Risk management identifies, assesses, and controls threats that severely impact an organization. The purpose is to minimize the destructive outcomes of those risks. Data technology is increasingly crucial in revolutionizing chance management throughout numerous industries. Data technology uses statistical fashions, predictive analytics, device-gaining knowledge of algorithms, and other advanced analytical techniques to derive actionable insights from records. Organizations can leverage those statistics-pushed insights to quantify risks, expect effects, and make more knowledgeable selections about threat mitigation techniques.
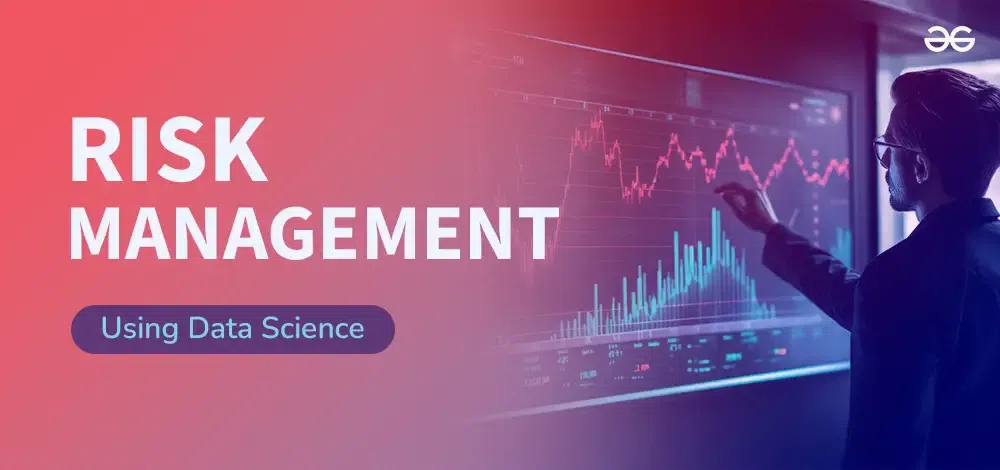
Risk Mangement used in Data Science
Data science in risk management is like having a crystal ball powered by math! It crunches numbers to predict future mishaps, spots sneaky fraudsters hiding in data shadows, and even plays matchmaker, pairing people with their credit scores. It’s the superhero cape that companies wear to dodge financial kryptonite!
In this article, we will explore How Data Science is used in Risk Management, the Application of Data Science and Balancing Quantitative Insights with Qualitative Considerations in Risk Mitigation Strategies.
What is Data Science?
Data science is the smart use of cutting-edge analytics to mine massive amounts of data from various sources for insightful information. In Data Science the methodical approach to interpreting unprocessed data and applying various classifications to predict and prevent future events. Consider data science to be a five-step scientific process. Data is initially gathered from various sources. Following that, it is stored and staged with extreme care to maintain its quality. The data is then categorized and summarized.
How does Risk Management Work?
In the business world, challenges are part and parcel – be it natural disasters, financial uncertainties, accidents, or issues affecting productivity and reputation. That’s where risk management steps in.
Risk management using Data Science is about making practical decisions to minimize the impact of potential risks on a business. By investing in tools and strategies that reduce risks, businesses can protect themselves from unexpected financial losses, uncertainties, and operational hiccups. The process begins by spotting potential threats and then exploring alternatives to lessen their impact. After putting solutions into action, the results are measured and analyzed. This straightforward approach helps businesses stay ahead of challenges, ensuring their operations can weather unforeseen setbacks.
The Role of Data in Risk Management
Data is crucial in risk management. It helps firms detect and address potential problems early. By analyzing past occurrences and trends, organizations can prioritize their responses. Machine learning and other advanced techniques assist in spotting future risks and improving transparency in decision-making. By using data effectively, firms can proactively manage threats, ensuring smooth operations even in unexpected conditions.
A risk data scientist is crucial for businesses dealing with uncertainty. They collect and analyze data to spot patterns showing risks. They predict future risks and help create strategies to avoid them. Working closely with managers and analysts, they turn insights into action to keep the company safe in a complex world.
How to Use Data Analytics for Risk Management?
Using data analytics for risk management involves several steps. First, gather relevant data from various sources. Analyze this data to detect patterns and anomalies indicating potential risks. Prioritize response strategies based on severity and probability. Utilize predictive analytics for forecasting future risks. Data visualization can help to better convey risk findings. Regularly monitor and update risk profiles. Collaborate with data analysts, risk managers, and stakeholders. Organizations may use data analytics to better predict, mitigate, and respond to hazards, therefore protecting operations and assets.
Is Data Science at Risk?
Data science isn’t in danger; it’s evolving and getting better. Challenges like data privacy and ethics give us chances to improve. New technology and methods make data science stronger. Lots of industries need data-driven insights, so data scientists are still important. They help companies make decisions, innovate, and stay competitive. We keep learning to handle new challenges. Data science is essential for progress and innovation in many areas. It’s not going away anytime soon.
Application of Data Science to Reduce Risk
Like some of the most prominent data analytics use cases in risk management include:
1. Risk Identification
The first step in dealing with risk is identifying capability threats, activities, uncertainties, or untapped opportunities that might impact a corporation’s key targets and performance. Data science is pivotal in increasing the identity of rising and hastily evolving risks. Predictive analytics techniques are used to analyze inner and external facts to come across anomalies and styles that factor into dangers that have not now manifested.
For example, banks use customer transaction data to pick out sports that could indicate fraud, cash laundering, or different monetary crimes before they arise. Historical statistics on risks and losses are also analyzed through the usage of information mining and statistical fashions to find particular events’ leading signs and hazard factors. Another vital utility is using devices gaining knowledge of algorithms to robotically scan news articles, social media conversations, industry reports, and different text data resources to perceive mentions of troubles that could characterize rising risks. This permits corporations to avoid risks before they make headlines and feature an outsized effect.
2. Risk Measurement
Once critical risks have been identified, quantitative risk measurement is essential for diagnostic analysis, prioritization of resources, setting tolerance thresholds, financial reporting, capital planning, and other core management functions. Data science is advancing risk measurement to be more accurate, consistent, and efficient. Based on available data, a wide range of statistical techniques are used to estimate the likelihood and potential impact of identified risks. Standard measures include Value at Risk (VaR), Expected Shortfall (ES), Loss Given Default (LGD) and more tailored metrics. Advanced analytics translate disparate data into quantitative risk parameters that feed into these models.
For example, banks build credit risk models that predict the probability of default and loss given default across various segments of their lending portfolio based on customer attributes, macroeconomic variables, financial transaction histories, and other data points. Models are backtested on historical data and then used to measure credit risk exposure. Machine learning classification algorithms are also applied to tagged internal loss data to build predictive models that can categorize incidents by risk category, quantify incident severity, and identify the biggest loss drivers. These data-driven risk measurement models enhance consistency, reduce subjectivity, and improve governance compared to qualitative or survey-based scoring methods.
3. Risk Monitoring
Ongoing monitoring of identified risks enables organizations to track shifts in exposure or early warning signs of emerging issues. Data science is powering more automated, real-time tracking of risks across more comprehensive datasets.
Dashboards populated by regularly updated data feeds allow risks to be monitored at times. Data visualization also converts risk metrics into more easily digestible formats to support risk governance. Exception-based alerts that trigger when early warning indicators or tolerance thresholds are breached enable timely escalations. Natural language processing and text analytics applied to news articles, regulatory filings, customer complaint escalations, and other text corpora also facilitate real-time monitoring of external signals that could signify changes in risk exposure. Tools can be configured to notify users automatically when specific keywords spike above historical levels. For financial institutions, transaction monitoring systems powered by machine learning provide continuous visibility into money laundering, fraud and other financial crime risks—ongoing screening, pattern detection, tracking suspicious behaviour, and investigation management support early detection and prevention.
Advantages afforded by tech-enabled monitoring include earlier response to address emerging issues before they escalate and productivity gains from automating manual tracking and reporting. However, the volume of data alerts may be overwhelming if not properly managed. There is also potential for false positives to distract from legitimate warnings. Users need training to interpret alerts and clearly defined protocols for escalation.
4. Risk Modeling
Data science supports more robust quantification of complex risks through enhanced modelling capabilities. Organizations can recreate an extensive range of operating scenarios using simulation techniques based on statistically reasonable assumptions. Modelling out the entire distribution of potential outcomes facilitates deeper analysis into tail risks and stress conditions.
For example, financial institutions use historical data to simulate GDP growth, unemployment figures, interest rate yield curve shifts, and other macroeconomic conditions under various recession scenarios. Based on stress test models built on this simulated economic environment, lenders then forecast credit losses and capital implications. This modelling technique meets regulatory requirements for stress testing. In addition, sophisticated catastrophe models for the insurance industry recreate potential natural disaster events using engineering and geoscience data combined with actuarial science. Running thousands of simulations helps insurers estimate potential claims and losses at specified return periods for floods, hurricanes, earthquakes, and other catastrophic perils. This informs underwriting, pricing decisions and reinsurance structures..
5. Risk Forecasting
While modelling focuses on hypothetical scenarios, risk forecasting leverages data to predict actual outcomes within a future period. A probabilistic view of potential losses or risk events in the near term allows organizations to initiate mitigation actions earlier and more effectively. Predictive analytics techniques like regression analysis identify leading indicators and critical risk drivers from historical data and then correlate these factors to actual risk outcomes. Once models are developed on past data, organizations can input the latest values of risk indicators to generate loss forecasts.
For instance, supply chain risk models could incorporate supplier performance data, shipment delivery logs, global events impacting production or transportation, and other variables to predict the likelihood of supply disruptions over a specified time horizon. Weather analytics also follow a similar approach to forecast extreme events that could impact operations.
6. Risk Reporting
Timely, consistent and accurate reporting provides risk transparency for senior management, the Board of Directors and external regulators. This necessitates aggregating quantitative metrics and qualitative assessments across disparate systems into user-friendly standardized formats. Data science facilitates more automated, integrated risk reporting. Financial, operational and compliance data can be harmonized using data integration platforms to calculate aggregated risk metrics across product lines, business units, legal entities and risk categories. Dashboards automatically populate with the latest underlying data flows to provide real-time visibility—natural language generation algorithms also auto-populate report templates with custom narratives. Capgemini says these techniques can reduce the risk reporting process timeline by 80% while improving quality and auditability. Risk teams can focus on robust analytics rather than manual reporting tasks. However, using black box algorithms for such sensitive use cases also raises accountability challenges which need governance.
7. Risk Mitigation
The end goal of risk management is to bring exposure levels within acceptable limits through targeted mitigation actions. Data science strengthens risk mitigation planning in several ways. Predictive models help risk managers understand how hypothetical strategies could impact future losses under various scenarios. Optimization algorithms also identify the most compelling portfolio of mitigations across constraints like cost, resource requirements and feasibility. In addition, analyzing past incidents helps quantify the historical success of specific mitigation programs in reducing losses. This empowers organizations to double down on the most impactful strategies. Sentiment analysis and natural language processing of textual data from news and social media also keep risk managers abreast of the latest developments that may warrant adjustments to mitigation tactics. However, when backtesting models on historical data to evaluate mitigation performance, organizations need caution not to be overly optimistic about strategy effectiveness. Unpredictable future events may render tactics less valid.
The Risk Management Team of the Future
As data science integrates further into risk management, the evolution of skill sets and structures in risk teams becomes imperative. The demand rises for expertise in both data science within risk functions and risk domain knowledge within data teams. Organizations address this by establishing dedicated analytics groups in existing risk departments or opting for centralized data science Centers of Excellence. These embedded data scientists collaborate closely with risk analysts or provide cross-functional analytics support to risk management groups. Regardless of the structure, fostering collaboration between data experts and risk experts is crucial. Multi-disciplinary teams working in agile sprints on specific use cases combine complementary skill sets. Training programs bridge knowledge gaps between technical and non-technical practitioners. Risk managers develop data literacy, while data scientists immerse themselves in risk concepts early in development sprints.
Balancing Quantitative Insights with Qualitative Considerations in Risk Mitigation Strategies
While data provides quantifiable estimates of mitigation impacts, organizations must weigh quantitative factors with qualitative considerations around ethics, values, corporate priorities and other human factors when deciding on the appropriate strategies. An overemphasis on data insights for decision-making without checks and balances from governance teams assessing qualitative impacts can skew risk mitigation tactics in the wrong direction.
For instance, an algorithm might identify customer profiling based on sensitive attributes like race, gender and income level as an optimized way to reduce credit risk. However, such an approach raises ethical concerns and reputational hazards, which could significantly outweigh any potential financial gains. An adaptive, balanced approach must be taken to develop mitigation tactics that apply human judgment to interpret data signals against business priorities, risk appetite constraints, and ethical considerations. As strategies are implemented, their practical impact should be monitored to feed enhanced insights into models in a virtuous cycle. This underscores why data science can only partially automate risk management alone. Human collaboration between data professionals and risk experts is crucial to realizing benefits while proactively addressing limitations.
Conclusion
Adopting data science disrupts risk management across identification, measurement, monitoring, modelling, forecasting, reporting and mitigating threats and uncertainties. Advanced analytics translates data into actionable risk intelligence so organizations can quantify exposures, predict losses, spot issues earlier, model hypothetical scenarios, forecast probabilistic outcomes, automate reporting for transparency, and plan mitigation strategies leveraging machine learning optimization.
These data-driven capabilities unlock immense potential value, including better-informed decisions, earlier interventions, productivity efficiencies, and improved risk governance. However, practitioners must maintain realistic expectations regarding the reliability of data science models and not use algorithms as a black box or replacement for human expertise.
Share your thoughts in the comments
Please Login to comment...