What Is Spatial Analysis, and How Does It Work
Last Updated :
02 Apr, 2024
Have you ever wondered how city planners come up with decisions like what is the best location for a school, hospital, or park? How did during the COVID outbreak the spread of the virus was recorded and monitored to effectively alienate affected areas to decrease the spread? How vaccination strategies are made for various diseases and monitored to ensure an equal distribution. How are such accurate maps created? How does our GPS know all the high-traffic routes in the area and help find the shortest and fastest route?
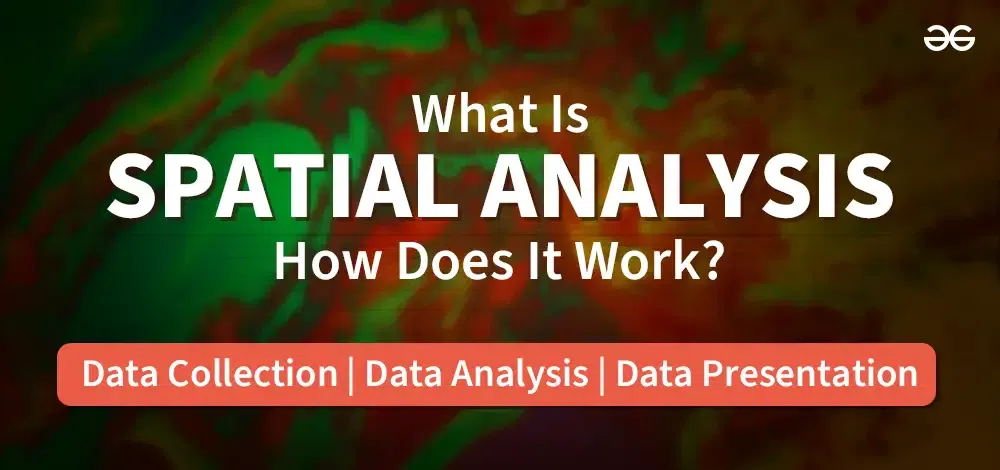
It can all be done using spatial data and spatial analysis. Spatial analysis involves understanding patterns and relationships based on spatial data. In this article, we will discuss what is spatial analysis and how it works.
What is Spatial Analysis?
The world is full of data. But this data is useless until we can gain some meaningful insights from it. Spatial analysis is the process of using analytical tools to analyze and represent data, relationships, and patterns among various geospatial data. This method converts data to information where we can get to know about various geographic features from the data collected by satellites, data on maps, etc. It uses a variety of analytical techniques, algorithms, and computational data to draw a relationship between data and use it for the target system like environment management, urban planning, etc.
What is Spatial Data?
Spatial data also called geospatial data contains information that has a geographic component. Spatial data is broadly classified into two categories, vector and raster. Let’s take a look at each one of them.
1. Vector Data
Vector data represents spatial features using points, lines, and polygons. In GIS, vector data is used to represent addresses and points of interest with points; rivers, railways, roads using lines and lakes, and buildings with polygons.
- Point – A point is depicted by a single dot on the layer. It is the simplest type of vector data and can be accessed using a single pair of coordinates i.e. x and y coordinates of that point. A point has zero dimension. Examples of points include the position of cities, landmarks, schools, etc. on a map.
- Line – Lines can be depicted as a sequence of connected points depicting the shape and location of a linear feature. A line is one-dimensional vector data. Examples of lines include rivers, roads, and power lines on a map.
- Polygon – A polygon is formed by connecting points in such a way that it forms a closed loop. It can also be seen as a line with the same start and end point, hence a closed loop. Each polygon can be differentiated from the others by assigning different colors to each polygon. Examples where polygons are used where we need to depict a defined area or boundary like buildings, closed water bodies, etc.
2. Raster Data
Raster data in contrast to vector data is a grid of cells where each cell represents a specific value. Examples of raster data include aerial photographs, imagery from satellites, digital pictures, and scanned maps. In raster data, each cell of the grid holds a single value representing various attributes like elevation, depth, etc.
- Digital Elevation Models(DEM) – This kind of raster data depicts the topography of the surface in terms of elevation or depth.
- Satellite Imagery – This kind of rater data depicts the aerial photographs taken by satellites where each cell in the grid takes up a color to imitate the image taken by the satellite.
- Temperature maps – This kind of raster data stores the temperature at each location in the cells of the grid.
Thus, rater data is used to store continuous data whereas vector data is used to store data with well-defined boundaries.
Apart from vector and raster data, there is another type of data called attribute data that usually comes along with spatial data. This data is used to add more information to the spatial data. For example, vector line data depicting a road might come along with attribute data defining the road name, the connecting cities, etc.
Importance of Spatial Analysis
Following are some of the reasons why spatial analysis is so important in today’s world.
- Decision-making – Since the spatial data used is collected from multiple sources, the data-driven insights provided can be used by decision-makers to choose an action based on the need. For example, geographic information about the outbreak of a specific disease can help the authorities make a decision as to which area has to be put under lockdown and also develop a vaccination strategy.
- Omnipresent Technology – Spatial Analysis is a very important technology used in today’s most used applications like food delivery applications like Zomato and Swiggy, transportation applications like Ola and Uber, and GPS systems like Google Maps where the geographic information is used to get the shortest route between two points.
- Recognizing Spatial Patterns – Using spatial analysis, you can visualize spatial data like population density, heat maps, and disease outbreaks that help develop patterns between various data. For example, population density and heat maps can be used to determine that some areas with high heat values are also areas with high population density thus creating a relationship between the two attributes.
How does Spatial Analysis Work?
Spatial analysis is the process of using analytical tools to analyze and represent data, relationships, and patterns among various geospatial data. This task of analyzing and recognizing patterns is discussed as follows.
1. Data Collection
The collection of data is the first step in spatial analysis. It uses varied ways to gather information from the sources. Data can be collected using remote sensing devices like LiDAR and airborne systems, field surveys by on-ground observations and measurements, open source datasets, etc.
Data acquired is then formatted and converted into a uniform format that can be used for further processing.
2. Data Analysis
This is the second step and is used to gain insightful information from the data collected and prepared. This can be done by employing various analysis models like the distance and proximity model that uses the distance between two points and proximity between two attributes to develop a relationship between data, spatial overlay where multiple data sets are layered together to recognize patterns, statistics which recognizes clusters that helps understand the distribution of the attributes. All this analysis can be easily done using AI and ML models where the models can be trained on multiple and varied data sets to increase accuracy.
3. Data Presentation
After the analysis of the data, it is important to present the results. This can be done in the form of graphs, charts, maps, tables, and various visualization tools. 2D can also get a better perspective using 3D visualization tools which add a variable to 2D data to make it 3D and hence more presentable.
Application of Spatial Analysis
1. Urban Development
- Create Resilient Urban Cities – Climate change has a great impact on urban life. Thus, policymakers are working on ways to minimize the effect of climate by analyzing deforestation patterns, sea level analysis due to increasing global warming, and emission analysis and strategy to shift to efficient energy resources.
- Monitor and Reduce Urban Heat Island (UHI) effect – The UHI effect is the phenomenon where natural vegetation is replaced with buildings. This leads to more heat retention. Spatial analysis techniques like thermal remote sensing, satellite imagery, and field observations can be used to collect relevant data and understand spatial patterns.
- Determine Quality of Life – Spatial data can be used to determine the socioeconomic quality of life. For example, areas with distributed hospital services have a better quality of life. Areas near industrial areas have a poor quality of life due to emissions.
- Traffic Analysis – Spatial imagery can be used to recognize congestion and high-traffic routes. This identification of busy routes can help improve public transportation infrastructure.
2. Public Health Sector
- Mapping Spreading of Disease – Satellite data can be used to monitor the spread of disease in an area that helps policymakers come up with prevention plans. The disease data can be integrated with climatic attributes and nearby water bodies’ presence to analyze how various factors combine to increase the spread.
- Sanitation and Health Facilities Analysis – Spatial data can be used to identify areas with low sanitation and health facilities. Recognizing these areas can help the authorities to come up with a better healthcare management system.
- Vaccination Statistics – GIS technologies and spatial data can be used by authorities to come up with vaccination strategies and track even distribution of vaccines among the population.
3. Agriculture and Farming
- Crop Monitoring – Remote sensing can be used to collect data related to climate, soil nutrients, and sunlight which play a major role in crop productivity.
- Crop Yield Prediction – Satellite imagery can be used to provide insights about climate, weather conditions, and soil nutrients. Using this information, farmers can make better decisions for the best crop yield.
- Farm Animals Monitoring – Spatial analysis can be used to monitor freely roaming livestock which play a major role in methane production and soil and water contamination.
- Soil Analysis – Spatial analysis can help soil specialists retrieve important information about soil like pH level, nitrogen levels, moisture content, etc. which play an important role in a better crop.
Conclusion
In conclusion, in this article, we discussed what is spatial analysis, the types of spatial data, the importance of spatial analysis, the process of spatial analysis, and some applications of spatial analysis. Spatial data can be divided into two main categories, vector and raster data which can be accompanied by additional attribute data. Spatial analysis is important to identify patterns, and decision-making and is an omnipresent technology. The steps involved in spatial analysis are data collection, analysis, and presentation. The technique of spatial analysis is used extensively in many sectors like urban planning, public health, and agriculture which are all discussed above in the article.
Share your thoughts in the comments
Please Login to comment...