What is Data Discovery?
Last Updated :
10 May, 2024
Data discovery is a pivotal step in the data analysis and business intelligence process, allowing organizations to make informed decisions, achieve dynamic growth, and stay competitive in the marketplace.
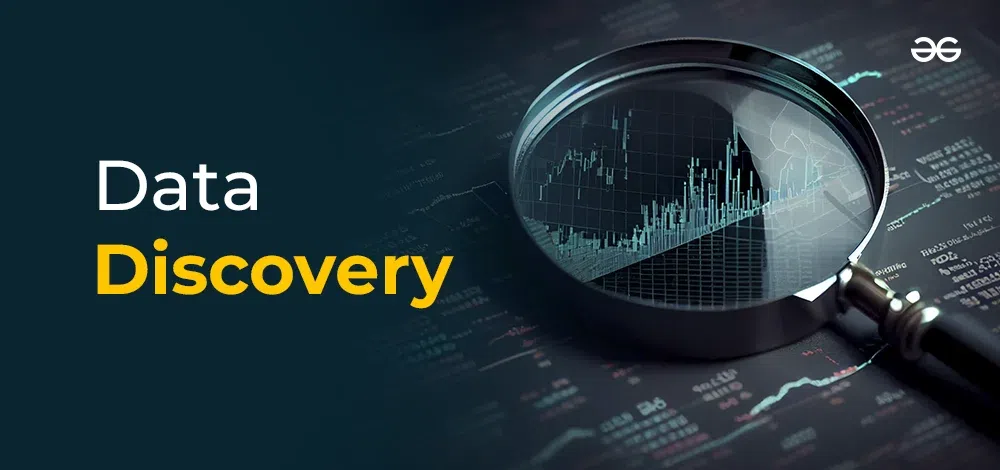
What is Data Discovery?
Data Discovery is the process of identifying patterns, trends, and insights within a meaningful dataset. It includes collecting data from various types of sources and then applying an advanced Data Analytical technique for identifying the patterns and themes within the collected dataset.
It involves examining & analyzing data to uncover the hidden patterns, correlations, connecting patterns and valuable information that can be used for references,decision making & problem solving etc. The main goal of data discovery is to gain a deeper understanding of data, discover new insights and get meaningful and knowledgeable information.
Key Aspects of Data Discovery
- Data Exploration – It includes exploring the dataset to understand its structure, characteristics and relationships between variables in a dataset. It includes the visualizations of data, summary statistics & other data analytical techniques. It includes exploring a large dataset and then finding patterns & meaningful insights in it.
- Recognizing Pattern – Identifying patterns, trends & correlations within a given dataset. It can involve various machine learning algorithms and other data mining techniques to uncover the hidden insights. Recognizing the pattern is very useful as it gives us future insights of a given dataset. The common patterns which are found helps us to understand a given dataset in a very technical way. Therefore, finding a significant pattern and trend is very useful.
- Visualization – Data visualization includes the use of charts, graphs, pictographs and other visual representations to present the data in a very systematic way. Using this visual representation helps to understand, interpret & analyze data in a very effective and easy way. Visualization also helps in spotting down the patterns and trends in the given data graph.
- Interactive Analysis – Interactive analysis enables users to interact with the dataset and modify the variables to gain better perspectives & insights. This often involves use of interactive dashboards and tools that allow users to go deep in specific aspects of a dataset. Interaction of the user with the data helps in better understanding of a dataset.
- Data Profiling – Data Profiling includes examining the quality of dataset, including the missing values, the outliers, the errors & the inconsistencies. Understanding the quality of a given dataset is a crucial factor for accurate data analysis and decision making. Therefore, data profiling is also an important key aspect of data discovery.
Why is Data Discovery important ?
Data discovery has a lot of importance for many reasons particularly in modern data driven decision, problem solving & business intelligence. The following are some key factors explaining why Data Discovery is Important:
- Generating Insights – Data discovery allows us to deeply analyze and understand the pattern in a given dataset,this helps in giving us an insight for the future. For example, business data analytics can gain a better understanding of market trends, customer preferences, planning strategies for growth of business and to compete in the marketplace.
- Informed Decision – Access to meaningful insights derived from data discovery leads to making a firmed decision and strategic choices. This improves efficiency and gets a competitive advantage in a market place.
- Continuous Improvement – Data Discovery is not a one time activity it’s an ongoing process. Regular exploration and analyzing in the business leads to the personal growth of the business as due to continuous analyzation of data it gets to know the pattern & loops to run a business smoothly leading to growth.
- Adaptability to Change – In a dynamic business environment an organization needs to adapt changes very quickly to compete in the marketplace. Data Discovery provides the real time insights, allowing business to respond quickly to the changing market, emerging new trends, strategies and changing the customer preferences.
Categories of Data Discovery
There are two main categories of data discovery:
- Manual Data Discovery :Manual data discovery is the management of a given dataset manually by a highly technical, human data. In earlier years before advancement in machine learning the technicians and the data specialist would manually map and prioritize data, monitor and categorize the metadata and understand,analyze & conceptualize all given data by critical thinking. Manual data discovery is a comparatively slower process and it has the chances of being inaccurate sometimes.
- Smart Data Discovery :Smart data discovery includes the experience of data discovery in a very automated way. As with the development of machine learning and AI smart data discovery has been developed. As Artificial Intelligence is growing, ways like automated data preparation ,data conceptualization, integration and presentation of hidden patterns and insights can be seen growing. Smart data discovery is a comparatively faster and accurate process of data discovery.
History of Data Discovery
The history of Data Discovery is closely associated with the development of data analytics. In the 1970s, Business Intelligence became popular, promoting the use of data to support decision-making. The Data Warehouses were developed in the 1990s, which aimed at collecting data for proper analysis. By the time 2000s arrived, technology had developed highly efficient analytical approaches like data mining and predictive modeling. After 2010, data discovery platforms with specific functionality became popular. They offered a full solution for data preparation, visualization, and discovery. This continuation brought in the 2010s with the Big Data era, necessitating such powerful tools and techniques. Today (roughly around the mid-2010 to the present), AI and ML are revolutionary and therefore, by automating insights generation and employing intelligent processes, data discovery is becoming enhanced.
How is Data Discovered? – Process
The data discovery cycle is a dynamic process that characterizes how organizations repeatedly improve their technique of elaborate insights drawing from data.
1. Define the Subject
- This beginning step is to set the goal/question you are looking to respond to through data discovery very explicitly.
- To do this one should determine those information sources that exist in the organization. This may include databases, spreadsheets, customer relationship management (CRM) systems, or even external one.
2. Data Collection
- This step requires you then to put together these data sources.
- It could be by harvesting data, making it a workable form, and confirming that it is in a common structure among different sources.
3. Data Cleaning and Preparation
- Raw data frequently has erroneous inputs, inconsistency, or missing data. This category deals with the cleaning and readying of the data to ensure its exactness and safeness for analysis.
- Techniques of data cleaning might be identifying and fixing errors, dealing with missing values and transforming data from inconsistent format to a uniform one.
4. Data Analysis and Exploration
- This is the magical part of the whole process!Your job will be about conducting analysis of the pre-processed data which can reveal patterns, trends, and relationships that are of worth investigating.
- At this stage, data visualization tools and statistical techniques are the most usual means of examining the data and revealing hidden trends from various perspectives.
5. Communicate Findings and Iterate
- The next step is to decipher what the data means and then to share your interpretation with the most relevant stakeholders through a simple and concise language.
- It might entail coming up with reports, dashboards, or presentations that enable you to cogently explain the insights you have gathered.
- The data discovery process operates iteratively. Per the knowledge outcomes from the analysis exercise, you may need to revisit your initial research questions, restep the process, or collect new data for additional analysis.
Common Data Discovery Challenges
- Data Quality and Consistency issues: Inaccuracies, inconsistencies, and incomplete data across various sources can hinder the accuracy and reliability of insights gained during the data discovery process misleading conclusions and compromised decision-making due to unreliable data.
- Data Security and Privacy: Ensuring compliance with data privacy regulations and securing sensitive information poses a significant challenge during data discovery, especially with the increasing focus on data protection.
- Data Integration Complexity : Combining and integrating diverse data sources with varying formats and structures can be complex, leading to difficulties in creating a unified view for analysis.
- Scalability Issues: As data volumes continue to grow exponentially, scaling up data discovery processes becomes a challenge, impacting performance and responsiveness leading to slower analysis, increased processing times, and potential system overload in handling large datasets.
- Lack of Standardization: Absence of standardized data formats, definitions, and terminologies across different departments or sources can create confusion and hinder effective collaboration.
- Limited Data Governance: Inadequate data governance practices, including the absence of clear data ownership, stewardship, and documentation, can result in uncontrolled and unmonitored data access.
- Technology Integration Challenges: Implementing and integrating new data discovery tools and technologies within existing IT infrastructure can be challenging, leading to compatibility issues and disruptions.
How to Overcome Common Data Discovery Challenges?
Overcoming common data discovery challenges with the modern data stack helps implementing effective strategies to navigate and extract insights from vast and complex datasets. One key challenge is the sheer volume of data generated, requiring organizations to adopt advanced data discovery tools and technologies that can efficiently sift through and analyze large datasets. Some of the Modern data discovery tools features that effectively address the challenges associated with data discovery:
- Data Quality and Consistency: Automated data profiling and cleansing tools automatically detect and rectify inconsistencies, missing values, and outliers, ensuring data quality prior to analysis. Data lineage tracking enables the monitoring of data origin and transformations, aiding in understanding reliability and error identification. Setting up data validation rules automatically flags suspicious entries for further investigation.
- Data Security and Privacy: In terms of data security and privacy, role-based access control grants data access based on user roles, ensuring regulatory compliance. Data encryption protects sensitive information both at rest and in transit, while data masking and anonymization techniques preserve privacy during analysis.
- Data Integration Complexity: Addressing data integration complexity, data connectors enable seamless integration with various sources, accommodating different formats and structures. Data virtualization creates a unified data view without physically moving it, simplifying analysis. ETL/ELT tools facilitate the extraction, transformation, and loading of data from diverse sources for centralized analysis.
- Scalability Issues: To tackle scalability issues, cloud-based deployment leverages the scalability of the cloud for efficient handling of large datasets. In-memory processing enhances performance, especially for extensive datasets, and parallelized processing distributes tasks across multiple cores or machines for accelerated analysis.
- Lack of Standardization: Dealing with the lack of standardization, data governance tools define standards, policies, and procedures for organizational consistency. Metadata management organizes data definitions and classifications, while data catalogs establish a central repository with searchable descriptions and lineage information.
- Limited Data Governance: In terms of limited data governance, clearly defining data ownership and stewardship ensures accountability for data quality and control. Data audit and logging track access for improved security and compliance, and data usage monitoring identifies potential misuse or inefficiencies. Choosing a data discovery tool with these characteristics ensures the accuracy, reliability, and security of data-driven insights.
Data Discovery Use Cases
Data discovery empowers organizations across various industries to unearth valuable insights, make informed decisions, and boost overall efficiency. Here’s a closer look at some prominent use cases:
1. Business Intelligence (BI) and Reporting
- Challenge: The increasing information overload hits the businesses as the volume of data becomes an issue rather than a source of knowledge.
- Solution: Data discovery applications allow users to look at the data, representation it, and build dashboard and reports. These tools help in identifying KPIs (Key Performance Indicators), tracking progress towards goals, and locating the points where improvement is necessary.
- Benefits: Enhanced decision-making through data-driven insight, better comprehension of businesses’ performance, and the capability of looking into and assessing business shortcomings.
Example: A retailer uses data analytics to explore sale figures for particular products in different regions or among various customer groups. This is how they find high-performing products, take a look at customer shopping habits, and adjust their inventory management.
2. Customer Analytics
- Challenge: Organizations grapple with pinpointing consumer behavior and tastes, therefore staying true to a persona driven mission becomes more difficult.
- Solution: With data discovery, businesses can analyze the data customers have left behind from many sources, like website interactions, purchase history, and social media. Through this, they are able to understand the consumers and what they need, prefer, and loath.
- Benefits: Advanced customer segmentation and targeting, development of personalized marketing communication, augmented customer satisfaction and loyalty, and decreased rate of customer churn.
Example: For instance, an e-commerce platform with the use of data discovery understands how customers interact on their website. They can determine if certain products are often viewed together or track browsing preference, thus recommend products which are relevant to shoppers’ previous purchase history in a personalized fashion.
3. Fraud Detection and Security mechanisms
- Challenge: Both online commerce and financial institutions are subject to massive financial losses through fraudulent activities.
- Solution: Data discovery enables the discovery of irregularities or abnormalities in transactions which can be a sign of unethical behavior. Through the use of these advanced analytics, a suspicious activity can be identified promptly, and immediate intervention can be set in motion.
- Benefits: Saving money from fraud, implementing better means of security, and making sure that customer information doesn’t get compromised.
Example: Bank uses data discovery for a detailed transaction analysis of the customer. They are capable of detecting such movements as unusual large purchases without an adequate cause and those payments that originate from unknown localities. This way detection and prevention of fraud may be made possible and customer accounts protected.
4. Supply Chain Optimization
- Challenge: Inefficient supply networks are resulting in too less in stock, hold up and cost.
- Solution: Data exploration allows businesses to pinpoint available stock, demand prediction and suppliers issues. This is the way of identifying the problems in the chain of supply and enhancing efficiency of the processes.
- Benefits: Appropriate inventory management, no more stockouts plus delays, optimal transportation logistics, and reduced costs.
Example: A manufacturing company applies data analytics to encode and analyze historical sales data and to forecast future demand for their products. This helps them in their resource planning and inventory management, to supply products based on the demand while keeping their stocks optimal to cater to customers’ needs.
5. Healthcare Analytics
- Challenge: The healthcare industry generates vast amounts of data from patient records, clinical trials, and medical research.
- Solution: Data discovery tools help healthcare providers analyze this data to improve patient care, identify disease trends, and develop more effective treatment strategies.
- Benefits: Improved patient outcomes, earlier disease detection, development of personalized treatment plans, and advancements in medical research.
Example: A hospital utilizes data discovery to analyze patient records and identify patients at high risk for certain diseases. This allows them to take preventive measures and provide proactive care.
Conclusion
Therefore, data discovery is a crucial step in the broader process of data analysis & business intelligence. It helps organizations or businesses to make informed decisions, making them dynamic, also leading to continuous personal growth and development which is required by an organization to compete well in the marketplace. That is why data discovery is so important.
Data Discovery- FAQs
What are the methods of data discovery?
Methods include data profiling, visualization, querying, and exploratory data analysis to uncover patterns, relationships, and insights within datasets.
What are the goals of data discovery?
Goals encompass uncovering hidden patterns, identifying trends, validating assumptions, and gaining actionable insights to support decision-making and business strategies.
What are the two types of data discovery?
Ad-hoc data discovery involves spontaneous exploration, while structured data discovery follows a predefined approach for systematic analysis and insight generation.
What are the process of Data Discovery?
Involves data preparation, exploration, visualization, hypothesis testing, pattern recognition, and insight generation, fostering a deeper understanding of the data and informing informed decision-making.
Share your thoughts in the comments
Please Login to comment...