Machine Learning for Healthcare
Last Updated :
20 Mar, 2024
Machine Learning is a branch of Artificial Intelligence that helps computers learn and understand the data and recognize trends to make future predictions. ML uses algorithms that allow computers to identify patterns, make predictions, and derive insights from data, much like humans learn from experience.
Machine Learning plays an important role in the Healthcare Sector helping researchers and doctors identify important trends in the patient’s history and make valuable decisions before the disease spreads or reaches the last stage, it also helps in identifying the disease and its causes accurately. In this article, we will understand how machine learning will play an important role in healthcare in 2024 in various ways.
Machine Learning in Healthcare
Introduction to Machine Learning for Healthcare
In recent years, Machine Learning has revolutionized the healthcare sector and helped in longstanding problems. Digital Growth in India has exponentially supported and encouraged the integration of AI, Machine Learning, and Data science. From electronic health records (EHRs), medical imaging, and genomic sequencing, to wearable sensor data ML has come a long way in helping scientists, researchers, and doctors in the early analysis of disease and suggesting preventive measures. From personalized medicine and early disease detection to drug discovery and remote patient monitoring, ML, data science, and AI are reshaping every aspect of the healthcare ecosystem, ushering in a new era of precision medicine, predictive analytics, and patient-centered care.
Need of ML in Healthcare in 2024
ML has been an important part of the Healthcare domain and now it works diversly in 2024. Some of the uses of ML in healthcare are mentioned below:
- Data Complexity: The medical sector produces a wide and complex range of data that is not easy to understand and study. ML algorithms make it easy to analyze and predict complex datasets.
- Early Disease Detection: Identifying from the patterns and medical history of the patient ML algorithms also helps in early detection of the disease.
- Treatment Optimization: ML algorithms also help in recommending accurate treatment based on the history and current condition of the patient. It also helps doctors avoid any side effects by identifying the possibilities before treatment.
- Predictive Analytics: Based on the diverse data provided and cases that occurred in the past ML models can also predict the disease and help in early treatment to spread it.
Top Uses of Machine Learning, Data Science, and AI in Healthcare
1. Enhanced Medical Imaging
Machine Learning Algorithms are widely used in the real world for image segmentation, classification, and anomaly detection. 2024 is also the year for advancement in convolutional neural networks (CNNs) which helps in diagnostic processes since it provides high accuracy and efficiency. ML functions help in the early detection of cancer, cardiovascular diseases, and neurological conditions. The MRI and CT scan of a patient provides knowledge about the condition of the patient and ML-powered technologies also help in dealing with these serious diseases at early stages. For example, This technology also helped Massachusetts General Hospital analyze MRI images and accurately predict the development of Alzheimer’s disease up to six years in advance helping in early medical treatment. The researchers used deep learning to fit the model and study the MRI of the brain. These early detections not only help researchers and doctors prevent the disease from spreading but it also helps in taking accurate steps to cure it.
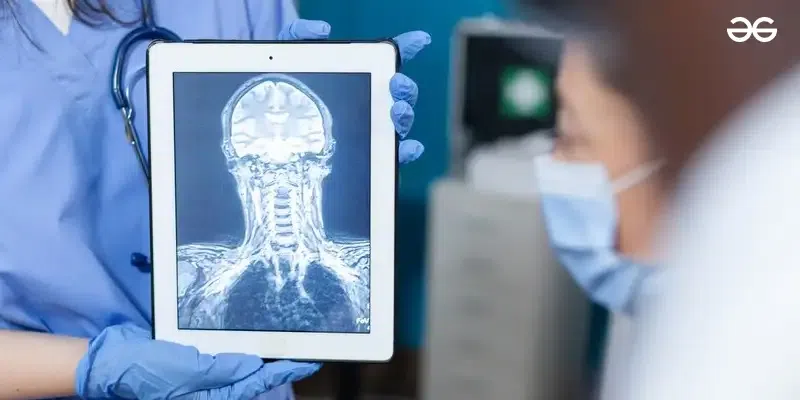
Enhanced Medical Imaging
2. Predictive Analytics and Disease Prevention
Machine Learning plays an important role in studying the data available for a certain disease and the patients associated with it. It tries to recognize the trends in patients’ medical history, lifestyle changes, and choices, genetic information, etc. The models we fit in machine learning identify trends in these datasets and predict the possible outcomes of diseases. Recent researchers have identified that ML algorithms can predict diabetic retinopathy progression based on retinal images. This technology has been in use for a long time the Geisinger Health System in Pennsylvania implemented a predictive analytics program to identify patients at high risk of sepsis and in 2024 this technology can help thousands of people identify and forecast diseases at early stages and bring accurate preventive measures to save lives.
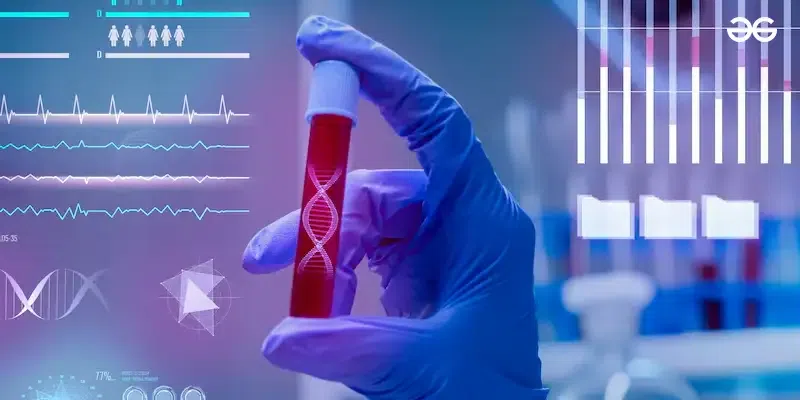
Predictive Analytics and Disease Prevention
3. Personalized Medicine
ML-powered decision systems and personalized prediction help management for individual patient based on their medical history, genetic makeup, biomarkers, and clinical profiles. Algorithms of machine learning study the complex data and suggest the best set of medicines for the patient based on their hath conditions. The proper study of all the aspects of clinical treatment also reduces the possibility of adverse effects. For example, IBM Watson for Oncology utilizes natural language processing(NLP) and machine learning to provide personalized treatment recommendations for cancer patients. ML is widely used to improve the treatment of cancer patients as pharmacogenomic models have helped in the targeted treatment improving the survival rate and quality of life.
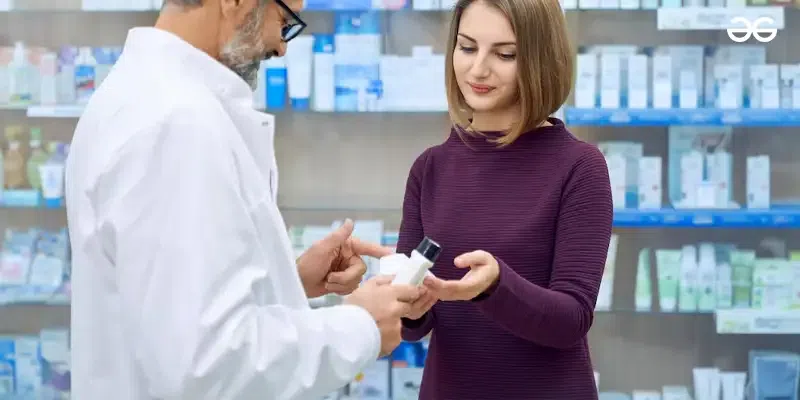
Personalized Medicine
4. Remote Patient Monitoring
After the COVID pandemic, remote patient monitoring became famous and Machine Learning algorithms are used in it to detect and analyze patient’s conditions timely and regularly. Many companies have now started using ML models to check on patients’ health and give signs of early deterioration and timely interventions to reduce hospital readmissions. These models help us doctors in monitoring the patients by taking proper measures of maintaining distance. As well as there are technologies that are growing for telemedicine that provide personalized health recommendations and facilitate remote consultations with healthcare providers.
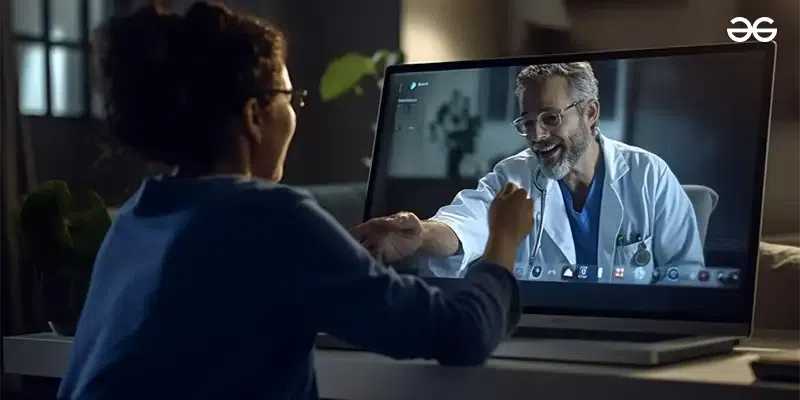
Remote Patient Monitoring
5. Drug Testing
ML models can go through a wide range of data and complex data sets including genomics, proteomics, and transcriptomics identifying the drug associated with a particular disease and its symptoms. ML models can also predict the likelihood of adverse drug reactions and toxicity based on chemical structure. It also helps in building a chemical library that can help identify details about the promising drug as well as it helps in virtual screening of the chemical library. ML models can also identify toxic drugs and suggest a selection of safer drugs for a particular patient based on the patient’s history and medical condition.
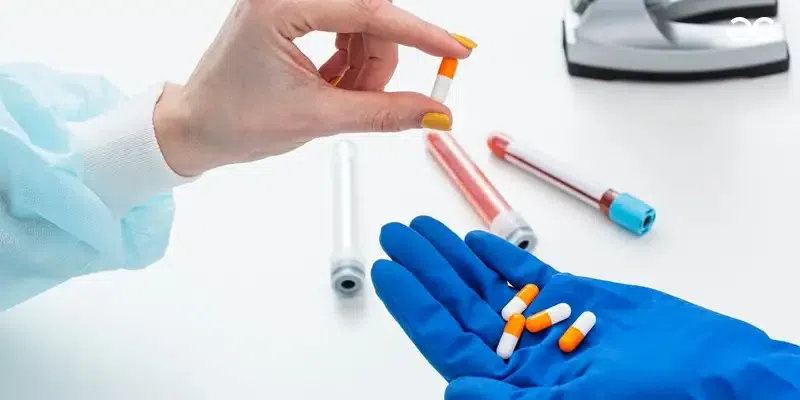
Drug Testing
6. Mental Health
By integrating patient-specific information with evidence-based guidelines and clinical expertise, ML-driven decision support systems can assist clinicians in selecting optimal interventions, medication dosages, and therapy modalities for each patient. ML models help in identifying these patterns at an early stage. Multiple ML-powered sources provide specific therapy to the patients making it cost effective and increasing the availability of treatment to everyone. It can also help understand the patterns of public health based on the factors present bring preventive measures and promote mental health awareness and education for the same. It also helps in reducing the stigma that we still have regarding mental health in 2024.
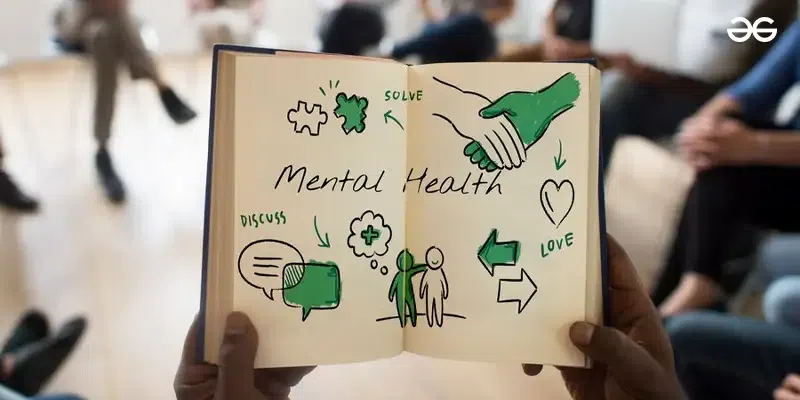
Mental Health
7. Ethical and Regulatory Considerations
While Machine Learning is growing rapidly in the medical sector it is also important to address that medical history and personal information of the patient is to be kept private and should not be misused. The collaborative work between data scientists, clinicians, ethicists, and policymakers should follow the guidelines and protect the privacy of an individual.
Machine Learning is a powerful tool in 2024 revolutionizing the medical domain and bringing effective research saving thousands of lives and it also helps in preventive measures. These ML-powered facilities and technologies also make treatment accessible to the large and needy.
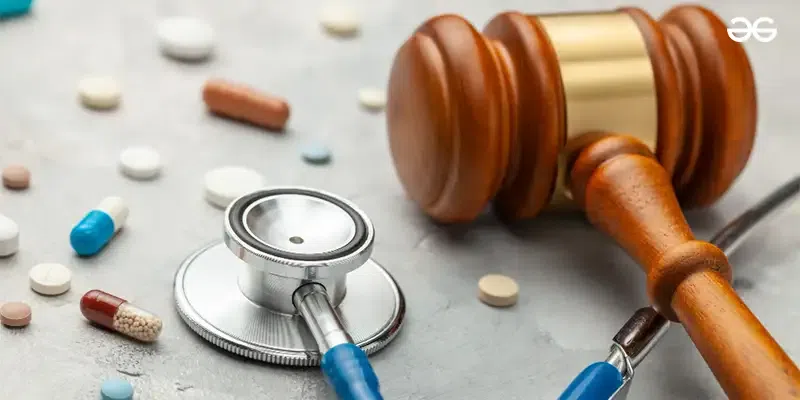
Ethical and Regulatory Considerations
Application of Machine Learning in Healthcare:
Machine Learning and AI are widely used in the healthcare sector, some of them are discussed below:
- Medical Imaging Analysis: Algorithms in Machine Learning help in analyzing the MRI or CT scan to find diseases like cancer, cardiovascular disorders, and neurological conditions.
- Predictive Analytics for Disease Prevention: Based on the past conditions of the patient and the symptoms available, ML models can predict the disease at an early stage and stop its initial stages.
- Personalized Medicine: ML models use genetic data, biomarkers, and the history of the patient to give personalized medicine and treatment.
- Drug Discovery and Development: AI models help in the virtual screening of chemical compounds, predicting drug-target interactions, identifying potential drug causes, and also taking care of the side effects.
- Remote Patient Monitoring: Machine Learning models along with IoT and devices can measure and keep records of the patient in real-time and detect any changes.
- Healthcare Operations Optimization: It is also used in the optimization of workforce allocation, management of medicines and patients, scheduling, and resource management.
- Fraud Detection and Healthcare Fraud Prevention: ML is used for medical purposes as well as management purposes. It can be used to detect fraud and billing systems, health records, etc.
- Healthcare Chatbots and Virtual Assistants: AI-powered chatbots can be helpful to the masses as it is accessible and reliable but not for serious issues. People can gain knowledge from these bots.
- Genomic Medicine and Precision Oncology: ML models identify possible threats of cancer based on genomic data, and biomarkers and help in oncology for personalized and specialized study.
- Healthcare Supply Chain Management and Inventory Optimization: Other than the medical support ML gives it also helps in maintaining the healthcare supply chain.
No-Code Machine Learning
Limited coding and Machine Learning knowledge shouldn’t be an obstacle to using these high technologies in our healthcare sector. In the healthcare sector, the adoption of no-code ML platforms is driving significant growth and innovation by empowering healthcare professionals, researchers, and organizations to leverage data-driven insights and AI-driven solutions to improve patient care, optimize clinical workflows, and drive operational efficiency. Here’s how no-code ML is affecting the growth of healthcare:
- Accessibility and Democratization: No-code Machine Learning makes it accessible to the large and helps people get the benefits of it without having extensive knowledge of technology
- Rapid Prototyping and Iteration: Prototyping helps the researchers use these prototypes for different algorithms, datasets and parameters. This reduces time and increases efficiency.
- Decision Support and Clinical Intelligence: Prediction and preventive measures offered by Machine Learning and AI based on history of patient. It analyses all the aspects related to the patient’s history before giving solutions.
Conclusion
In this article, we discussed the potential of Machine Learning, AI and Data science in the Healthcare and how people who do not know advanced coding can benefit from it. ML is rapidly increasing and helping researchers and doctors in making health services more affective and advanced recognizing potential threat of disease and its cure at early stages.
Machine Learning for Healthcare in 2024 – FAQ’s
What is machine learning, and how is it used in healthcare?
Machine Learning is a subset of Artificial Intelligence that enables computer learn from the provided datasets and give predictions or future data on the basis of old dataset. It can be used in Healthcare for prediction of diseases, preventive measures and cure.
What are some examples of machine learning applications in medical imaging?
Machine learning algorithms analyze medical images such as X-rays, MRI scans, and CT scans to assist in diagnosing diseases like cancer, cardiovascular disorders, and neurological conditions with greater accuracy and efficiency.
How does machine learning contribute to personalized medicine?
Machine Learning can analyze personal data, history of diseases, biomarkers, genetic information to recommend personalized medicines.
What are some challenges associated with implementing machine learning in mental health care?
Protecting the data addressing algorithm bias and fairness, integrating machine learning into existing clinical workflows, and navigating ethical considerations related to the use of AI in mental health diagnosis and treatment is some of the challenges associated with machine learning in mental healthcare.
What is no-code machine learning, and how does it differ from traditional machine learning?
No-code machine learning and easy to access technique for using Machine Learning without writing code. Unlike traditional ML, which requires programming expertise, no-code ML platforms provide intuitive graphical interfaces and drag-and-drop tools, enabling users with limited technical skills to create and customize ML models.
Share your thoughts in the comments
Please Login to comment...