Step into the dynamic world of Data Visualization Interview Questions, where the power of visual storytelling meets the precision of data analysis. In today’s data-driven world, the ability to effectively communicate insights through visualization is a coveted skill sought after by employers across various industries. As organizations increasingly rely on data to guide decision-making processes, professionals proficient in data visualization play a vital role in transforming complex datasets into actionable intelligence.
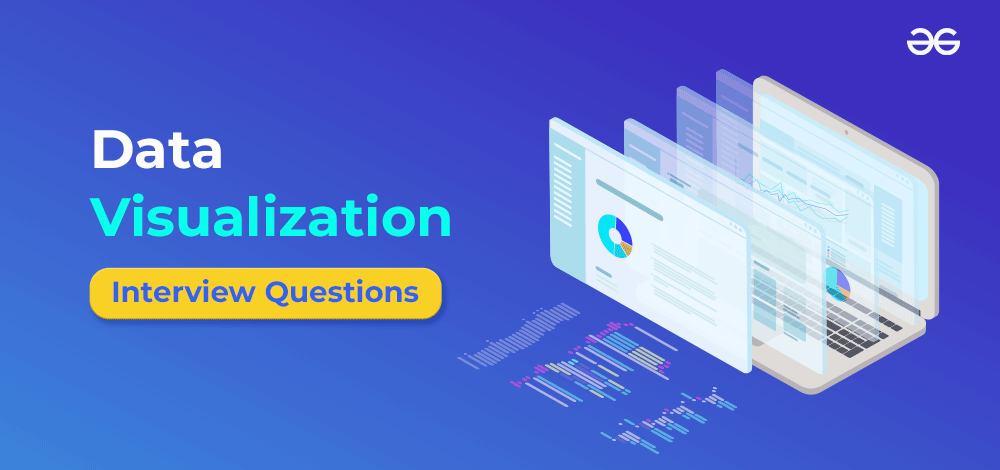
Data Visualization Interview Questions
This article serves as a comprehensive guide for both aspiring data visualization experts and seasoned practitioners preparing for interviews. Through a curated selection of insightful questions, we delve into the fundamental principles, advanced techniques, and real-world applications of data visualization. Whether you’re exploring the intricacies of chart design, mastering visualization tools and platforms, or navigating the nuances of storytelling with data, this resource equips you with the knowledge and confidence to excel in interviews and beyond.
Data Visualization Interview Questions And Answer
- Q.1 What is data visualization, and why is it important?
- Q.2 What are the key components of good data visualization?
- Q.3 How can colour be utilized in data visualization?
- Q.4 What are the different types of data visualizations?
- Q.5 What is a bar chart, and when it is typically used for data visualization?
- Q.6 Define outliers and discuss potential methods for handling them.
- Q.7 How do you choose the appropriate visualization type for your data?
- Q.8 What is the importance of storytelling in data visualization?
- Q.9 How can you choose an appropriate color palette for your visualizations?
- Q.10 What are some common mistakes to avoid when creating data visualizations?
- Q.11 How can you assess the effectiveness of data visualization?
- Q.13 Describe the concept of data-ink ratio in data visualization.
- Q.14 What is the purpose of a legend in a chart or graph?
- Q.15 What is a pie chart, and when is it suitable for visualizing data?
- Q.16 Explain the main elements of a pie chart.
- Q.17 What is a line chart, and when is it commonly employed for data visualization?
- Q.18 Describe the components of a line chart.
- Q.19 What is a scatter plot, and under what circumstances would you use it for data visualization?
- Q.20 Explain the key elements of a scatter plot.
- Q.21 What is a histogram, and when is it employed for data visualization?
- Q.22 Describe the essential features of a histogram.
- Q.23 What is a heatmap, and when is it useful for data visualization?
- Q.24 Explain the primary components of a heatmap.
- Q.25 What is a box plot and why is it used for data visualization?
- Q.26Explain the differences between descriptive and inferential statistics.
- Q.27 What is the purpose of a box plot in statistics visualization.
- Q.28 When is a quantile-quantile (Q-Q) plot used in statistics, and how does it help assess the normality of a dataset?
- Q.29 What is a heat map, and how is it useful for visualizing correlations and patterns in a matrix of data in statistics?
- Q.30 Describe the purpose of a violin plot in statistics visualization.
- Q.31 What is univariate data visualization, and why is it important in data analysis?
- Q.32 Describe the purpose of a density plot in univariate data visualization.
- Q.33 What are the different plots used for univaraite analysis
- Q. 34 What is bubble chart?
- Q.35 What is a grouped bar chart?
- Q.36 Explain the importance of data visualization in statistics.
- Q.37 What are some common methods for visualizing correlations between variables?
- Q.38 How can you determine if a dataset follows a normal distribution using visualizations?
- Q.39 What is the key advantage of using a logarithmic scale in a visualization?
- Q.40 When would you choose a bar chart over a pie chart for displaying categorical data?
- Q.41 What is the primary difference between a line chart and a scatter plot.
- Q.42 What does the term “overplotting” mean in the context of scatter plots?
- Q.43 Why is it important to consider colorblindness when designing visualizations?
- Q.44 What is the purpose of jitter in scatter plots?
- Q.45 Explain the concept of a “word cloud” in text data visualization.
- Q.46 What is the significance of word size and color in a word cloud?
- Q.47 How can you address the issue of word overlap or crowding in a word cloud?
- Q.48 What are the main limitations of using word clouds for text analysis?
- Q.49 What is the difference between a word cloud and a tag cloud?
- Q.50 What are some alternatives to word clouds for visualizing text data?
Q.1 What is data visualization, and why is it important?
Data visualization is the graphical representation of data to help individuals, organizations, and analysts to better understand patterns, trends, and insights within the data. It involves the use of visual elements like charts, graphs, maps, and infographics to convey complex information in a more accessible and comprehensible format.
Q.2 What are the key components of good data visualization?
Effectively communicating knowledge and insights while being simple to understand and aesthetically beautiful are all qualities of successful data visualization. A strong data visualization should have the following critical elements:
- Data Accuracy
- Clear and Relevant Title
- Appropriate Visual Representation
- Data Labels and Legends
- Consistent Scale and Units.
Q.3 How can colour be utilized in data visualization?
In data visualisation, colour is a potent tool that can improve comprehension, draw attention to patterns, and effectively communicate ideas. When applied carefully, colour may increase the interest and clarity of your data visualisation. Following are some examples of how colour can be used in data visualisation:
- Differentiating Categories or Groups
- Highlighting Data Points or Trends
- Gradient Scales
- Colour Coding for Meaning
- Colour Legends and Labels
Q.4 What are the different types of data visualizations?
Data visualisations come in a variety of forms, each of which is intended to effectively communicate a particular type of knowledge and insight. Here are a few examples of prevalent data visualisations:
- Bar Charts:Bar charts use rectangular bars to represent data values, making them suitable for comparing data across categories or groups.
- Line Charts: Line charts display data points connected by lines, making them useful for showing trends and changes over time.
- Scatter Plots: Scatter plots use individual data points to display the relationship between two continuous variables, making them helpful for identifying correlations or patterns.
- Pie Charts: Pie charts represent parts of a whole, with each slice of the pie corresponding to a percentage or proportion of the total.
- Histograms: Histograms display the distribution of a single variable’s values, showing how data is distributed across different bins or intervals.
- Box Plots: Box plots provide a summary of the distribution of data, including measures such as the median, quartiles, and potential outliers.
- Heatmaps: Heatmaps use color to represent data values in a grid, making them suitable for visualizing correlations or patterns in large datasets.
- Treemaps: Treemaps represent hierarchical data structures, such as the organization of files on a computer, using nested rectangles.
- Sankey Diagrams: Sankey diagrams illustrate the flow or distribution of data between categories or entities, often used in energy or resource analysis.
- Bubble Charts: Bubble charts extend scatter plots by using bubbles of varying sizes to represent data points, with the size of the bubble indicating an additional variable.
- Choropleth Maps: Choropleth maps use color-coding to represent data values in geographic regions, making them useful for visualizing regional data.
- Parallel Coordinates Plots: Parallel coordinates plots visualize multivariate data by representing each data point as a line crossing parallel axes.
- Waterfall Charts: Waterfall charts display incremental changes in data values, commonly used for financial or budget analysis.
- Radar Charts (Spider Charts): Radar charts display data points on a circular grid, making them useful for comparing multiple variables across different categories.
- Network Diagrams: Network diagrams illustrate relationships between entities in a network, such as social networks or transportation systems.
- Word Clouds: Word clouds visually represent the frequency of words in a text, with more frequently occurring words displayed in larger text.
- Bullet Graphs: Bullet graphs provide a compact way to display a single data point in relation to a target or benchmarks, often used in dashboards.
- Sunburst Charts: Sunburst charts display hierarchical data in a radial layout, with segments representing parent and child categories.
- 3D Plots: 3D plots add a third dimension to 2D plots, allowing for the visualization of data in three-dimensional space.
These are just some of the data visualization types. The choice of visualization method depends on the nature of the data, the goals of the analysis, and the audience’s needs for understanding the information presented.
Q.5 What is a bar chart, and when it is typically used for data visualization?
A bar chart, also called a bar graph, is a tool for data visualisation. Each bar in a bar chart is proportional to the value it displays in terms of height or length. The bars are normally aligned along an axis either horizontally or vertically.
Here are some of the main key components of a bar chart.
- Bars: These are the rectangular elements that visually represent the data values. The length or height of each bar corresponds to the magnitude of the data it represents.
- Axes: A bar chart usually has two axes: a vertical or y-axis (on the left or bottom) and a horizontal or x-axis (on the bottom or left). The y-axis typically represents the data values, while the x-axis represents categories or data points.
- Labels: The axes are labeled to indicate the scale and the categories being represented. The bars may also have data labels or values at their endpoints.
Bar charts are typically used for the following purposes in data visualization:
- Comparing Categories
- Displaying Discrete Data
- Showing Rankings
- Tracking Changes Over Time
- Part-to-Whole Relationships
Q.6 Define outliers and discuss potential methods for handling them.
Outliers are the data point that significantly different from the rest of the data points. Outliers can occur for various reasons, including data entry errors, measurement errors, natural variation, or the presence of rare events. Identifying and handling outliers is important in data analysis because they can have a significant impact on statistical analyses and machine learning models.
Here are some methods for handling outliers:
- Data Trimming
- Data Transformation
- Robust Statistical Methods
- Machine Learning Models
- Visualization
- Ensemble Methods
Q.7 How do you choose the appropriate visualization type for your data?
It is important to carefully analyse the nature of the data, the objectives of the research, and the audience you’re attempting to reach before selecting the right visualisation method for your data. Here is a step-by-step tutorial to assist you in selecting the best option:
- Understand Your Data
- Identify Your Goals
- Consider Your Audience
- Choose the Right Chart Type
- Document and Explain
Q.8 What is the importance of storytelling in data visualization?
Storytelling is a crucial aspect of data visualization because it transforms raw data into a compelling narrative that can inform, persuade, and engage the audience. Here are several reasons why storytelling is important in data visualization.
- Contextualization
- Clarity and Comprehension
- Engagement
- Emotional Connection
- Memory Retention
- Decision-Making
Q.9 How can you choose an appropriate color palette for your visualizations?
Choosing an appropriate color palette for our visualizations is crucial for ensuring clarity, readability, and effective communication of data. Here’s a step-by-step guide on how to choose a suitable color palette:
- Understand the Data and Context
- Consider Color Meaning and Symbolism
- Ensure Accessibility
- Start with a Base Color
- Select Additional Colors
Q.10 What are some common mistakes to avoid when creating data visualizations?
Creating effective data visualizations requires careful attention to detail and thoughtful design choices. Here are some common mistakes to avoid when creating data visualizations:
- Misleading Scaling: Misrepresenting the scale of axes or using inconsistent scales can distort the data and lead to incorrect interpretations. Ensure that scales accurately reflect the data.
- Incomplete or Missing Labels: Labels on axes, data points, and legends are essential for context. Missing or incomplete labels can confuse viewers and hinder understanding.
- Overloading with Data: Avoid cluttering your visualization with too much information. Overloading with data points, labels, or details can overwhelm the audience and reduce clarity.
- Non-Zero Baseline for Bar Charts: When using bar charts, make sure the baseline starts at zero. Truncated axes can exaggerate differences and mislead viewers.
- Ignoring Data Outliers: Ignoring or mishandling outliers in your visualization can lead to skewed perceptions of the data. Consider whether to address or mention outliers, depending on their relevance.
- Inadequate Data Cleaning: Failure to clean and preprocess data before visualization can result in inaccuracies and visual artifacts. Ensure data quality and consistency.
Q.11 How can you assess the effectiveness of data visualization?
Assessing the effectiveness of data visualization involves evaluating how well it achieves its intended goals, communicates insights, and engages the audience. Here are several methods and considerations for assessing the effectiveness of your data visualization:
- Clearly Defined Objectives
- Audience Feedback
- Usability Testing
- Objective Metrics
- Comparative Analysis
Q.13 Describe the concept of data-ink ratio in data visualization.
The concept of the data-ink ratio is a principle introduced by Edward Tufte, a prominent expert in data visualization. It emphasizes the idea that in a data visualization, every piece of ink or pixel used to represent data should contribute directly to the audience’s understanding of the information. In other words, unnecessary ink or non-data ink should be minimized to maximize the efficiency and clarity of the visualization.
Here are key components and principles related to the data-ink ratio:
- Data-Ink
- Non-Data Ink
- Maximizing Data-Ink
- Simplicity and Clarity
- Enhancing Readability
Q.14 What is the purpose of a legend in a chart or graph?
A chart or graph’s legend serves as a guide or explanation for the different data series or components displayed in the visualisation. It aids the viewer in comprehending the significance of the many hues, symbols, or lines used to represent various data categories, variables, or groupings in the chart or graph.
Q.15 What is a pie chart, and when is it suitable for visualizing data?
The circular data visualisation tool known as a pie chart shows data as a segmented circle, with each segment (or “slice”) denoting a certain category or percentage of the overall data. Each segment’s size is proportionate to the amount or percentage it contributes to the dataset. In situations when the categories are distinct and do not follow a logical order, pie charts are frequently used to depict categorical or nominal data.
When to Use Pie Charts:
- Showing Part-to-Whole Relationships
- Comparing Categories
- Highlighting Percentages
- Simple Data Structures
- Visual Appeal
Q.16 Explain the main elements of a pie chart.
A pie chart consists of several main elements that work together to visually represent data as a circular graph. Understanding these elements is essential for interpreting and creating pie charts effectively. Here are the key components of a pie chart:
- Circle (or Pie)
- Slices (Segments)
- Central Angle
- Category Labels
- Data Labels
- Legend
- Title
- Exploded or Offset Slices
- Colors
- Lines or Leader Lines
Q.17 What is a line chart, and when is it commonly employed for data visualization?
A style of data visualisation called a line chart shows data points connected by straight lines. It is especially useful for identifying trends, patterns, and relationships in time-series data since it is frequently used to represent data that changes continuously over a predetermined period or sequence. Line graphs are another name for line charts.
Common Use Cases for Line Charts:
- Time-Series Data
- Trend Analysis
- Comparing Multiple Data Series
- Forecasting
- Performance Metrics
- Scientific Data
- Economic and Financial Data
- Population and Demographic Trends
Q.18 Describe the components of a line chart.
A line chart consists of several components that work together to visually represent data and convey trends or patterns effectively. Understanding these components is essential for interpreting and creating line charts. Here are the key components of a typical line chart:
- Title
- X-Axis (Horizontal Axis)
- Y-Axis (Vertical Axis)
- Axis Labels
- Data Points
Q.19 What is a scatter plot, and under what circumstances would you use it for data visualization?
Individual data points can be seen on a two-dimensional graph using a technique called a scatter plot. The values of two variables, one depicted on the horizontal (X) axis and the other on the vertical (Y) axis, are represented by each data point on the scatter plot. The relationship, correlation, or dispersion of data points between two variables can be visualised using scatter plots.
Characteristics of Scatter Plots:
- Two Variables
- Data Points
- No Connecting Lines
- Variable Scales
Q.20 Explain the key elements of a scatter plot.
A scatter plot consists of several key elements that work together to visually represent the relationship between two variables. Understanding these elements is essential for interpreting and creating scatter plots effectively. Here are the key components of a typical scatter plot:
- Title
- X-Axis (Horizontal Axis)
- Y-Axis (Vertical Axis)
- Axis Labels
- Data Points
Q.21 What is a histogram, and when is it employed for data visualization?
A histogram is a graph that shows how a dataset is distributed. It shows the frequency or count of data points along a continuous range that fall into predetermined intervals or “bins”. Histograms are frequently used to visualise the frequency and distribution of numerical data, which makes them very helpful for examining trends and traits in datasets.
Common Use Cases for Histograms:
- Data Distribution Analysis
- Frequency Count
- Outlier Detection
- Data Transformation
- Quality Control
- Statistical Analysis
Q.22 Describe the essential features of a histogram.
A histogram is a graphical representation of the distribution of a dataset, displaying the frequency or count of data points within specified intervals or “bins” along a continuous range. To understand and interpret a histogram effectively, it’s important to be familiar with its essential features. Here are the key components and features of a histogram:
- Bins or Intervals
- Frequency or Count
- Continuous Scale
Q.23 What is a heatmap, and when is it useful for data visualization?
A heatmap is a data visualization technique that uses colors to represent the values of a matrix or a table of data. It is particularly useful for visualizing patterns, relationships, and variations in data, especially when dealing with large datasets or data organized in a two-dimensional format. Heatmaps are versatile and can be applied to various types of data analysis.
- Common Use Cases for Heatmaps
- Genomic Data Analysis
- Website User Behavior
- Financial Data Analysis
- Sports Analytics
Q.24 Explain the primary components of a heatmap.
A heatmap is a data visualization that uses color to represent the values of a matrix or a table of data. It consists of several primary components that work together to convey information effectively. Understanding these components is crucial for interpreting and creating heatmaps. Here are the primary components of a heatmap:
- Color Scale
- Matrix of Data
- Row Labels and Column Labels
- X-Axis and Y-Axis
- Color Legend
Q.25 What is a box plot and why is it used for data visualization?
A box plot, also known as a box-and-whisker plot, is a graphical representation of a dataset’s distribution and central tendency. It is used to visualize the spread, variability, and potential outliers within the data. Box plots are particularly useful for comparing multiple datasets or identifying patterns in a single dataset.
Reasons for Using Box Plots
- Summary of Data Distribution
- Comparison of Distributions
- Identification of Skewness
- Detection of Outliers
- Robustness to Extreme Values
- Statistical Insights
Q.26Explain the differences between descriptive and inferential statistics.
Descriptive statistics and inferential statistics are two branches of statistics used to analyze and interpret data. They serve different purposes and employ distinct methods. Here are the key differences between descriptive and inferential statistics:
Function
|
Descriptive Statistics
|
inferential statistics
|
Purpose
|
Descriptive statistics are used to summarize, describe, and present data in a meaningful and understandable way.
|
Inferential statistics are used to make inferences, predictions, or generalizations about a population based on a sample of data.
|
Data Usage
|
Descriptive statistics focus on the data that are available and provide a summary of these data.
|
Inferential statistics use sample data to make inferences about a larger population.
|
Methods
|
Descriptive statistics use various measures and techniques to describe the characteristics of data.
|
Inferential statistics involve hypothesis testing, confidence intervals, regression analysis, and various statistical tests.
|
Q.27 What is the purpose of a box plot in statistics visualization.
A box plot, commonly referred to as a box-and-whisker plot, is a graphical representation used in statistics to show summary statistics, such as measures of central tendency and spread, and to visualise the distribution of a dataset.
Q.28 When is a quantile-quantile (Q-Q) plot used in statistics, and how does it help assess the normality of a dataset?
A Quantile-Quantile (Q-Q) plot is a statistical visual aid for evaluating the normality or closeness of a dataset’s distribution to a theoretical normal distribution. When determining if your dataset follows a normal (Gaussian) distribution or any other particular distribution, it is especially helpful.
Here’s how a Q-Q plot works and how it helps assess the normality of a dataset:
- Basic Concept
- Procedure
- Interpretation
- Assessing Normality
- Outliers
Q.29 What is a heat map, and how is it useful for visualizing correlations and patterns in a matrix of data in statistics?
A heatmap is a type of graphic that uses colour to show a data matrix’s values. When dealing with numerical or categorical data structured in a matrix or table, heatmaps are extremely helpful for visualising relationships and patterns within huge datasets. For the following reasons, they are frequently used in statistics, data analysis, and data visualisation:
- Correlation Analysis
- Pattern Recognition
- Data Comparison
- Hierarchical Clustering
- Anomaly Detection
- Decision-Making
Q.30 Describe the purpose of a violin plot in statistics visualization.
A violin plot is a data visualisation technique used in statistics to show the distribution of a dataset and reveal both its underlying probability density function (PDF) and summary statistics. Its major objective is to combine elements of a kernel density plot and a box plot, providing a more thorough understanding of the data distribution. A violin plan has the following objectives and elements:
Q.31 What is univariate data visualization, and why is it important in data analysis?
A approach for exploring and displaying the distribution and properties of a single variable or one-dimensional dataset is called univariate data visualisation. Without taking into account its relationships with other variables, univariate data visualisation focuses on helping you comprehend the characteristics and patterns of a single variable. Data analysis requires this kind of visualisation for a number of reasons:
- Data Exploration
- Summary Statistics
- Identifying Patterns and Trends
- Outlier Detection
- Distribution Assessment
- Variable Transformation
Q.32 Describe the purpose of a density plot in univariate data visualization.
The probability density function (PDF) of a continuous variable can be calculated and displayed using a density plot, sometimes referred to as a kernel density plot. Its main objective is to visualise the distribution of a single variable and reveal information about the underlying data distribution. The function and properties of a density plot in univariate data visualisation are described as follows:
- Estimation of Probability Density
- Smoothed Curve
- Visualizing Distribution Shape
- Complementing Histograms
- Probability and Relative Likelihood
Q.33 What are the different plots used for univaraite analysis
Univariate analysis focuses on exploring and summarizing a single variable at a time. There are several common types of plots and visualizations used in univariate analysis to gain insights into the distribution and characteristics of a single variable. Here are some of the most commonly used univariate plots:
- Histogram
- Box Plot (Box-and-Whisker Plot)
- Density Plot (Kernel Density Plot)
- Bar Chart
- Frequency Plot
- Pie Chart
- Dot Plot
- Violin Plot
- Time Series Plot
- Probability Plot (Q-Q Plot)
Q. 34 What is bubble chart?
A bubble chart is a data visualization technique used to display three-dimensional data in a two-dimensional space. It is an extension of a scatter plot, where each data point is represented as a circle (or “bubble”) on a two-dimensional coordinate system, with the size of the circle indicating a third variable.
Q.35 What is a grouped bar chart?
A grouped bar chart, also known as a clustered bar chart, is a type of data visualization used to display and compare data for multiple categories or groups across two or more subcategories or variables. It is an extension of a standard bar chart, where bars are grouped together to show the relationships between multiple sets of data within each category or group.
Q.36 Explain the importance of data visualization in statistics.
Data visualization is a vital component of statistics that enhances data exploration, communication, and decision-making. It transforms raw data into actionable insights, making statistics more accessible and impactful in various domains. Effective visualization can lead to better-informed decisions and a deeper understanding of data patterns and relationships.
Q.37 What are some common methods for visualizing correlations between variables?
Visualizing correlations between variables is essential for understanding relationships and dependencies in data. Several common methods for visualizing correlations between variables include:
- Scatter Plots
- Correlation Matrix Heatmap
- Correlation Matrix Dendrogram
- Scatterplot Matrix
- Line Plots with Multiple Variables
- Bubble Charts
- Correlograms
- Parallel Coordinates Plot
Q.38 How can you determine if a dataset follows a normal distribution using visualizations?
To determine if a dataset follows a normal distribution using visualizations, you can use various graphical tools and techniques to assess the distribution’s shape and characteristics. While visual inspection is not a formal statistical test for normality, it can provide valuable insights. Here’s how you can use visualizations to assess normality:
- Histogram
- Probability Plot (Q-Q Plot)
- Normal Probability Plot (P-P Plot)
- Kernel Density Plot
- Box Plot
Q.39 What is the key advantage of using a logarithmic scale in a visualization?
The key advantage of using a logarithmic scale in a visualization is its ability to effectively represent and visualize data that spans a wide range of values or exhibits exponential growth or decay.
Q.40 When would you choose a bar chart over a pie chart for displaying categorical data?
Choosing between a bar chart and a pie chart for displaying categorical data depends on the nature of the data and the specific message you want to convey. Here are some situations in which you would prefer a bar chart over a pie chart:
- Comparing Categories
- Showing Relative Magnitudes
- Handling Many Categories
- Displaying Ranking
- Showing Trends Over Time
Q.41 What is the primary difference between a line chart and a scatter plot.
A line chart connects data points with lines and is ideal for visualizing trends or changes in data over a continuous scale or time. It is commonly used for time-series data, such as stock prices or temperature variations.
Scatter Plot: A scatter plot represents individual data points as unconnected dots, making it suitable for showing the relationship or correlation between two continuous variables. It helps identify patterns, clusters, or outliers in the data.
Key Difference: The primary distinction is that a line chart emphasizes connected data points to depict trends, while a scatter plot displays unconnected data points to reveal relationships between two variables without assuming a specific sequence.
Q.42 What does the term “overplotting” mean in the context of scatter plots?
“overplotting” refers to a situation where multiple data points on the plot overlap or occupy the same or nearly the same position on the graph. Overplotting can occur when you have a large number of data points or when the data values are tightly clustered, making it difficult to discern individual points.
Q.43 Why is it important to consider colorblindness when designing visualizations?
Considering colorblindness in visualization design is essential for inclusivity, effective communication, and avoiding misinterpretation. Approximately 8% of the population has some form of color vision deficiency, so using distinguishable color palettes, adding labels and annotations, and providing alternative representations ensures that visualizations are accessible and informative to a broader audience. Testing for accessibility and promoting awareness of colorblindness are also crucial steps in creating inclusive visualizations.
Q.44 What is the purpose of jitter in scatter plots?
The purpose of jitter in scatter plots is to add a small amount of random noise or displacement to the data points along one or both axes. This is done to prevent overplotting, which occurs when multiple data points share the same or very close coordinates, making it difficult to discern individual points.
Q.45 Explain the concept of a “word cloud” in text data visualization.
A “word cloud” is a text data visualization technique used to represent the frequency or importance of words in a given text or document. In a word cloud, words are displayed graphically, and their size or prominence is determined by their frequency or significance within the text. The more frequently a word appears in the text, the larger and more prominent it appears in the word cloud.
Q.46 What is the significance of word size and color in a word cloud?
Word size and color in a word cloud serve as effective visual cues to highlight word frequency, importance, and categorical information. When used appropriately, they enhance the readability and informativeness of the word cloud, aiding in the quick understanding of key insights within the textual data.
Q.47 How can you address the issue of word overlap or crowding in a word cloud?
The significance of word size and color in a word cloud lies in their role in visually representing the importance or prominence of words within a given text or dataset. These visual attributes are essential for conveying information and insights in a word cloud.
Q.48 What are the main limitations of using word clouds for text analysis?
Loss of Context: Word clouds don’t capture the context in which words appear, leading to a loss of critical information and nuances in meaning.
Limited Vocabulary: They display only the most frequent words, excluding potentially meaningful terms, resulting in a biased representation.
Equal Treatment of Words: All words are treated equally, regardless of their importance or relevance, which can be misleading and overlook significant terms.
Q.49 What is the difference between a word cloud and a tag cloud?
Word Cloud: Emphasizes word frequency in a given text, with word size based on frequency, typically used for exploratory analysis.
Tag Cloud: Displays keywords or tags associated with a collection of content, with tag size and style reflecting importance or relevance within a specific context, often used in information retrieval systems.
Visual Cues: Word clouds use minimal visual cues, while tag clouds may incorporate color and interactivity to convey context-specific information and enable user interactions.
Q.50 What are some alternatives to word clouds for visualizing text data?
- Bar Charts and Histograms
- Word Frequency Tables
- Heatmaps
- Word Cloud Variations
- Topic Modeling
Share your thoughts in the comments
Please Login to comment...