Have you ever used a ride-sharing app to find the nearest drivers, pinpointed a meeting location on a map, or checked a weather forecast showing precipitation patterns? If so, you have already interacted with geospatial analysis! This widespread, versatile field integrates geography, statistics, and data science to extract powerful insights from location-based information.
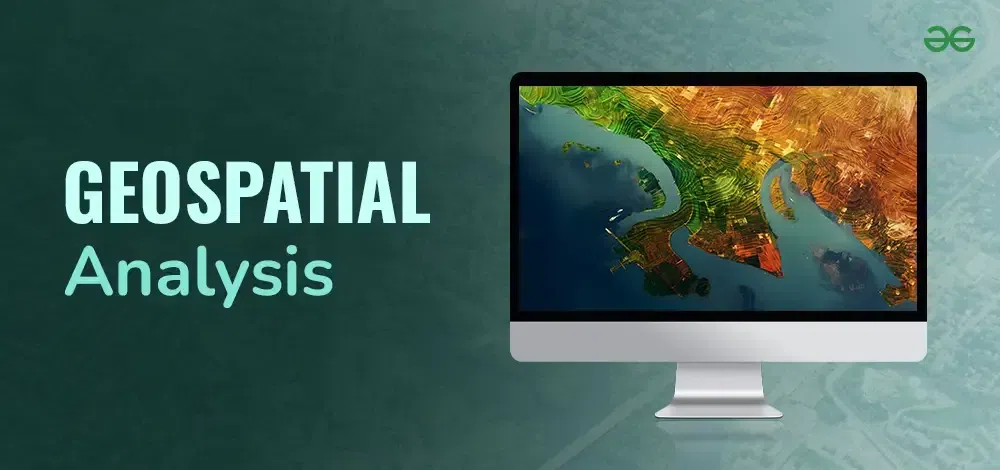
In this tutorial we’ll delve into depth of Geospatial Analysis.
What is Geospatial Analysis?
Geospatial analysis refers to the quantitative techniques applied to analyze and interpret geographical data—that is, data that contains location coordinates or spatial references. By combining cartography, spatial modeling, computational methods and GIS (geographic information systems), geospatial analysis reveals trends, relationships and patterns that inform business strategy, policy decisions, research discoveries and much more.
Key Components of Geospatial Analysis
Several integral components work together to enable the magic of geospatial analysis:
Geographic Information Systems (GIS)
GIS provides the fundamental framework to gather, organize, analyze and visualize geospatial data. This is specialized software that integrates many different layers of location-based information onto digital maps. For example, a city’s GIS may combine geospatial data on building footprints, road networks, subway routes, land-use zoning, utility infrastructure, climate patterns etc. GIS makes it possible to overlay these diverse datasets for a comprehensive view.
Advanced GIS capabilities allow users to generate 3D scenes, build data models, automate workflows, publish interactive web maps and more. Leading GIS software tools include ArcGIS, QGIS, Mapbox, CARTO and Google Earth Engine.
Spatial Data
Geospatial analysis involves examining and interpreting data with a geographic component, providing insights into spatial patterns, relationships, and trends. Various types of data contribute to geospatial analysis, enhancing our understanding of the Earth’s surface and its interactions. Here are some key types of data used in geospatial analysis:
- Vector Data (points, lines, polygons) representing discrete features like city boundaries, roads, landmarks etc.
- Raster Data (gridded cells, e.g., satellite imagery), aerial photos and digital elevation models.
- GPS Coordinates for precise location information
- Demographic Information
- Climate and Weather Data
- Crowdsourced Mapping Information
- Geotagged Social Media Posts.
- Traffic Data
- Transportation Patterns
- Temporal data – Time-series measurements from sensors and IoT devices, showing how phenomena vary over time.
Once spatial data has been collected and organized, various computational techniques can be applied to analyze patterns, relationships and trends. Geospatial analysis utilizes quantitative methods adapted from fields like statistics, data mining, simulation modeling and image processing.
Key techniques include:
- Spatial interpolation – Estimating unknown values at unmeasured locations based on surrounding known values. Useful for predicting continuous phenomena like elevation.
- Heat mapping – Using color gradation to represent the density and intensity of events or attributes clustered in an area. Often used to map crime rates, pollution, disease cases etc.
- Routing and logistics optimization – Finding the most efficient routes and distribution patterns based on parameters like transit time, capacity, or cost.
- Point pattern analysis – Examining the arrangement of point events like trees, retail stores, or bird sightings to identify spatial clusters, randomness or uniformity.
- Spatial regression – Statistically modeling relationships between variables and outcomes, while accounting for geographic proximity and dependencies.
Why is Geospatial Analysis Important?
Location-based context plays an increasingly ubiquitous role in drawing insights from data across industries and applications:
- Understand consumer behavior based on geography to optimize sales and marketing.
- Plan transportation infrastructure considering existing routes, landmarks, communities.
- Respond quickly to natural disasters by mapping affected populations and infrastructure.
- Track disease outbreaks and access to healthcare through spatial epidemiology.
- Assess environmental impacts by analyzing geographical patterns and changes.
- Improve agricultural productivity by mapping soil conditions, drainage, and other location-specific variables.
- Strengthen national security by monitoring borders, critical infrastructure etc.
- Design emergency responses by mapping real-time disasters and vulnerable areas.
In essence, adding geographical context through geospatial analysis creates visibility that drives better decision-making. It uncovers trends not apparent in data tables or dashboards. Geospatial analytics and mapping form the backbone of location intelligence that has become indispensable across sectors.
Key Applications of Geospatial Analysis
Let’s explore some major domains that routinely apply geospatial analysis to tap into its benefits:
Urban and Regional Planning
Urban planners face the complex task of designing infrastructure and services to sustainably support growing populations. Geospatial analysis helps tremendously by mapping current landscapes and modeling future scenarios. For instance, planners can overlay spatial data on:
- Population density and growth projections
- Zoning regulations
- Utility networks like water, sewage, electricity
- Transportation systems
- Vegetation, hydrology, and environmental aspects
- Land usage patterns and accessibility
- Socioeconomic demographics
Powerful geospatial computational methods help optimize land use allocation, simulate development impacts, forecast urban growth, evaluate accessibility to amenities, and ultimately improve quality of life through evidence-based planning.
Disaster Management
Geospatial technology plays a huge role in disaster monitoring, preparedness, response and recovery. For example:
- Track wildfires, floods and storms by layering real-time sensor data onto maps and 3D displays.
- Assess damage to critical infrastructure like roads, hospitals, power lines based on aerial imagery.
- Identify communities and facilities most vulnerable to disaster impacts through spatial analysis.
- Coordinate evacuations by mapping evacuation zones, routes and shelters.
- Develop early warning systems by modeling the spread of disasters.
- Guide emergency crews to affected sites using real-time routing and navigation.
- Manage logistics like dispatching supplies, mobilizing services and allocating aid efforts.
Business Intelligence
The business potential unlocked by location-based insights is invaluable across sectors like retail, real estate, transportation, tourism and more. For example:
- Select optimal locations for new stores or warehouses based on demographics, competitors, accessibility etc.
- Design sales territories, optimize delivery routes and fleet logistics using network analysis.
- Analyze customer behavior based on geography to tailor products, promotions and experiences.
- Enrich market segmentation by layering spatial variables like income, population, spending habits onto customer data.
- Monitor supply chain disruptions like port congestion by integrating logistics data with maps.
Environmental Management
Geospatial analytics help monitor environmental systems to detect changes and guide conservation efforts. Applications include:
- Create detailed land-use/land cover maps using satellite imagery and vector layers showing forests, water bodies, soil types etc.
- Model geographic distribution and migration patterns of flora and fauna.
- Identify biodiversity hotspots and establish protected wildlife corridors.
- Detect illegal activities like mining, logging, poaching through earth observation.
- Track gradual impacts like deforestation, desertification, melting glaciers by comparing geospatial data over time.
- Map pollution levels, greenhouse gas emissions, waste sites to assess and mitigate environmental risks.
- Analyze climate variability by integrating weather data with location-based measurements.
Public Health
The geographic dimensions of health issues provide key insights for policymakers and practitioners seeking to improve population wellbeing. Geospatial analysis helps in many ways:
- Pinpoint disease clusters and outbreak hotspots using location-tagged case data and statistical techniques.
- Identify access barriers and coverage gaps in healthcare facilities based on proximity analysis.
- Correlate health metrics like infection rates, morbidity, life expectancy with socioeconomic, environmental and demographic variables.
- Determine high-risk areas and efficient locations for public health interventions.
- Track population movements after disasters and epidemics to coordinate relief.
- Model spread of diseases to target containment measures to at-risk populations.
Archaeology and Social Sciences
Analyzing spatial patterns is invaluable for uncovering insights about past and present societies. Applications include:
- Map locations of archaeological artifacts and features to study ancient settlements and movements.
- Identify buried historical structures and artifacts using techniques like ground-penetrating radar, thermal imaging, and aerial LiDAR surveys.
- Understand urban dynamics by mapping socioeconomic data on race, income, education, language, crime etc.
- Analyze spatial diffusion patterns of languages, religions, innovations, social movements and cultural traits.
- Study geographic segregation, polarization, and inequalities.
- Establish territorial boundaries and interactions between groups based on archaeological records.
How Does Geospatial Analysis Work?
While specific techniques and tools vary across applications, the general geospatial analysis workflow contains the following steps:
Defining the Problem:
First, identify the specific question, decision or problem that requires spatial examination. This may relate to site selection, pattern analysis, predictive modeling, or other location-based needs. Clear objectives are necessary to plan the appropriate analytical approach.
Data Acquisition:
Next, relevant geospatial data must be collected from various primary and secondary sources. Key data collection methods include:
- GPS devices, radars, satellites, drones, sensors etc. to capture new field measurements.
- Surveys, interviews, field observation etc. to gather first-hand spatial data.
- Databases, government agencies, online repositories to obtain existing geospatial datasets.
- Techniques like geocoding to convert addresses into lat-long coordinates.
- Data integration from multiple sources into a GIS for further processing and analysis.
Pre-processing and Quality Checks:
Collected spatial data needs to be formatted, cleaned, and enhanced to resolve any errors, gaps or inconsistencies:
- Data is checked for completeness, accuracy, reliability and transformed into usable structures.
- Geometric corrections are applied to raw geospatial data to align and scale it appropriately.
- Duplicates are removed, metadata added, projections aligned to enable data integration.
- Spatial and temporal resolution may be standardized across datasets.
Exploratory Analysis:
Preliminary visual and statistical analysis is conducted to become familiar with spatial patterns, relationships and anomalies in the data:
- Interactive mapping provides a big picture overview of the geographical distribution.
- Basic analytics like mean center, directional distribution, nearest neighbour analysis quantifies spatial characteristics.
- Outlier detection, clustering, and hot spot analysis reveal interesting patterns.
- Spatial joins combine attribute data from different layers based on location.
Advanced Modeling and Predictions:
Sophisticated computational techniques are applied to achieve the desired spatial insights:
- Simulation models analyze scenarios and forecast impacts of spatial phenomena.
- Spatial statistics quantify patterns and correlations between variables.
- Geospatial machine learning develops location-based predictive models.
- Routing and logistics optimization algorithms solve spatial network problems.
- Image analysis extracts information from aerial photos and satellite imagery.
Visualization and Interpretation:
Results are translated into understandable, actionable insights using:
- Maps, infographics, and spatial dashboards tailored for the audience.
- Interactive web maps that allow self-directed exploration.
- 3D displays, AR/VR environments to immerse users in landscapes.
- Animations showing phenomena over time and space.
Communication and Results:
Finally, geospatial analysis results guide decisions, policy, operations and research in the application domain. Maps and spatial models may be deployed through apps and platforms. Over time, new data is incorporated to provide updated, ongoing location intelligence.
In summary, geospatial analysis typically follows a workflow that progresses from spatial data acquisition to problem-focused analysis and impactful visualization.
Key Skills required for Geospatial Analysis
A combination of technical and domain expertise is required to conduct impactful geospatial analysis:
- GIS skills: Proficiency with GIS software for spatial data management, analysis and cartography.
- Statistics and geospatial modeling: Expertise in statistical analysis methods for spatial data.
- Programming: For automated data processing, geospatial computing, and development of custom tools.
- Domain knowledge: Understanding of the subject matter and nuances of the application industry.
- Data science: Data mining, machine learning, algorithm design skills to handle large, complex geospatial data.
- Visualization: Ability to effectively communicate spatial insights through maps, 3D, infographics etc.
- Remote sensing: Interpreting and analyzing imagery from satellites, aerial photos, LiDAR etc.
- Cartography: Designing static and interactive maps that intuitively convey information and insights.
Many powerful geospatial analysis software options exist spanning open source, commercial, and cloud-based solutions:
- ESRI ArcGIS – The industry-leading commercial GIS software with advanced analysis capabilities.
- QGIS – Open source GIS popular for its flexibility and extensive functionality.
- Google Earth Engine – Cloud-based geospatial analysis using Google’s public geospatial datasets.
- GeoServer – Open source platform for publishing spatial data as web services.
- GRASS GIS – Open source GIS focused on raster processing and analysis.
- GeoDa – Specialized tool for spatial statistical analysis and geovisualization.
- R Spatial Packages – Libraries like sf, raster, spatstat for geospatial analysis using R.
- PostgreSQL/PostGIS – Extends PostgreSQL databases with robust spatial data support.
- Python Geospatial Libraries – Modules like Geopandas, PySAL, and Rasterio for geospatial data science.
Many GIS tools like QGIS also integrate with external environments like R and Python for advanced analysis. Cloud computing provides the power to apply geospatial analysis at massive scales.
The Future of Geospatial Analysis
Some emerging directions that will shape the next generation of geospatial analytics include:
- Big data and cloud computing – Applying high performance geospatial analytics on vast datasets in the cloud.
- Real-time data – Incorporating streaming data from sensors, drones, apps for dynamic insights.
- AI and Machine Learning – Automating identification of features, predictive modeling, decision optimization from spatial data.
- 3D/4D mapping – Rich 3D maps overlaid with temporal data enable new insights and immersive experiences.
- Augmented reality – Overlaying digital information and analytics on real-world scenes provides intuitive exploration.
- Open data – Consuming and combining publicly available geospatial data fuels innovation.
- Mobile mapping – Smartphones, apps and crowd-sourced data expand collection and usage.
Geospatial capabilities will increasingly be embedded into everyday apps and commercial tools, empowering everyone to leverage location-based intelligence.
Conclusion
Geospatial analysis offers an indispensible lens to uncover powerful insights from location-based data.
Geospatial Analysis- FAQs
Q. What are some commonly used geospatial analysis tools?
Some popular geospatial analysis tools and technologies include ArcGIS, QGIS, GeoServer, PostGIS, Leaflet, Mapbox, Google Earth Engine.
Q. What skills are required to perform geospatial analysis?
Key skills include GIS, spatial analysis, data science, programming, geostatistics, cartography, remote sensing, database management.
Q. How is geospatial analysis used in public health?
It helps track disease outbreaks, identify access barriers to health services, analyze environmental hazards – ultimately improving healthcare planning and service delivery.
Q. What is spatial interpolation in geospatial analysis?
It is a method of estimating unknown values for any geographic point from surrounding known values. It helps predict trends in areas with sparse measurements.
Q. What are some limitations of geospatial analysis?
Potential limitations include inaccurate/incomplete data, simplistic assumptions, over-reliance on software, not factoring in local knowledge of geography.
Q. What is a geospatial information system?
It is a framework for gathering, managing, analyzing, and visualizing geospatial data. GIS integrates map layers, analyzes spatial relationships, and enables location-based decision making.
Share your thoughts in the comments
Please Login to comment...