Top Free Dataset Resources for Data Science Projects
Last Updated :
05 Jan, 2024
Imagine your data journey as a quirky adventure! The Iris dataset is a friendly neighborhood where flowers spill their secrets. Titanic data is like solving a dramatic mystery – who survived the shipwreck? Boston Housing is your real estate rollercoaster, predicting house prices with flair. MNIST digits are the whimsical characters in a pixelated parade, while CIFAR-10 is a vibrant carnival of colorful images. Wine Quality, your connoisseur escapade, sipping data for the perfect blend. Netflix Prize data? Your ticket to a cinematic treasure hunt! And don’t forget the Credit Card Fraud dataset, the Sherlock Holmes of anomalies, catching sneaky transactions! Data science: where numbers meet laughter.
In this article we will discuss about List of Datasets you need to practice data science skills.
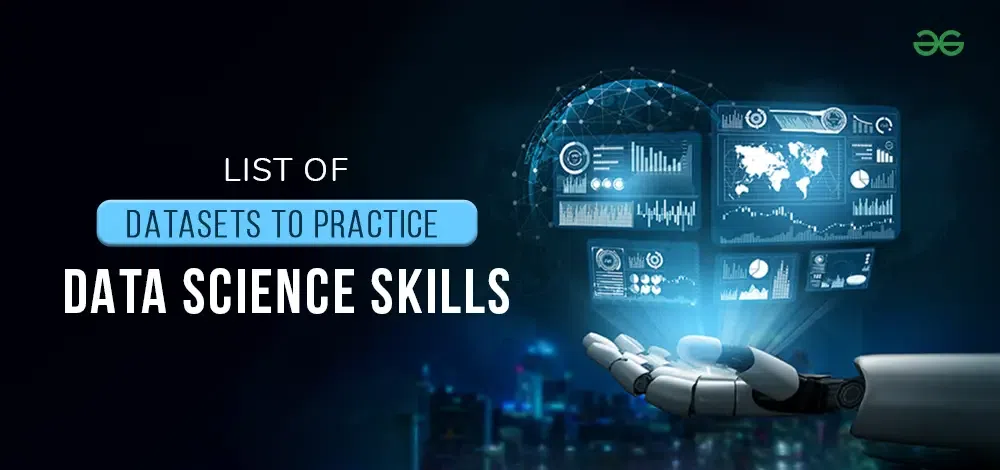
List of Datasets
Data Science
Data Science is a dynamic field that leverages statistical analysis, machine learning, and domain expertise to extract valuable insights from vast datasets. The practice encompasses stages like data collection, cleaning, exploratory analysis, and model building, emphasizing an iterative approach for continuous refinement. Key techniques include machine learning algorithms, statistical analysis, and deep learning, implemented through programming languages like Python and R. Ethical considerations, such as privacy and bias, are crucial, and effective communication of findings to diverse stakeholders is essential. Data scientists employ tools like Matplotlib, Scikit-Learn, and big data technologies to handle and analyze data efficiently. Continuous learning and adaptability to evolving technologies characterize the professional landscape, making data science an integral part of decision-making processes across various industries.
Importance of Hands-on Experience
Hands-on experience with datasets is paramount in data science as it translates theoretical knowledge into practical skills. Working directly with real-world data fosters a deeper understanding of preprocessing challenges, feature engineering nuances, and model intricacies. It hones the ability to address issues like missing data and outliers, enhancing problem-solving skills. This practical engagement cultivates an intuitive grasp of data patterns and a proficiency in selecting and fine-tuning models. Moreover, it instills a sense of confidence and adaptability, vital traits in navigating the diverse challenges posed by data-driven projects. Ultimately, hands-on experience empowers data scientists to apply their expertise effectively in real-world scenarios.
Classic Datasets for Fundamental Skills
Classic datasets like Iris, Titanic, and Boston Housing are fundamental for mastering data science. Iris aids classification, Titanic explores survival analysis, and Boston Housing teaches regression. These classics offer a diverse playground for building essential skills in data analysis, machine learning, and statistical modeling.
Image Classification Datasets
Image classification datasets are essential for developing skills in computer vision. MNIST Handwritten Digits, a staple, involves recognizing handwritten numerals. CIFAR-10/CIFAR-100 offers a diverse set of images for multi-class classification. Fashion MNIST focuses on classifying fashion items. These datasets provide a solid foundation for understanding image classification techniques and algorithms in data science.
Regression and Predictive Modeling Datasets
Regression and predictive modeling datasets are crucial for mastering predictive analytics. The Wine Quality dataset allows regression on wine properties. NYC Taxi Trip Duration involves predicting trip durations, while Credit Card Fraud Detection tackles anomaly detection in transaction data, honing regression skills for varied applications.
Natural Language Processing (NLP) Datasets
Natural Language Processing (NLP) datasets are essential for refining language analysis skills. IMDB Movie Reviews facilitates sentiment analysis. Twitter Sentiment Analysis involves classifying tweets. Amazon Customer Reviews provides diverse textual data, making these datasets valuable resources for honing NLP techniques in data science.
Specific Use Cases
These specific use cases showcase the versatility of datasets in addressing unique challenges across industries, from improving user experience in entertainment platforms to optimizing workforce management and contributing to environmental sustainability.
Anomaly Detection Datasets
Anomaly detection is a critical task in data science that involves identifying patterns in data that do not conform to expected behavior. Here are some example datasets commonly used for anomaly detection:
Recommender System Datasets
Recommender system datasets are used for building algorithms that predict user preferences or recommend items based on past user behavior. Here are some popular datasets for recommender systems:
Healthcare Datasets
Healthcare datasets are crucial for developing and testing data-driven models to improve patient care, diagnostics, and medical research. Here are some notable healthcare datasets:
Text Classification Datasets
Text classification datasets are essential for training and evaluating natural language processing (NLP) models. Here are some widely used text classification datasets:
IoT (Internet of Things) Datasets
IoT (Internet of Things) datasets are essential for developing and testing algorithms related to sensor data, connected devices, and the broader IoT ecosystem. Here are some noteworthy IoT datasets:
Time Series Datasets
Time series datasets are crucial for developing and evaluating models that can make predictions based on temporal patterns. Here are some notable time series datasets:
Clustering Datasets
Clustering is a type of unsupervised learning where the goal is to group similar data points together. There are various clustering algorithms available, and the choice of algorithm depends on the nature of the data and the goals of the analysis. Here are some common clustering algorithms
Conclusion
As we conclude this data-driven escapade, we celebrate the joy of discovery, the thrill of prediction, and the satisfaction of solving mysteries. In the world of data science, where numbers meet laughter, each dataset is a story waiting to be told, and our adventure has been a delightful exploration of this ever-fascinating realm. Cheers to the next chapter in the whimsical world of data!
Share your thoughts in the comments
Please Login to comment...