In this transformative realm of healthcare, Are you ready to journey through the incredible world of machine learning and its groundbreaking impact on healthcare? In this era where technology and medicine intertwine more closely than ever, machine learning stands out as a beacon of innovation, pushing the boundaries of what’s possible in patient care, diagnosis, and treatment.
This article can be your golden ticket to understanding the Top Machine Learning projects for Healthcare making waves in the healthcare sector in 2024.
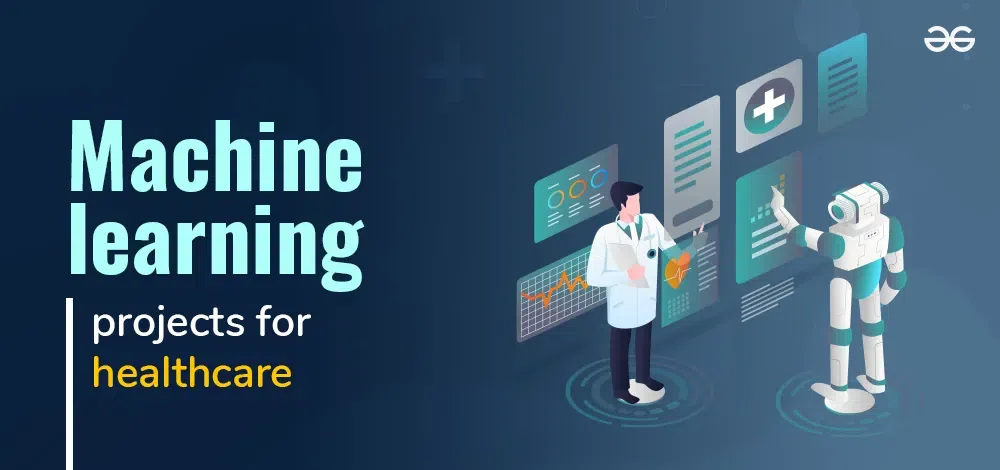
Machine Learning Projects for Healthcare
Introduction to Machine Learning in Healthcare
When we talk about humans, their health comes along with them. The global population is aging, and day-to-day lifestyle changes, such as unhealthy diets and lack of physical activity, have contributed to the prevalence of diseases like obesity and diabetes and many chronic diseases and the need for long-term care. The demand for high-quality healthcare services is on the rise, fueled by increasing incomes and growing health awareness. In this context, technologies providing efficient, helpful, and rapid health analysis are highly sought after. Artificial Intelligence (AI) and Machine Learning (ML) have emerged as dominant forces across various industries, continually making headlines with their innovative applications. While Machine Learning for healthcare has gained prominence in finance and banking, its influence extends to diverse sectors, including healthcare. With substantial data generated for each patient, machine learning algorithms hold immense potential in the healthcare landscape. Recognizing the transformative capabilities of Machine Learning projects for healthcare.
Top 10 Machine Learning Projects for Healthcare
Healthcare providers are harnessing machine learning to enhance patient outcomes, streamline disease diagnosis, analyze Electronic Health Records (EHRs), reduce medical costs, and optimize operational efficiency. According to a Grand View Research report, the global machine learning market in healthcare is projected to reach $31.65 billion by 2025, showcasing a notable CAGR of 44.5% from 2019 to 2025. The increasing adoption of machine learning applications in healthcare is reshaping both patient care and administrative processes within the industry. Here is a list of the Top 10 Machine Learning Healthcare Projects that underscore ML’s potential to outperform human capabilities, particularly in disease diagnosis, where algorithms exhibit superior proficiency in detecting diseases.
1. Medical Diagnostics
Medical diagnostics have become increasingly important in modern healthcare, as they provide invaluable insights that can help medical doctors detect and diagnose diseases and Machine learning (ML) is playing a transformative role in advancing it. Through the utilization of ML algorithms, medical professionals can leverage vast datasets, including medical images, patient records, and clinical notes, to enhance diagnostic accuracy and efficiency. ML excels in image recognition tasks, making it particularly valuable in medical imaging diagnostics. Algorithms can analyze complex medical images such as X-rays, MRIs, and CT scans, aiding in the identification of anomalies and assisting healthcare practitioners in making more precise diagnoses. Image recognition algorithms have shown great success in recognizing patterns to spot diseases, for example, to help physicians identify minor changes in tumors to detect malignancy. In the realm of medical diagnostics, ML holds the potential to streamline workflows, reduce diagnostic errors, and optimize patient outcomes. By embracing these technological advancements, healthcare providers can offer more accurate and timely diagnoses, ultimately improving the overall quality of healthcare.
Here is a project for your reference: Disease Prediction Using Machine Learning
2. Parkinson’s Disease Detection
Parkinson’s disease (PD) is a prevalent neurological disorder impacting muscle movement, affecting mobility, speech, and posture with symptoms such as tremors, muscle rigidity, and bradykinesia. This condition results from neuronal death, causing a decline in dopamine levels in the brain. The reduction in dopamine adversely affects synaptic communication, leading to impaired motor functions. Early detection of PD is crucial for effective treatment, enabling patients to maintain a normal life. With the global increase in the aging population, there is a growing emphasis on the importance of early, remote, and accurate PD detection. Machine learning techniques, including voice analysis, handwriting analysis, and movement sensors, present non-invasive and potentially cost-effective alternatives to traditional diagnostic methods like PET scans. Personalized treatment plans can be developed through machine learning models tailored to individual patient data, enhancing the potential for more targeted and effective interventions.
Here is a project for your reference: Parkinson Disease Prediction
3. Breast Cancer Diagnosis
Machine learning revolutionizes breast cancer diagnosis with advanced tools for early detection and precise predictions. These models analyze diverse data sources, including mammography, ultrasound, and MRI, to identify patterns and anomalies, enhancing the accuracy of diagnoses. Computer-aided diagnosis (CAD) systems, powered by machine learning, assist radiologists by highlighting potential areas of concern in medical imaging, optimizing diagnostic capabilities. Additionally, machine learning utilizes large datasets encompassing patient demographics, medical history, and genetic factors to create predictive models for assessing breast cancer risk. This proactive approach enables personalized screening strategies and interventions for individuals at high risk. In essence, machine learning applications in breast cancer diagnosis empower healthcare professionals with innovative solutions for improved accuracy, rapid detection, and more effective treatment strategies, ultimately leading to better patient outcomes.
Here is the project for your reference:
4. Cancer Cell classification
Cancer cell classification using machine learning is a groundbreaking approach in oncology that involves the application of advanced algorithms to categorize cancer cells into distinct subtypes. This process is pivotal for gaining a comprehensive understanding of tumor diversity, aiding in the development of tailored treatment strategies. Machine learning models, particularly convolutional neural networks (CNNs), are instrumental in image-based classification. In this project uses approach that leverages the Breast Cancer Wisconsin (Diagnostic) dataset, which includes data on tumor attributes such as radius, texture, and perimeter. After installing necessary Python modules, loading and organizing the dataset, and splitting it into training and test sets. It then details the process of building a machine learning model using the Naive Bayes algorithm, a choice driven by its effectiveness in binary classification tasks. The model’s accuracy, evaluated at approximately 94.15%, demonstrates the potential of machine learning in enhancing diagnostic processes. This practical example not only highlights the steps involved in applying machine learning for medical diagnostics but also underscores the broader implications of technology in transforming healthcare outcomes through data-driven insights.
Here is a project for your reference: Cancer cell classification using Scikit-learn
5. Heart Disease Prediction
Leveraging machine learning for heart disease detection represents a groundbreaking approach in modern healthcare. Advanced algorithms, such as decision trees, support vector machines, and neural networks, are employed to scrutinize extensive datasets encompassing patient demographics, medical histories, and diagnostic test results. This data-driven methodology enables the identification of intricate patterns and correlations, facilitating early diagnosis and personalized treatment plans. This project outlines a methodical approach to predict heart disease using ANN, a type of deep learning model that mimics neural networks of the brain. This process begins with importing essential libraries in Python, such as TensorFlow and Keras, and progresses through data preprocessing, model building, and evaluation stages. The dataset used includes 13 attributes like age, sex, and cholesterol levels, serving as independent variables to predict the presence of heart disease. The ANN model is meticulously constructed with an input layer, hidden layers with activation functions, and an output layer, optimized using the ‘adam’ optimizer and ‘binary_crossentropy’ loss function. The model’s performance, achieving an accuracy of approximately 85%, underscores the potential of ANN in enhancing diagnostic accuracy and facilitating early intervention strategies. This project highlights accuracy and facilitating the border implications of machine learning in transforming healthcare.
Here is a project for your reference: Heart Disease Prediction using ANN
6. Lung Cancer Detection
Machine learning’s role in lung cancer detection is reshaping medical diagnostics. Advanced algorithms, including support vector machines and convolutional neural networks, analyze diverse datasets like medical images and genetic information. These models excel in early and accurate identification of lung cancer, particularly in analyzing chest X-rays and CT scans for subtle abnormalities. Early detection through machine learning significantly improves treatment outcomes. Machine learning into lung cancer detection not only enhances diagnostic accuracy but also enables personalized treatment plans. Tailoring interventions based on individual genetic makeup and risk factors maximizes efficacy and minimizes side effects. In summary, machine learning applications in lung cancer detection promise to revolutionize pulmonary diagnostics, offering a proactive and personalized approach to combat this prevalent health condition.
Here is the project for your reference: Lung Cancer Detection
7. Pneumonia Detection
The landscape of pneumonia detection has witnessed a revolutionary transformation through the integration of cutting-edge technologies such as deep learning and machine learning. These sophisticated methodologies, notably leveraging convolutional neural networks (CNNs) and support vector machines (SVMs), have showcased exceptional precision in scrutinizing medical imagery like chest X-rays and CT scans. Machine learning models undergo rigorous training on expansive datasets, acquiring an adept understanding of nuanced patterns and variations associated with pneumonia. This enables these algorithms to swiftly and accurately identify abnormal conditions within the lungs, providing clinicians with invaluable support for prompt diagnosis and intervention. The incorporation of deep learning, particularly through CNNs, introduces a layer of automation in feature extraction from medical images.By taking into account patient histories, demographic information, and other relevant factors, these models assist in forecasting potential pneumonia risks. This proactive approach empowers healthcare providers to implement preventive measures and tailor treatment plans based on the unique profiles of individual patients. This amalgamation promises not only enhanced accuracy and efficiency in identifying pneumonia but also a more personalized and patient-centric approach to managing and mitigating this respiratory condition, ultimately resulting in improved overall patient outcomes.
Here is a project for your reference: Pneumonia Detection
8. Skin Cancer Detection
The landscape of skin cancer detection has undergone a transformative shift, driven by cutting-edge technologies like machine learning and deep learning. These advanced methodologies, prominently featuring convolutional neural networks (CNNs) and support vector machines (SVMs), showcase remarkable prowess in scrutinizing dermatological imagery for the early identification of skin cancer. Machine learning models, honed on diverse datasets encompassing a broad spectrum of skin conditions, exhibit a keen ability to discern subtle patterns indicative of malignant lesions. Analyzing features such as asymmetry, border irregularities, color variations, and diameter within images, these algorithms deliver rapid and accurate assessments, proving invaluable to dermatologists for early diagnosis. By considering patient history, demographic factors, and other pertinent information, these models assist in forecasting potential risks, facilitating preventive measures and personalized treatment plans tailored to individual patient profiles. The powerful combination of machine learning and deep learning ensures not only enhanced accuracy in identifying skin cancer but also enables a proactive and personalized approach to managing and mitigating this serious health concern. The result is improved patient outcomes, with the technology proving to be a game-changer in the ongoing battle against skin cancer.
Here is the project for your reference: Skin Cancer Detection using TensorFlow
9. Detecting Covid-19
Detecting COVID-19 through machine learning and deep learning has emerged as a pivotal tool in the global battle against the pandemic. Machine learning models, trained on diverse datasets of medical imaging such as X-rays and CT scans, exhibit remarkable proficiency in identifying patterns and anomalies associated with COVID-19. Analyzing radiological images, these models discern subtle features that serve as indicators of the viral infection. The swift and accurate analysis by these algorithms facilitates rapid diagnoses, enabling prompt isolation and treatment of affected individuals. Utilizing the Xception model, a deep learning framework known for its efficiency in handling image data, the article outlines a method for analyzing chest X-ray images to differentiate between COVID-19 positive cases, viral pneumonia, and normal instances. The process involves preprocessing the images to fit the model’s input requirements, employing data augmentation techniques to enhance the dataset, and finally, training the Xception model to classify the images into the respective categories. Achieving an impressive accuracy rate on both training and validation sets, this approach exemplifies the potential of deep learning models like Xception in providing rapid and accurate diagnostic tools.
Here is a project for your reference: Detecting Covid-19 with Chest X-ray
10. Health records improvement
“Text Detection and Extraction using OpenCV and OCR” delves into the technical prowess of OpenCV and OCR for text detection and extraction, a methodology that can be adeptly applied to the healthcare sector for managing patient records. By employing OpenCV for image processing and Python-tesseract as a wrapper for Google’s Tesseract-OCR Engine, this approach automates the extraction of textual data from images, which is pivotal for digitizing and structuring health records. The process involves image preprocessing techniques such as color space conversion and thresholding, followed by contour detection to identify text blocks within images. This automated system not only streamlines the extraction of critical health information from various formats but also significantly reduces manual entry errors, thereby lightening the workload of healthcare professionals. The integration of machine learning, particularly through NLP algorithms, further refines the extraction and structuring of data from clinical notes, ensuring organized and standardized records. This synergy of machine learning technologies holds the promise of revolutionizing healthcare record management, offering a more accurate, real-time, and error-minimized approach to diagnosing and treating diseases.
Here is the project for your reference:
Conclusion
Top machine learning projects for Healthcare analytics, showcasing its prowess across various critical applications as detailed in this article. From the management of electronic health records to the diagnosis and prognosis of diseases, machine learning algorithms emerge as indispensable tools for healthcare providers. Using ML models the healthcare industry has emerged and a lot is yet to come. NLP and Computer Vision technology are widely used and is proofing their dominance in the healthcare industry. Harnessing the power of machine learning empowers healthcare providers to elevate patient outcomes, slash costs, and optimize operational efficiency.. Here we explored 10 machine learning projects for healthcare domain but I assure you there are many more projects and use cases in healthcare domain.
Machine Learning Projects for Healthcare – FAQ’s
How machine learning can help in predicting pandemics ?
By harnessing data and real time updates, and web-based sources, the ML excel in foreseeing and predicting epidemic outbreaks. Although these examples showcase the current landscape of ML applications, the future holds the promise of even more sophisticated and revolutionary implementations in the realm of healthcare. As ML undergoes continuous evolution, we can expect paradigm-shifting developments that will transform lives, proactively prevent diseases.
Which machine learning models/Algorithms are used in healthcare?
Many models and algorithms are used in healthcare depending on the use case. From simpler Model like Logistic Regression to very complex model like RNN and LSTM can be used.
What is the use of NLP in Healthcare?
Since many of the healthcare records are unstructured textual data, so NLP can extract relevant information from clinical notes, transcripts, and other unstructured text, helping in the creation and updating of patient records. This improves the accuracy and efficiency of maintaining electronic health records.
Share your thoughts in the comments
Please Login to comment...