Reinforcement learning from Human Feedback
Last Updated :
17 Feb, 2024
Reinforcement Learning from Human Feedback (RLHF) is a method in machine learning where human input is utilized to enhance the training of an artificial intelligence (AI) agent. Let’s step into the fascinating world of artificial intelligence, where Reinforcement Learning from Human Feedback (RLHF) is taking center stage, forming a powerful connection between machine smarts and human know-how.
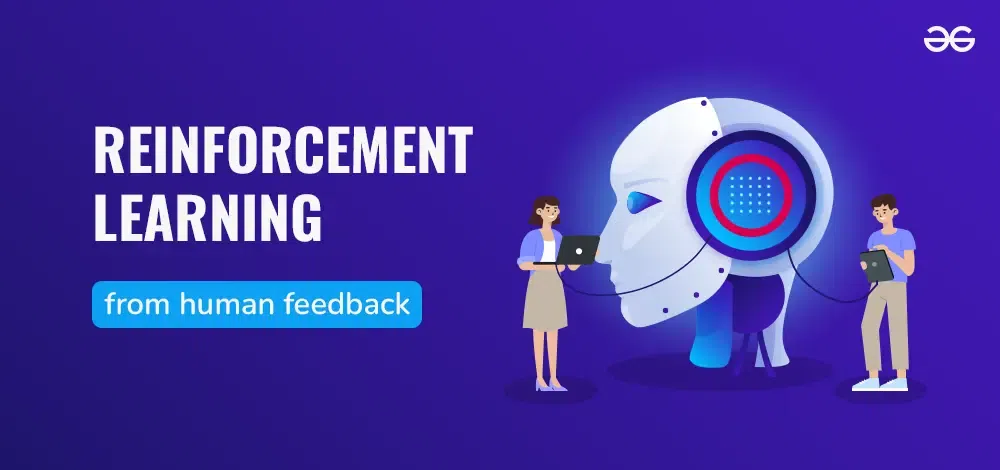
Imagine this approach as the brainchild that not only shakes up how machines grasp information but also taps into the goldmine of insights from us, the human experts. Picture algorithms navigating intricate decision realms, learning and growing through the wisdom of human feedback. It’s like the perfect dance between artificial intelligence and our collective experience, paving the way for a new era of intelligent systems. So, buckle up as we are going to explore the all whereabouts of RLHF in this article.
What is Reinforcement learning from Human Feedback?
In the realm of Artificial Intelligence, Reinforcement Learning from Human Feedback emerges as a game-changer, reshaping the landscape of how machines comprehend and evolve. In the intricate relationship between algorithms and human evaluators, RLHF takes center stage by fusing the computational might of Machine Learning with the nuanced insights brought by human experience. Unlike the traditional reinforcement learning script, where machines follow predetermined reward signals, RLHF introduces a dynamic feedback loop, enlisting humans as guides in the algorithmic decision-making process.
Let’s assume this: humans, armed with their expertise, provide real-time feedback on the system’s actions, creating a dynamic interplay that propels machines to navigate complex decision spaces with unprecedented intuition and adaptability. This symbiotic relationship isn’t just a tweak to existing models; it’s a revolutionary shift that harnesses the collective intelligence of human evaluators, fine-tuning algorithms to create not just efficient but context-aware systems. RLHF, with its innovative approach, doesn’t merely stop at enhancing Machine Learning models; it unfolds new horizons, paving the way for intelligent systems seamlessly woven into the tapestry of human experience.
RLHF in Autonomous Driving Systems
The autonomous driving systems learns from human drivers’ actions and feedback to improve its driving behavior. For instance, if the autonomous vehicle performs a maneuver that makes the human driver uncomfortable or seems unsafe, the driver can provide feedback through various means, such as pressing a button indicating discomfort or giving verbal feedback.
The reinforcement learning algorithm then analyzes this feedback to adjust the vehicle’s driving policies. Over time, the system learns to emulate safer and more comfortable driving behaviors based on the aggregated feedback from multiple human drivers. This iterative process allows autonomous driving systems to continuously improve and adapt to the preferences and safety concerns of human users.
How RLHF works?
RLHF works in three stages which are discussed below:
Initial Learning Phase:
In this foundational stage, the AI system embarks on its learning journey through traditional reinforcement learning methods. The machine engages with its environment by selecting a pre-trained model, fine-tuning its behavior based on predefined reward signals. This phase sets the groundwork for the system to grasp the basics and navigate its initial understanding of the task at hand.
Human Feedback Integration:
The second stage injects a powerful human element into the learning process. Enter the human evaluators – experts who provide insightful feedback on the machine’s actions and evaluate model output on accuracy or custom metrics. This human perspective introduces a layer of complexity and nuance beyond rigid reward structures, enriching the AI’s understanding. The amalgamation of machine and human insights is pivotal in crafting a more holistic and context-aware learning experience.
Reinforcement Learning Refinement:
Armed with the valuable feedback from human evaluators, the AI system enters the third stage of more intrinsic fine-tuning. Here, it undergoes further training, incorporating the refined reward model derived from human feedback. This iterative process of interaction, evaluation, and adaptation forms a continuous loop, progressively enhancing the machine’s decision-making capabilities. The result is an intelligent system that not only learns efficiently but also aligns with human values and preferences, marking a significant stride toward creating AI that resonates with human-compatible intelligence.
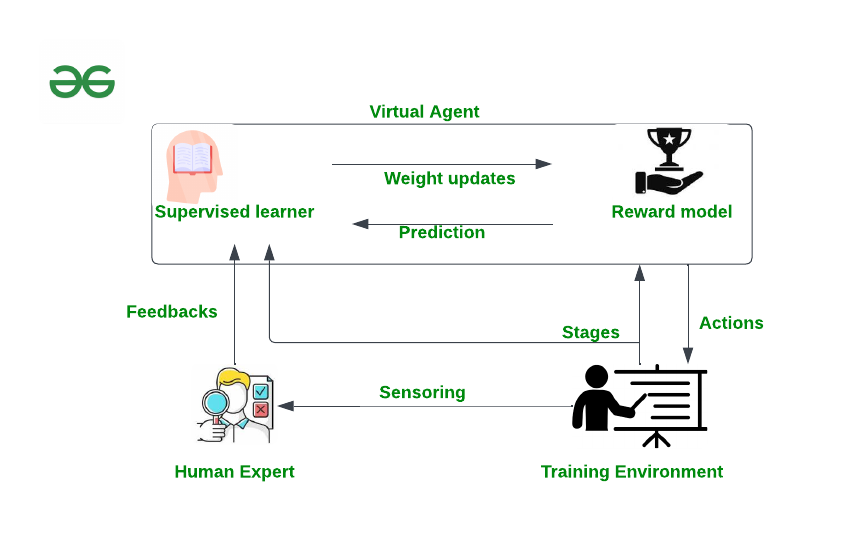
Workflow architecture of RLHF
Applications of RLHF
In recent days RLHF is being used in various important applications which are discussed below:
- Enhancing Language Model Training: Reinforcement Learning from Human Feedback finds a compelling application in the realm of language models, notably exemplified by models like GPT (Generative Pre-trained Transformer). In the initial phase, these models undergo pre-training on vast datasets, acquiring a broad understanding of language patterns. The incorporation of RLHF introduces a transformative second stage. Human evaluators, armed with linguistic expertise, provide nuanced feedback on the model’s generated text. This feedback, whether on fluency, coherence, or relevance, refines the language model’s understanding. Through iterative reinforcement learning refinement, the model adapts and aligns its outputs more closely with human expectations, resulting in an advanced language model capable of generating contextually rich and coherent text.
- Elevating ChatGPT Conversations: ChatGPT, our modern go-to conversational agent, getting even more savvy with every interaction. In the first phase, it immerses itself in a treasure trove of conversational data to grasp the subtleties of language. Then comes RLHF, taking the spotlight in the second stage. Human evaluators step up, providing insights into the appropriateness and relevance of it’s responses. This continuous loop of interaction, evaluation, and adaptation works wonders, refining it’s conversational prowess. The end result? A ChatGPT that effortlessly crafts contextually relevant and coherent responses, transforming it into a valuable asset for Natural Language understanding and communication.
- Adapting AI Systems with Human Intuition or GenAI: RLHF isn’t only confined to language models; it’s a game-changer for the broader realm of Artificial Intelligence. Take its contextual adaptation, for example – it’s like infusing human intuition into the very core of the learning process. This adaptability extends beyond language, influencing applications like customer support chatbots, virtual assistants, and automated content creation. By harnessing RLHF, these AI systems evolve from rule-bound responders to dynamic entities, understanding and adapting to diverse user inputs with an unprecedented level of nuance and sophistication. Essentially, RLHF acts as the driving force behind enhancing the performance and adaptability of language models like ChatGPT or any LLM, across a spectrum of real-world applications.
Advantages
Some of its major advantages are discussed below:
- Enhanced Adaptability: RLHF equips AI systems with remarkable adaptability, allowing them to navigate nuanced and ever-changing environments. The iterative feedback loop facilitates real-time learning which effectively ensures continuous improvement to the system.
- Human-Centric Learning: By integrating human evaluators into the learning process, RLHF captures the richness of human intuition and expertise.
- Context-Aware Decision Making: RLHF takes decision-making to a whole new level by allowing AI models to grasp the context of diverse situations which enhances their capability to respond appropriately. This proves especially valuable in applications like natural language processing and conversational agents where nuanced understanding is crucial.
- Improved Generalization: Another notable advantage lies in the improved generalization of AI models. Thanks to the integration of human feedback, these models become more adaptable across various scenarios, making them versatile and proficient in handling a wide range of tasks.
Disadvantages
Some of its limitations are discussed below:
- Bias Amplification: RLHF may inadvertently amplify biases present in human feedback. If evaluators introduce subjective biases, the system can perpetuate and even exacerbate these biases during the learning process.
- Limited Expertise Availability: The effectiveness of RLHF heavily relies on the availability of human evaluators with relevant expertise. Limited access to domain experts can hinder the quality and diversity of feedback which directs impacts the system’s learning process.
- Complex Implementation: Implementing RLHF can be intricate and resource-intensive. Integrating human feedback seamlessly into the learning loop requires careful design and managing the iterative process demands significant computational resources.
- Slow Learning Process and High Computational Costs: The iterative nature of RLHF involves human evaluation and model refinement which can slow down the learning process and increases computational costs. In scenarios where rapid adaptation is crucial, this method may not be as efficient as other, faster and cheaper learning approaches.
- Limited Generalization in Unseen Scenarios: While RLHF enhances generalization to some extent, AI models may struggle in entirely unforeseen scenarios. The reliance on human feedback may limit adaptability when faced with novel situations that were not covered during the training phase.
Future trend
Looking ahead, the future of Reinforcement Learning from Human Feedback (RLHF) looks promising with ongoing advancements in artificial intelligence. We can expect a focus on refining algorithms to tackle biases, improving the scalability of RLHF for broader applications, and exploring integrations with emerging technologies like augmented reality and natural language interfaces. However, an easier, faster and cheaper alternative method of RLHF is already proposed in 2023 which is Direct Preference Optimization. This alterative way can replace RLHF effectively as this new approach uses reward function of human preferences by skipping one costly steps of reward model training of RLHF.
Share your thoughts in the comments
Please Login to comment...