Data Analytics and its type
Last Updated :
11 Feb, 2024
Data analytics is an important field that involves the process of collecting, processing, and interpreting data to uncover insights and help in making decisions. Data analytics is the practice of examining raw data to identify trends, draw conclusions, and extract meaningful information. This involves various techniques and tools to process and transform data into valuable insights that can be used for decision-making.
In this article, we will learn about Data analytics,data which will help businesses and individuals that can help them to enhance and solve complex problems, Types of Data Analytics, Techniques, Tools, and the Importance of Data Analytics.
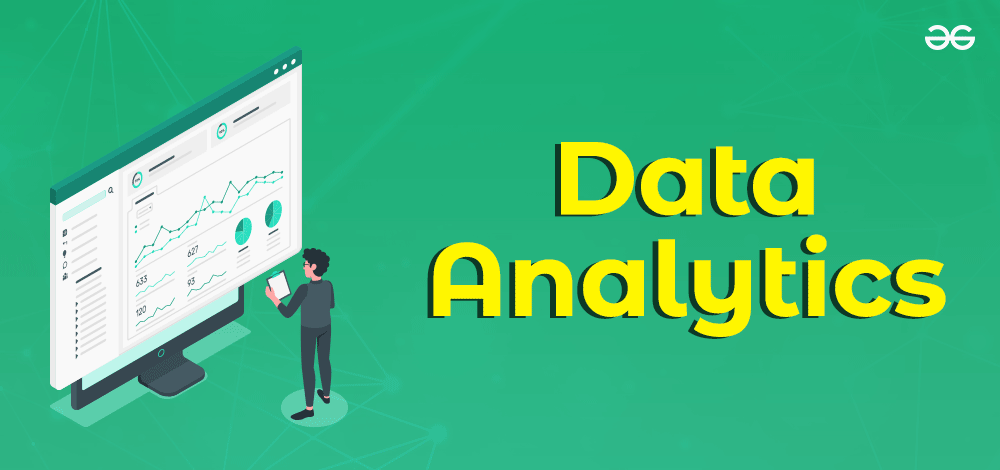
Data Analytics
What is Data Analytics?
In this new digital world, data is being generated in an enormous amount which opens new paradigms. As we have high computing power and a large amount of data we can use this data to help us make data-driven decision making. The main benefits of data-driven decisions are that they are made up by observing past trends which have resulted in beneficial results.
In short, we can say that data analytics is the process of manipulating data to extract useful trends and hidden patterns that can help us derive valuable insights to make business predictions.
Understanding Data Analytics
Data analytics encompasses a wide array of techniques for analyzing data to gain valuable insights that can enhance various aspects of operations. By scrutinizing information, businesses can uncover patterns and metrics that might otherwise go unnoticed, enabling them to optimize processes and improve overall efficiency.
For instance, in manufacturing, companies collect data on machine runtime, downtime, and work queues to analyze and improve workload planning, ensuring machines operate at optimal levels.
Beyond production optimization, data analytics is utilized in diverse sectors. Gaming firms utilize it to design reward systems that engage players effectively, while content providers leverage analytics to optimize content placement and presentation, ultimately driving user engagement.
Types of Data Analytics
There are four major types of data analytics:
- Predictive (forecasting)
- Descriptive (business intelligence and data mining)
- Prescriptive (optimization and simulation)
- Diagnostic analytics
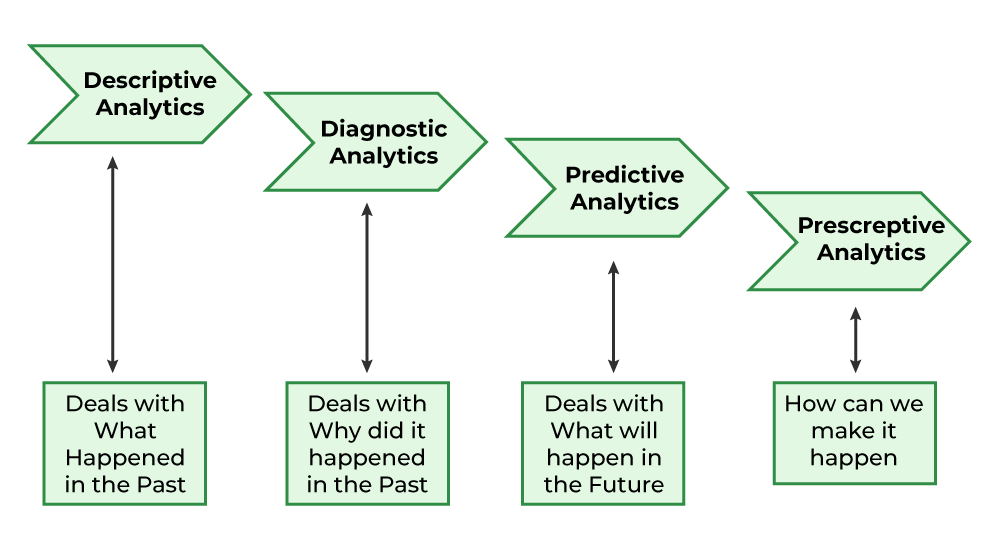
Data Analytics and its Types
Predictive Analytics
Predictive analytics turn the data into valuable, actionable information. predictive analytics uses data to determine the probable outcome of an event or a likelihood of a situation occurring. Predictive analytics holds a variety of statistical techniques from modeling, machine learning, data mining, and game theory that analyze current and historical facts to make predictions about a future event. Techniques that are used for predictive analytics are:Â
- Linear Regression
- Time Series Analysis and Forecasting
- Data Mining
Basic Cornerstones of Predictive Analytics
- Predictive modeling
- Decision Analysis and optimization
- Transaction profiling
Descriptive Analytics
Descriptive analytics looks at data and analyze past event for insight as to how to approach future events. It looks at past performance and understands the performance by mining historical data to understand the cause of success or failure in the past. Almost all management reporting such as sales, marketing, operations, and finance uses this type of analysis.Â
The descriptive model quantifies relationships in data in a way that is often used to classify customers or prospects into groups. Unlike a predictive model that focuses on predicting the behavior of a single customer, Descriptive analytics identifies many different relationships between customer and product.Â
Common examples of Descriptive analytics are company reports that provide historic reviews like:Â
- Data Queries
- Reports
- Descriptive Statistics
- Data dashboard
Prescriptive Analytics
Prescriptive Analytics automatically synthesize big data, mathematical science, business rule, and machine learning to make a prediction and then suggests a decision option to take advantage of the prediction.Â
Prescriptive analytics goes beyond predicting future outcomes by also suggesting action benefits from the predictions and showing the decision maker the implication of each decision option. Prescriptive Analytics not only anticipates what will happen and when to happen but also why it will happen. Further, Prescriptive Analytics can suggest decision options on how to take advantage of a future opportunity or mitigate a future risk and illustrate the implication of each decision option.Â
For example, Prescriptive Analytics can benefit healthcare strategic planning by using analytics to leverage operational and usage data combined with data of external factors such as economic data, population demography, etc.Â
Diagnostic Analytics
In this analysis, we generally use historical data over other data to answer any question or for the solution of any problem. We try to find any dependency and pattern in the historical data of the particular problem.Â
For example, companies go for this analysis because it gives a great insight into a problem, and they also keep detailed information about their disposal otherwise data collection may turn out individual for every problem and it will be very time-consuming. Common techniques used for Diagnostic Analytics are:Â
- Data discovery
- Data mining
- CorrelationsÂ
The Role of Data Analytics
Data analytics plays a pivotal role in enhancing operations, efficiency, and performance across various industries by uncovering valuable patterns and insights. Implementing data analytics techniques can provide companies with a competitive advantage. The process typically involves four fundamental steps:
- Data Mining: This step involves gathering data and information from diverse sources and transforming them into a standardized format for subsequent analysis. Data mining can be a time-intensive process compared to other steps but is crucial for obtaining a comprehensive dataset.
- Data Management: Once collected, data needs to be stored, managed, and made accessible. Creating a database is essential for managing the vast amounts of information collected during the mining process. SQL (Structured Query Language) remains a widely used tool for database management, facilitating efficient querying and analysis of relational databases.
- Statistical Analysis: In this step, the gathered data is subjected to statistical analysis to identify trends and patterns. Statistical modeling is used to interpret the data and make predictions about future trends. Open-source programming languages like Python, as well as specialized tools like R, are commonly used for statistical analysis and graphical modeling.
- Data Presentation: The insights derived from data analytics need to be effectively communicated to stakeholders. This final step involves formatting the results in a manner that is accessible and understandable to various stakeholders, including decision-makers, analysts, and shareholders. Clear and concise data presentation is essential for driving informed decision-making and driving business growth.
Steps in Data Analysis
- Define Data Requirements: This involves determining how the data will be grouped or categorized. Data can be segmented based on various factors such as age, demographic, income, or gender, and can consist of numerical values or categorical data.
- Data Collection: Data is gathered from different sources, including computers, online platforms, cameras, environmental sensors, or through human personnel.
- Data Organization: Once collected, the data needs to be organized in a structured format to facilitate analysis. This could involve using spreadsheets or specialized software designed for managing and analyzing statistical data.
- Data Cleaning: Before analysis, the data undergoes a cleaning process to ensure accuracy and reliability. This involves identifying and removing any duplicate or erroneous entries, as well as addressing any missing or incomplete data. Cleaning the data helps to mitigate potential biases and errors that could affect the analysis results.
Usage of Data Analytics
There are some key domains and strategic planning techniques in which Data Analytics has played a vital role:
- Improved Decision-Making – If we have supporting data in favour of a decision, then we can implement them with even more success probability. For example, if a certain decision or plan has to lead to better outcomes then there will be no doubt in implementing them again.
- Better Customer Service – Churn modeling is the best example of this in which we try to predict or identify what leads to customer churn and change those things accordingly so, that the attrition of the customers is as low as possible which is a most important factor in any organization.
- Efficient Operations – Data Analytics can help us understand what is the demand of the situation and what should be done to get better results then we will be able to streamline our processes which in turn will lead to efficient operations.
- Effective Marketing – Market segmentation techniques have been implemented to target this important factor only in which we are supposed to find the marketing techniques which will help us increase our sales and leads to effective marketing strategies.
Future Scope of Data AnalyticsÂ
- Retail: To study sales patterns, consumer behavior, and inventory management, data analytics can be applied in the retail sector. Data analytics can be used by retailers to make data-driven decisions regarding what products to stock, how to price them, and how to best organize their stores.
- Healthcare: Data analytics can be used to evaluate patient data, spot trends in patient health, and create individualized treatment regimens. Data analytics can be used by healthcare companies to enhance patient outcomes and lower healthcare expenditures.
- Finance: In the field of finance, data analytics can be used to evaluate investment data, spot trends in the financial markets, and make wise investment decisions. Data analytics can be used by financial institutions to lower risk and boost the performance of investment portfolios.
- Marketing: By analyzing customer data, spotting trends in consumer behavior, and creating customized marketing strategies, data analytics can be used in marketing. Data analytics can be used by marketers to boost the efficiency of their campaigns and their overall impact.Â
- Manufacturing: Data analytics can be used to examine production data, spot trends in production methods, and boost production efficiency in the manufacturing sector. Data analytics can be used by manufacturers to cut costs and enhance product quality.
- Transportation: To evaluate logistics data, spot trends in transportation routes, and improve transportation routes, the transportation sector can employ data analytics. Data analytics can help transportation businesses cut expenses and speed up delivery times.
Conclusion
Data Analytics act as tool that is used for both organizations and individuals that seems to use the power of data. As we progress in this data-driven age, data analytics will continue to play a pivotal role in shaping industries and influencing future.
Share your thoughts in the comments
Please Login to comment...