Top Challenges for Artificial Intelligence in 2024
Last Updated :
29 Apr, 2024
In 2024, Artificial Intelligence (AI) is leading the way in innovation, bringing big changes to different fields. But with all the exciting possibilities, AI also faces some tough problems.
In this article, we’ll explore the top challenges facing AI in 2024. From technical complexities to ethical considerations, we’ll delve into the hurdles that AI must overcome to realize its full potential.
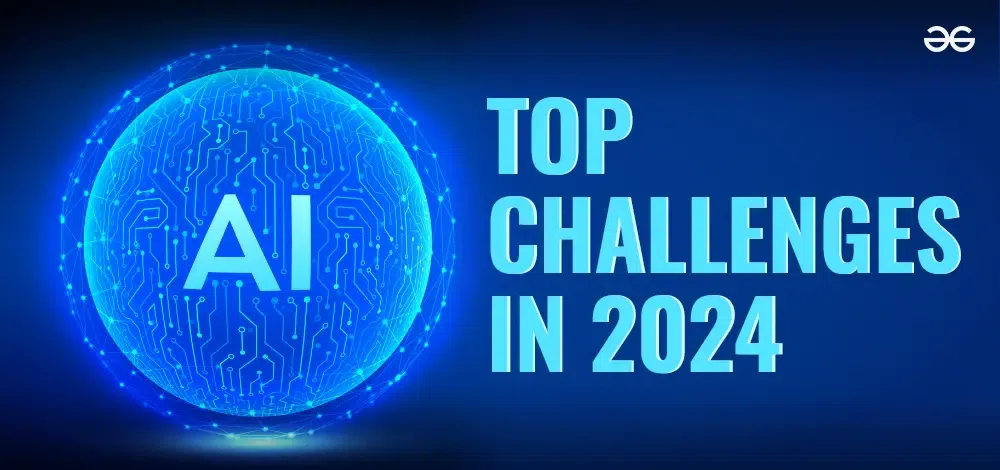
Top Challenges For AI in 2024
Most of these challenges for Artificial Intelligence faced by companies can be handled if they are keen to move ahead into the AI market. And so, this article details some of these challenges and how companies can overcome them to implement AI in their work culture. So let’s see these challenges now!
Top Challenges for Artificial Intelligence
1. Limited Knowledge
Artificial Intelligence may be a trendy topic in tech circles but there are very few people who understand what it is. it can be the top challenge in Artificial intelligence. Many myths are floating about Artificial Intelligence such as that only big companies like Google, Facebook, etc. have AI capabilities or even that AI can become smarter than humans and end the world! This lack of knowledge about how Artificial Intelligence is practically implemented in the day-to-day operations of companies means that it is very difficult for smaller and mid-level companies to use it successfully.
Another factor that leads to limited knowledge of AI is that there are very few AI experts that can apply AI solutions to real-life business problems. Most of the smaller companies struggle to find good AI talent that can form their in-house AI team. However, a solution to this is that these companies can develop their Artificial Intelligence and Data Science teams.
2. Black Box Problem
Another Challenge in Artificial Intelligence is Artificial Intelligence algorithms are like black boxes, which means humans know what the prediction generated by AI is, but they are unaware of how it is produced or how AI generates this kind of prediction. This means that people have no means of understanding the inner workings of AI algorithms. This makes them slightly unreliable. If the predictions generated are the same as those anticipated by the AI professionals then that’s great, but what if they are not? There is no way to understand how AI algorithms arrive at their predictions.
An approach that aims to solve this problem challenge in Artificial intelligence is Local interpretable model-agnostic explanations or LIME. This means that the AI algorithm will also provide the pieces of data that led to its eventual prediction. So if humans are provided the reason behind how an algorithm made a particular prediction, it eliminates the black box problem and also makes the algorithm more trustworthy in general.
3. High Computing Power
Artificial Intelligence is becoming more and more popular but it takes up a lot of computing power to train the AI. It can be a good challenge in Artificial Intelligence As deep learning algorithms become more and more complex, it becomes even more difficult to arrange the number of cores and GPUs they require to work efficiently. This is the reason that Artificial Intelligence is still underused in some fields like asteroid tracking, and healthcare deployment, even though it could contribute a lot of value. The integration of Cloud Computing and parallel processing systems have made it easier to work with artificial intelligence but it is still a power that few can afford to utilize fully.
4. Artificial Intelligence Bias
Artificial Intelligence Bias is also a challenge in artificial intelligence companies. AI bias means that sometimes AI systems can make unfair decisions or treat people unfairly.
This happens because the AI might be using wrong or unfair information to make decisions, or its rules might not be fair. For example, if an AI system is taught using data that has biases or unfairness in it, it might end up making decisions that are also biased or unfair. It is a challenge for artificial intelligence as the bias may also creep into the systems because of the wrong data that is generated by human beings.
5. Data Inconsistency
Artificial Intelligence algorithms learn from the data already available. Therefore, the better the data they are provided, the better the final algorithm will be. However, it eventually became a challenge in artificial intelligence as this requires a lot of data that may sometimes not even be available.
The only method to resolve this is to understand the available data and the data that is missing. When the AI experts know the data that is missing, they may be able to obtain it if it is data that is publicly available or even buy it from third-party data vendors. However, some data is difficult or even illegal to obtain. In that case, some AI algorithms use synthetic data which is artificially created from scratch while simulating the real data. This method using synthetic data is a good option when there is data scarcity and there is not enough data available to train the AI model.
6. Situation-specific Learning
Artificial Intelligence algorithms can be trained for a particular situation but they cannot transfer their learning from one situation to another. It ends up becoming a challenge for artificial intelligence. For example, humans can use their experiences in a situation to help them in other situations as well. However, this is not possible for AI algorithms as they are trained only on data for one specified task. However, This can be done using transfer learning in which an AI model is trained on data in a particular situation but it can transfer its learning to another similar situation without starting from scratch. This means that an AI model developed for a particular task can then be used as a starting point for another AI model for a related task.
Conclusion
The most important thing to remember is that these challenges cannot just be handled in a short time. So companies must familiarise themselves with Artificial Intelligence and understand the process of creating AI solutions. Then they must create an AI strategy for its implementation into their work culture. After the strategy is created, it is much easier to just follow it and handle the challenges as they arise.
Top Challenges for Artificial Intelligence in 2024- FAQs
What are the main technical challenges facing artificial intelligence in 2024?
Technical challenges include developing AI algorithms capable of handling complex tasks, improving the scalability and efficiency of AI systems, and ensuring interoperability and compatibility across different AI platforms.
How are ethical considerations impacting the development and deployment of AI?
Ethical considerations are increasingly important in AI development, with concerns about bias, fairness, transparency, and accountability. Addressing these issues is crucial to building trust in AI systems and ensuring they benefit society as a whole.
What are the potential socio-economic implications of AI advancements?
AI advancements have the potential to disrupt industries, transform job markets, and exacerbate inequalities. Understanding and mitigating these socio-economic implications are essential for fostering inclusive growth and sustainable development.
How can we ensure fairness and transparency in AI algorithms?
Ensuring fairness and transparency in AI algorithms involves designing algorithms that are unbiased, accountable, and transparent in their decision-making processes. This requires robust data collection, rigorous testing, and ongoing monitoring of AI systems.
What regulatory frameworks are in place to govern the use of AI?
Regulatory frameworks governing the use of AI vary by region and jurisdiction. In 2024, governments and international organizations are increasingly focusing on developing and implementing regulations to address ethical, legal, and societal concerns related to AI.
How can individuals and organizations contribute to responsible AI development?
Individuals and organizations can contribute to responsible AI development by promoting ethical AI practices, advocating for transparency and accountability, and actively participating in discussions and initiatives aimed at addressing the challenges and opportunities of AI
Share your thoughts in the comments
Please Login to comment...