Machine learning tools have turned out to be integral assets for recording technological know-how professionals, facilitating the extraction of precious insights, and informing facts-driven decision-making. Machine learning tools are like helpful buddies for tech experts, helping them understand data and make smart decisions.
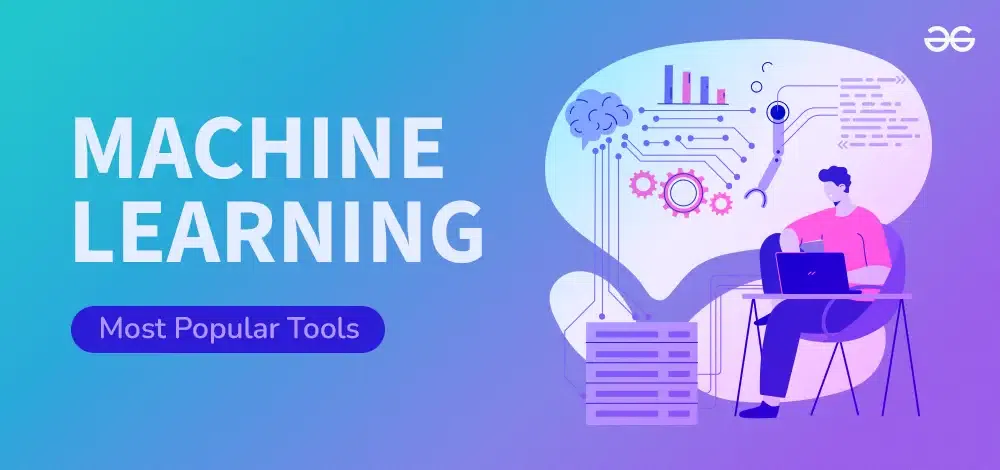
Machine learning Tools
In this article, we break down the Top 10 tools in 2024, making it super easy for you to choose the perfect one. We talk about it’s features pros, and cons, giving you all the info you need. This guide is like your friendly guidebook, telling you everything about each tool so you can pick the one that fits your needs.
Here are the listed 10 best machine learning tools with their features, pricing, etc These tools are widely used in industry and academia for various machine learning tasks, ranging from data preprocessing and feature engineering to model training and deployment.
TensorFlow
TensorFlow, an open-source machine learning tool, is renowned for its flexibility, ideal for crafting diverse models, and simple to use. With abundant resources and user-friendly interfaces, it simplifies data comprehension.
.webp)
Features of Tensorflow
- Strong and flexible open-supply machine learning library.
- Supports diverse applications for all types of tools.
- Bendy structure, scalability, and comprehensive documentation.
Pros:
- Lots of publications and help
- Good for one-of-a-kind styles of fashion
Cons:
- Can be a piece difficult for novices
- You want to realize some programming
Visit Site: https://www.tensorflow.org
PyTorch
PyTorch is a user-friendly machine learning tool, facilitating seamless model construction. Loved by researchers for its simplicity, it fosters easy idea testing and error identification. Its intuitive design makes it a preferred choice, offering a smooth and precise experience in model development.
.webp)
Features of Pytorch
- Popular for its simplicity and flexibility.
- User-friendly interface and great computation.
- Especially favoured amongst researchers for its ease of use.
Pros:
- Easy to try out ideas
- Simple to use
Cons:
- Not as good for big projects
- Smaller community than some other tools
Visit Site: https://pytorch.org/
Scikit-learn
Scikit-learn is a valuable tool for everyday machine-learning tasks, offering a plethora of tools for tasks like pattern recognition and prediction. Its user-friendly interface and extensive functionality make it accessible for various applications, whether you’re identifying patterns in data or making accurate predictions.
.webp)
Features of Scikit Learn
- Effective and easy-to-use machine learning library.
- The toolbox consists of algorithms for class, regression, clustering, and greater.
- Reliable machine for informative records.
Pros:
- Easy for everyday tasks
- Lots of tools for figuring out patterns
Cons:
- Not great for really complex tasks
- Doesn’t work as well for deep learning
Visit Site: https://scikit-learn.org/
Keras
Keras helps easily create models, great for quick experiments, especially with images or words. It’s user-friendly, making it simple to try out ideas, whether you’re working on recognizing images or understanding language.
.webp)
Features of Keras
- Famous for its consumer-friendly API.
- Simplifies the manner of constructing neural networks.
- High-level abstractions allow customers to focus on version structure.
Pros:
- Easy to build models
- Good for images and words
Cons:
- Not as good for very detailed projects
- You can’t change everything about the model
Visit Site: https://keras.io/
XGBoost
XGBoost excels in analyzing tabular data, showcasing exceptional prowess in pattern identification and prediction, making it a top choice for competitive scenarios. This machine learning tool is particularly adept at discerning trends and delivering accurate predictions, making it a standout performer, especially in competitive environments.
.webp)
Features of XGBoost
- Gradient boosting excels in coping with based/tabular statistics.
- High performance, scalability, and interpretability.
- Preference for accomplishing pinnacle-tier consequences in predictive modelling obligations.
Pros:
- Great at working with tables
- Finds patterns well
Cons:
- Not as good for other types of data
- Needs a lot of computer power for big tasks
Visit Site: https://xgboost.readthedocs.io/
Apache Spark MLlib
Apache Spark MLlib is a powerful tool designed for handling massive datasets, making it ideal for large-scale projects with extensive data. It simplifies complex data analysis tasks by providing a robust machine-learning framework. Whether you’re dealing with substantial amounts of information, Spark MLlib offers scalability and efficiency, making it a valuable resource for projects requiring the processing of extensive data sets.
.webp)
Features of Apache Spark MLlib
- Specialises in allotted system studying.
- Scalability, versatility, and seamless integration with other Spark components.
- Huge-scale data processing supports numerous machine-learning algorithms.
Pros:
- Good for lots of data
- Works well with other Spark tools
Cons:
- A bit hard for beginners
- Needs a big computer to work best
Visit Site: https://spark.apache.org/mllib/
Microsoft Azure Machine Learning
Microsoft Azure Machine Learning makes it easy to do machine learning in the cloud. It’s simple, user-friendly, and works well for many different projects, making machine learning accessible and efficient in the cloud.
.webp)
Features of Microsoft Azure:
- Cloud-primarily based platform imparting quit-to-cease machine learning solutions.
- Seamless integration with other Azure services.
- Novices and professionals, imparting scalable solutions for machines.
Pros:
- Easy to use in the cloud
- Good for many different projects
Cons:
- Costs money for big projects
- You need to learn a bit about the cloud
Pricing: Costs money based on use
Visit Site: https://azure.microsoft.com/en-us/services/machine-learning/
Google Cloud AI Platform is a strong tool for using machine learning on Google Cloud. Great for big projects, it easily works with other Google tools. It provides detailed stats and simple functions, making it a powerful option for large machine-learning tasks.
.webp)
Features of Google Cloud AI Platform:
- A comprehensive set of gear for machine learning obligations.
- Scalable infrastructure for large-scale machine learning.
- Extensive spectrum of users, from personal researchers to big companies.
Pros:
- Good for big projects in the cloud
- Works well with other Google tools
Cons:
- Costs money based on the use
- You need to know a bit about the Google Cloud
Visit Site: https://cloud.google.com/ai-platform
H2O.ai
H2O.ai is a tool that helps you use machine learning easily. It’s good for many jobs and has a helpful community. With H2O.ai, you can use machine learning well, thanks to its easy interface and helpful people.
.webp)
Features of H2O.ai :
- Open-supply machine-learning platform with a focus on simplicity and pace.
- Consumer-friendly AutoML capability, assistance for numerous machine-learning algorithms.
- An invaluable tool for model development techniques.
Pros:
- Easy to use quickly
- Good for many tasks
Cons:
- Not as good for really detailed tasks
- Doesn’t have everything you might need
Visit Site: https://www.h2o.ai/
RapidMiner
RapidMiner is an all-rounder tool for the entire machine learning method, ideal for concept exploration and collaboration on tremendous projects. It enables trying out ideas and permits seamless teamwork, making it a versatile tool for diverse stages of machine learning development.
.webp)
Features of RapidMiner:
- Records technology platform that covers the complete machine learning lifecycle.
- User-friendly interface and complete machine-learning workflows.
- Designing, training, and deploying machine learning fashions made easy.
Pros:
- Good for all the steps of machine learning
- Easy to try out ideas
Cons:
- Some things might be a bit hard for beginners
- Costs money based on the use
Pricing: Costs money based on use
Visit Site: https://rapidminer.com/
Choosing the right tool depends on what you want to do. If you’re getting into deep machine learning, TensorFlow and PyTorch are good because they have lots of tools and support for complex networks. For everyday tasks, scikit-learn is easy to use and reliable. For big projects, cloud platforms like Azure and Google Cloud offer lots of resources and services. They help with handling large amounts of data and complex models. If you want something simple and quick, H2O.ai and RapidMiner are good choices. They have easy interfaces for people with different levels of knowledge. H2O.ai is great for machine learning and predictions, while RapidMiner has a visual way of working with data tasks. Remember to think about your skill level and what you need for your project. If you’re just starting, scikit-learn might be a good fit.
If you’re more experienced, TensorFlow and PyTorch offer more advanced options. Cloud platforms are useful for big computations and storage needs. H2O.ai and RapidMiner are good for quick and easy solutions in machine learning and data tasks.
Conclusion
In the big world of machine learning, choosing the right tool depends on what you need to do. We’ve got 10 tools that are good for different jobs, like working with data, pictures, or words. If you’re dealing with lots of data or doing complicated things with neural networks, TensorFlow or PyTorch are good choices. They’re like toolboxes for heavy math stuff, especially for deep learning.
For everyday tasks where you want things to be easy, scikit-learn is there to help. It’s simple to use and works well for common machine-learning jobs. If you’re working on big projects, cloud platforms like Azure or Google Cloud can give you the power and space you need. They have lots of resources for machine learning, from training models to putting them into action.
What are the most popular machine learning tools in 2024?
These top 10 machine learning tools, like TensorFlow and PyTorch, are like superhero sidekicks for tech enthusiasts. They include scikit-learn for easy tasks, Keras for simple neural networks, and XGBoost for boosting model performance.
Tools like Apache Spark MLlib and Microsoft Azure Machine Learning bring big data capabilities. Google Cloud AI Platform offers a cloud-based playground, while H2O.ai and RapidMiner make complex tasks very easier.
What is TensorFlow, and why is it popular?
TensorFlow is sort of a wonderful smart calculator for machines. It allows them to learn things by processing masses of facts. It’s famous because it’s effective, flexible, and utilized by many large companies to construct cool system mastering models.
Are these tools easy for beginners?
Yes, tools like scikit-learn, Keras, and RapidMiner are easy for beginners to use. Scikit-learn is user-friendly, making everyday machine-learning tasks simple. Keras provides a high-level interface, making it easy for beginners to create neural networks. RapidMiner is intuitive and accessible for novice users, streamlining the machine learning process.
Which tool is best for big projects?
Tools like Azure, Google Cloud, H2O.ai, and RapidMiner are good for big projects with lots of data. These platforms offer not only ample computational resources but also efficient tools for managing and analyzing extensive datasets in large-scale machine-learning projects.
What is the role of Apache Spark MLlib in machine learning?
Apache Spark MLlib is like a speedy engine for big data. It helps machines learn from massive amounts of information quickly. It’s great for when you have a ton of data and need to teach machines fast.
Share your thoughts in the comments
Please Login to comment...