Market Basket Analysis in Data Mining
Last Updated :
15 Feb, 2023
A data mining technique that is used to uncover purchase patterns in any retail setting is known as Market Basket Analysis. In simple terms Basically, Market basket analysis in data mining is to analyze the combination of products which been bought together.
This is a technique that gives the careful study of purchases done by a customer in a supermarket. This concept identifies the pattern of frequent purchase items by customers. This analysis can help to promote deals, offers, sale by the companies, and data mining techniques helps to achieve this analysis task. Example:
- Data mining concepts are in use for Sales and marketing to provide better customer service, to improve cross-selling opportunities, to increase direct mail response rates.
- Customer Retention in the form of pattern identification and prediction of likely defections is possible by Data mining.
- Risk Assessment and Fraud area also use the data-mining concept for identifying inappropriate or unusual behavior etc.
Market basket analysis mainly works with the ASSOCIATION RULE {IF} -> {THEN}.
- IF means Antecedent: An antecedent is an item found within the data
- THEN means Consequent: A consequent is an item found in combination with the antecedent.
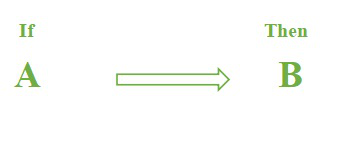
Let’s see ASSOCIATION RULE {IF} -> {THEN} rules used in Market Basket Analysis in Data Mining. For example, customers buying a domain means they definitely need extra plugins/extensions to make it easier for the users.
Like we said above Antecedent is the item sets that are available in data. By formulating from the rules means {if} component and from the example is the domain.
Same as Consequent is the item that is found with the combination of Antecedents. By formulating from the rules means {THEN} component and from the example is extra plugins/extensions.
With the help of these, we are able to predict customer behavioral patterns. From this, we are able to make certain combinations with offers that customers will probably buy those products. That will automatically increase the sales and revenue of the company.
With the help of the Apriori Algorithm, we can further classify and simplify the item sets which are frequently bought by the consumer.
There are three components in APRIORI ALGORITHM:
Now take an example, suppose 5000 transactions have been made through a popular eCommerce website. Now they want to calculate the support, confidence, and lift for the two products, let’s say pen and notebook for example out of 5000 transactions, 500 transactions for pen, 700 transactions for notebook, and 1000 transactions for both.
SUPPORT: It is been calculated with the number of transactions divided by the total number of transactions made,

support(pen) = transactions related to pen/total transactions
i.e support -> 500/5000=10 percent
CONFIDENCE: It is been calculated for whether the product sales are popular on individual sales or through combined sales. That is calculated with combined transactions/individual transactions.

Confidence = combine transactions/individual transactions
i.e confidence-> 1000/500=20 percent
LIFT: Lift is calculated for knowing the ratio for the sales.

Lift-> 20/10=2
When the Lift value is below 1 means the combination is not so frequently bought by consumers. But in this case, it shows that the probability of buying both the things together is high when compared to the transaction for the individual items sold.
With this, we come to an overall view of the Market Basket Analysis in Data Mining and how to calculate the sales for combination products.
Types of Market Basket Analysis
There are three types of Market Basket Analysis. They are as follow:
- Descriptive market basket analysis: This sort of analysis looks for patterns and connections in the data that exist between the components of a market basket. This kind of study is mostly used to understand consumer behavior, including what products are purchased in combination and what the most typical item combinations. Retailers can place products in their stores more profitably by understanding which products are frequently bought together with the aid of descriptive market basket analysis.
- Predictive Market Basket Analysis: Market basket analysis that predicts future purchases based on past purchasing patterns is known as predictive market basket analysis. Large volumes of data are analyzed using machine learning algorithms in this sort of analysis in order to create predictions about which products are most likely to be bought together in the future. Retailers may make data-driven decisions about which products to carry, how to price them, and how to optimize shop layouts with the use of predictive market basket research.
- Differential Market Basket Analysis: Differential market basket analysis analyses two sets of market basket data to identify variations between them. Comparing the behavior of various client segments or the behavior of customers over time is a common usage for this kind of study. Retailers can respond to shifting consumer behavior by modifying their marketing and sales tactics with the help of differential market basket analysis.
Benefits of Market Basket Analysis
- Enhanced Customer Understanding: Market basket research offers insights into customer behavior, including what products they buy together and which products they buy the most frequently. Retailers can use this information to better understand their customers and make informed decisions.
- Improved Inventory Management: By examining market basket data, retailers can determine which products are sluggish sellers and which ones are commonly bought together. Retailers can use this information to make well-informed choices about what products to stock and how to manage their inventory most effectively.
- Better Pricing Strategies: A better understanding of the connection between product prices and consumer behavior might help merchants develop better pricing strategies. Using this knowledge, pricing plans that boost sales and profitability can be created.
- Sales Growth: Market basket analysis can assist businesses in determining which products are most frequently bought together and where they should be positioned in the store to grow sales. Retailers may boost revenue and enhance customer shopping experiences by improving store layouts and product positioning.
Applications of Market Basket Analysis
- Retail: Market basket research is frequently used in the retail sector to examine consumer buying patterns and inform decisions about product placement, inventory management, and pricing tactics. Retailers can utilize market basket research to identify which items are sluggish sellers and which ones are commonly bought together, and then modify their inventory management strategy accordingly.
- E-commerce: Market basket analysis can help online merchants better understand the customer buying habits and make data-driven decisions about product recommendations and targeted advertising campaigns. The behaviour of visitors to a website can be examined using market basket analysis to pinpoint problem areas.
- Finance: Market basket analysis can be used to evaluate investor behaviour and forecast the types of investment items that investors will likely buy in the future. The performance of investment portfolios can be enhanced by using this information to create tailored investment strategies.
- Telecommunications: To evaluate consumer behaviour and make data-driven decisions about which goods and services to provide, the telecommunications business might employ market basket analysis. The usage of this data can enhance client happiness and the shopping experience.
- Manufacturing: To evaluate consumer behaviour and make data-driven decisions about which products to produce and which materials to employ in the production process, the manufacturing sector might use market basket analysis. Utilizing this knowledge will increase effectiveness and cut costs.
Share your thoughts in the comments
Please Login to comment...