplotlib.pyplot as plt
from deepface import DeepFace
|
The path of the image whose expression detection is to be performed should be copied. The image should then be read using the “imread()” method in cv2, the image is stored in the form of an array. Afterward, utilize Matplotlib’s imshow() method to display the image.
Python3
img = cv2.imread( "sample.jpg" )
plt.imshow(img[:, :, :: - 1 ])
plt.show()
|
Output:
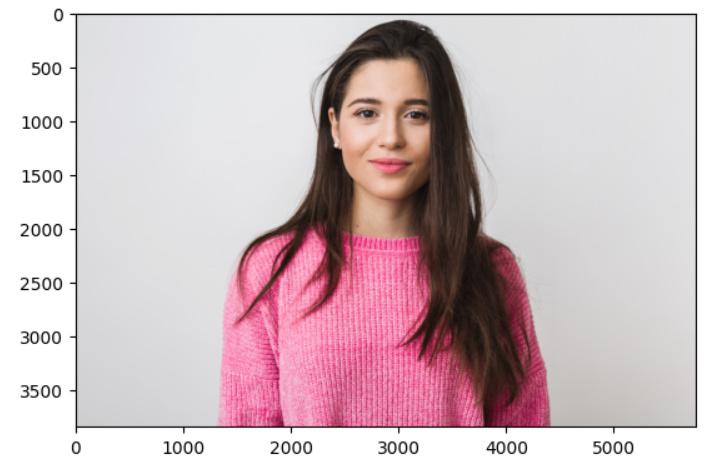
A Sample image with happy emotion on the face
Recognizing Emotion using DeepFace
Use deepface to analyze the emotion in an image. Pass the image stored as an array to Deepface’s analyze function. It will return a Python dictionary with the percentage of all emotions.
Python3
result = DeepFace.analyze(img, actions = [ 'emotion' ])
print (result)
|
Output:
[{'emotion': {'angry': 2.9941825391265356e-05,
'disgust': 3.6047339119136895e-10,
'fear': 0.00011003920865101386,
'happy': 97.65191646241146,
'sad': 0.0015582609232700413,
'surprise': 0.0032574247843123716,
'neutral': 2.343132812456228},
'dominant_emotion': 'happy',
'region': {'x': 325, 'y': 64, 'w': 128, 'h': 128}}]
Extract the emotion with the highest percentage.
Python3
query = str ( max ( zip (result[ 0 ][ 'emotion' ].values(),
result[ 0 ][ 'emotion' ].keys()))[ 1 ])
print (query)
|
Output:
happy
Recommending Music using Spotify API
Using Spotify API to search music according to the emotion with the highest percentage. We use the Spotify API from RapidAPI. You can edit the following parameters in the below code:
- Type: The type of result we want to collect. You can input any of these values:
- multi: returns albums, artists, episodes, genres, playlists, podcasts, and tracks related to the search query
- albums: returns albums related to a search query
- artists: returns artists related to a search query
- episodes: returns episodes related to a search query
- genres: returns genres related to the search query
- playlists: returns playlists related to the search query
- podcasts: returns podcasts related to a search query
- tracks: returns tracks related to the search query
- Offset: Parameter to get the next set of results. The maximum value can be 100.
- Limit: Number of results to be fetched by the API
- Number of Top Results: Number of top picks according to user’s playing activity
You can add your API key by subscribing to Spotify API on the Rapid API website. Replace the <YOUR_API_KEY> with your generated key.
Python3
querystring = { "q" : f "{query}" , "type" : "multi" ,
"offset" : "0" , "limit" : "10" ,
"numberOfTopResults" : "5" }
headers = {
"X-RapidAPI-Key" : "<YOUR_API_KEY>" ,
"X-RapidAPI-Host" : "spotify81.p.rapidapi.com"
}
response = requests.get(url, headers = headers,
params = querystring)
for i in range ( 10 ):
print ( 'song name:' , response.json()[ 'tracks' ]
[i][ 'data' ][ 'name' ],
'\nalbum name:' ,
response.json()[ 'tracks' ][i]
[ 'data' ][ 'albumOfTrack' ][ 'name' ], '\n' )
|
Output:
song name: Happy - From "Despicable Me 2"
album name: G I R L
song name: Happy Together
album name: Happy Together
song name: HAPPY
album name: HOPE
song name: Happy?
album name: Lost and Found
song name: Happy Pills
album name: Happy Pills
song name: Happy
album name: Ashanti
song name: Happy Birthday to You
album name: Happy Birthday to You! Songs & Lieder zum Geburtstag,
Geburtstagslieder
song name: Happy Birthday Song
album name: CoComelon Kids Hits, Vol. 3
song name: Happy Birthday
album name: Hotter Than July
song name: The Happy Song
album name: The Happy Song
The response from API shows 10 songs that match the search query. Here is the complete implementation:
Python3
def img_to_song(image_location,
api_key = "fbfcb9f8c1msh77a0f765228b1cap14b26djsned951f12e1cd" ,
api_host = "spotify81.p.rapidapi.com" ,
offset = 0 ,
limit = 10 ,
numberOfTopResults = 5 ):
img = cv2.imread(image_location)
result = DeepFace.analyze(img,
actions = [ 'emotion' ])
query = str ( max ( zip (result[ 0 ][ 'emotion' ].values(),
result[ 0 ][ 'emotion' ].keys()))[ 1 ])
url = str (api_url)
querystring = { "q" : f "{query}" , "type" : "multi" ,
"offset" : str (offset), "limit" : str (limit),
"numberOfTopResults" : str (numberOfTopResults)}
headers = {
"X-RapidAPI-Key" : str (api_key),
"X-RapidAPI-Host" : str (api_host)
}
response = requests.get(url, headers = headers,
params = querystring)
output = list ()
for i in range (limit):
output.append(f
)
return output
loc = 'image.jpg'
k = img_to_song(loc)
print (k)
|
Output:
Action: emotion: 100%|██████████| 1/1 [00:00<00:00, 2.30it/s]
Action: emotion: 100%|██████████| 1/1 [00:00<00:00, 14.28it/s]
Action: emotion: 100%|██████████| 1/1 [00:00<00:00, 15.68it/s]
Action: emotion: 100%|██████████| 1/1 [00:00<00:00, 14.28it/s]
['song name: Happy - From "Despicable Me 2"
album name:G I R L\n', 'song name: Happy Together
album name:Happy Together\n', 'song name: HAPPY
album name:HOPE\n', 'song name: Happy?
album name:Lost and Found\n', 'song name: Happy Pills
album name:Happy Pills\n', 'song name: Happy
album name:Ashanti\n', 'song name: Happy Birthday to You
album name:Happy Birthday to You! Songs & Lieder zum Geburtstag, Geburtstagslieder
\n', 'song name: Happy Birthday Song
album name:CoComelon Kids Hits, Vol. 3\n', 'song name: Happy Birthday
album name:Hotter Than July\n', 'song name: The Happy Song
album name:The Happy Song\n']
Conclusion
In this article, we discussed how to recommend songs from Facial Expressions. There are various methods available to achieve higher accuracy, this is a basic implementation for you to get started.
Share your thoughts in the comments
Please Login to comment...