What is Data Lifecycle Management?
Last Updated :
24 Jan, 2024
In the digital era, data has become the most valuable currency driving competitive advantage and organizational success. But without systematic governance, relentless data growth leads to uncontrolled chaos. This exponential flood of data flowing into disparate lakes and silos creates overwhelming complexity and risk. This is where implementing comprehensive data lifecycle management becomes critical. Data lifecycle management provides end-to-end visibility and control over the data flowing through systems and processes. It enables harnessing data as an asset at every stage – from planning through active use and eventual retirement. With strong data lifecycle management, organizations can channel data into a strategic advantage rather than suffer from overwhelming disarray.
This article will explore the key stages, practices, benefits, and implementation steps for robust data lifecycle management programs. With these foundations, organizations can transform even exponentially growing data from an unruly liability into a trustworthy and adaptable asset powering competitive advantage. Taming the data deluge through lifecycle management unlocks lasting business value.
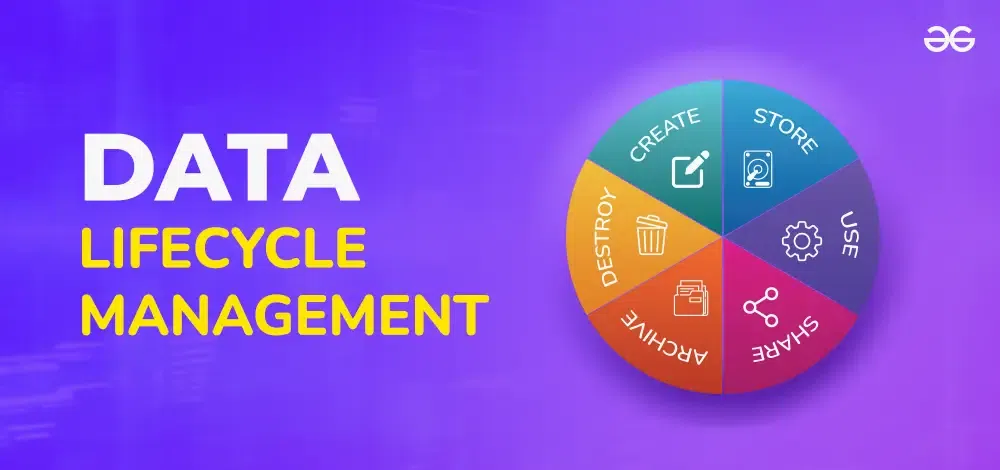
Data LIfecycle Management System
The Critical Role of Data Lifecycle Management in the Era of Big Data
With data volume, variety, and velocity all exploding exponentially, effectively managing data has become a top priority. However static, siloed data lakes provide little strategic value. This is where implementing comprehensive data lifecycle management becomes critical – to keep pace with relentless data growth and complexity. When executed well, data lifecycle management delivers continuous visibility and control over the data deluge. It enables harnessing data as an asset for competitive advantage.
What is data Lifecycle management ?
Data lifecycle management refers to the holistic set of practices for governing data from inception through retirement across the entire organization. It focuses on managing data seamlessly through all phases of its useful life – from planning and creation to processing, storage, utilization, archival, and deletion. Robust policies and procedures systematically cover data security, integrity, usability, costs, and regulatory compliance at each stage.
Main Phases of Data Lifecycle Management
Here are the main phases of the data lifecycle management process:
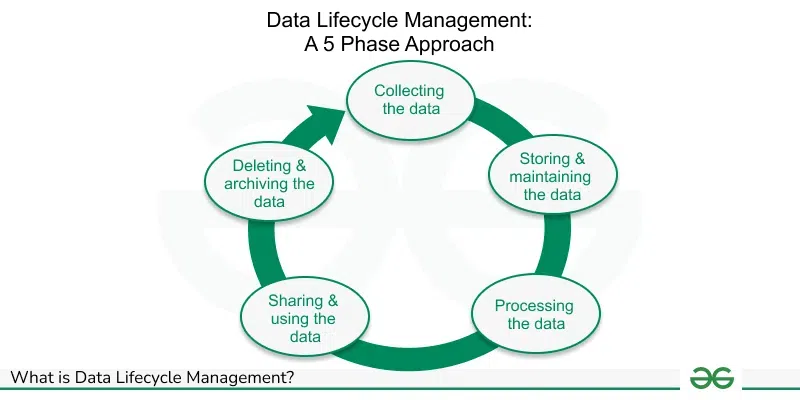
Data Lifecycle Management
- Data Generation and Collection: Strategize new data needs, sources, architecture standards, and policies. Get required approvals. This initial phase involves identifying the data sources and requirements, then collecting or generating the raw data from those sources. Potential data sources could include databases, file systems, software applications, social media APIs, sensors, etc.
- Data Processing and Cleaning: Once data is acquired, it needs to be processed and cleaned to remove errors, inconsistencies, duplicates, and irrelevant records. Tasks in this phase can involve data validation, normalization, formatting, deduplication, etc. Quality checks are performed to ensure the data is accurate and reliable.
- Data Analysis: In this phase, different techniques are applied to analyze, interpret, and model the data to discover useful patterns, insights, and knowledge. Analytics techniques like descriptive, diagnostic, predictive, and prescriptive modeling are utilized based on the specific business needs.
- Data Visualization and Reporting: Data analysis results need to be summarized and translated into a human-understandable format via data visualizations, dashboards, and reporting. Effective presentation is key for stakeholders to absorb the information and direct strategy.
- Data Storage and Archiving: Processed and analyzed data needs to be stored and managed efficiently. Data archiving policies determine what data should be actively accessed vs. archived offline or deleted based on usage and legal requirements. Database management ensures high availability, backup, and retrieval.
- Data Access and Distribution: In this phase, data access permissions, protocols, and interfaces are managed to facilitate discovery and sharing of data assets across the organization and with external stakeholders when appropriate. APIs, queries, and data catalogs enable distribution.
- Data Security and Governance: Robust security measures, policies, standards, and controls are implemented throughout the data lifecycle to ensure compliance with regulations. Data governance defines the roles and responsibilities around usage of data.
Data Lifecycle Management Capabilities
- Centralized policies and standards for managing data end-to-end.
- Metadata tracking from data origins through processing and usage.
- Data quality and validity monitoring and alerts.
- Automated data archiving, retention, and disposal tools.
- Storage infrastructure optimization for cost and performance.
- Backup and disaster recovery provisions for data availability.
- Data integration, preparation, and delivery pipelines.
- Cataloging of available data and lineage for discovery.
- Roles and responsibilities for distributed data stewardship.
- Security controls aligned to classification levels, users, and environments.
Benefits of Data Lifecycle Management
Effective data lifecycle management confers multiple benefits:
- Greater Agility – Smooth data pipelines allow faster utilization of new data for enhanced decision making.
- Risk Reduction – Continuous controls improve data integrity, compliance, availability, and recovery.
- Storage Efficiency – Unneeded data can be automatically removed per retention policies to reduce costs.
- Productivity – Self-service access to high quality data accelerates analysis and insights.
- Innovation – Better enterprise data knowledge enables identifying new opportunities and applications.
- Trust – Documented data provenance provides confidence in analytical outcomes.
- Future-Proofing – Adaptable data architectures withstand evolving technologies and needs.
Implementing Data Lifecycle Management
Key steps for implementing data lifecycle programs include:
- Defining data classes and assigning ownership – This enables policies tailored to data types.
- Creating data requirements for each lifecycle stage – This identifies necessary procedures and controls.
- Evaluating technologies and tools – Solutions should unify siloed data environments.
- Building metadata management capabilities – This is crucial for tracking data end-to-end.
- Establishing data quality standards – These quantify and monitor data health.
- Developing data retention schedules – These optimize storage costs and compliance.
- Piloting with high-value use cases – This demonstrates benefits and refines processes.
- Cultivating data steward roles – This distributed accountability model aids governance.
- Ongoing reviews and improvements – This continuous evolution keeps pace with data landscapes.
Expert Techniques for High-Impact Data Lifecycle Programs
Beyond the basics, mature programs leverage advanced techniques like:
- Using workflow automation across the lifecycle for efficiency.
- Building centralized data services and tools for reuse.
- Leveraging artificial intelligence for classification and quality monitoring.
- Developing multi-tiered storage strategies to balance cost and performance.
- Extensive use of master data management and data catalogs.
- Embedding data stewardship into cross-functional teams.
- Collaborative issue escalation and change control processes.
- Convergence of analytical sandbox environments for flexibility.
- Automating disposal of unused data replicas and versions.
- Monitoring adoption and success metrics to guide enhancements.
What are the 3 Main Goals of Data Lifecycle Mangement ?
Here are 3 main goals of data lifecycle management:
- Improve Data Quality: A key goal of DLM is to implement processes that enhance the quality of data as it flows through various phases. Data cleansing, validation, and integration helps resolve inconsistencies, errors, duplication, and missing information. Higher quality data equates to more accurate analytics and insights.
- Optimize Data Utility: DLM aims to maximize the usefulness and value extracted from data at each stage of processing. Steps like data mining, predictive modeling, and metadata tagging augment data utility for different applications. Useful data can be leveraged to drive innovation and competitive advantage.
- Manage Costs: An effective DLM strategy reduces costs associated with unreliable, redundant, and unused data. Establishing retention policies, archival procedures, and optimizing storage helps minimize expenses. DLM provides visibility into most relevant datasets to focus budgets on high-value data. DLM goals revolve around enhancing data quality, utility, and cost-efficiency throughout the data lifecycle. Implementing DLM best practices helps ensure data is always fit-for-purpose at the point of use. Organizations can leverage quality information, extract maximal value from data assets, and manage expenses – achieving these three objectives is at the core of DLM programs.
Conclusion
In today’s exponentially expanding data landscapes, organizations can no longer allow data to proliferate recklessly as an uncontrolled liability. Instead, data must be strategically governed as an asset through comprehensive lifecycle management. By implementing centralized policies, integrated technologies, and distributed data stewardship, companies can gain control over their data from inception through retirement.
FAQ’s on Data Lifecycle Management
1. What are some leading data lifecycle management software solutions?
Top solutions include InformaticaAxon, Oracle DI suite, IBM InfoSphere Optim, ASG Technologies, and Innovative Routines International. Diverse products focus on different capabilities.
2. How is data lifecycle management different than data governance?
Data governance defines overall policy and strategy. Data lifecycle management executes tactical plans across the data pipelines to fulfill the governance directives. The two work hand-in-hand.
3. Can data lifecycle management help manage compliance with regulations?
Yes, data lifecycle retention rules, access controls, enhanced metadata, and better disposal align well with many compliance requirements. But additional controls may still be needed.
Share your thoughts in the comments
Please Login to comment...