Social media has become a major platform for communication and entertainment bringing a need to constantly seek innovative ways to keep users engaged and entertained. Instagram one of the leading social media platforms, has a key feature called Instagram Reels, a short-form video format designed to capture the attention of users and keep them scrolling. This has become a major feature since it successfully makes the platform more engaging for most users. From the technical view, Instagram Reels leverages advanced technology, including recommender systems, to curate a personalized and engaging content experience for each user.
In this article, we will explore Understanding Instagram Reels, The Role of Recommender systems, the Impact of Reels on Instagram Systems, Stages of the Recommender System
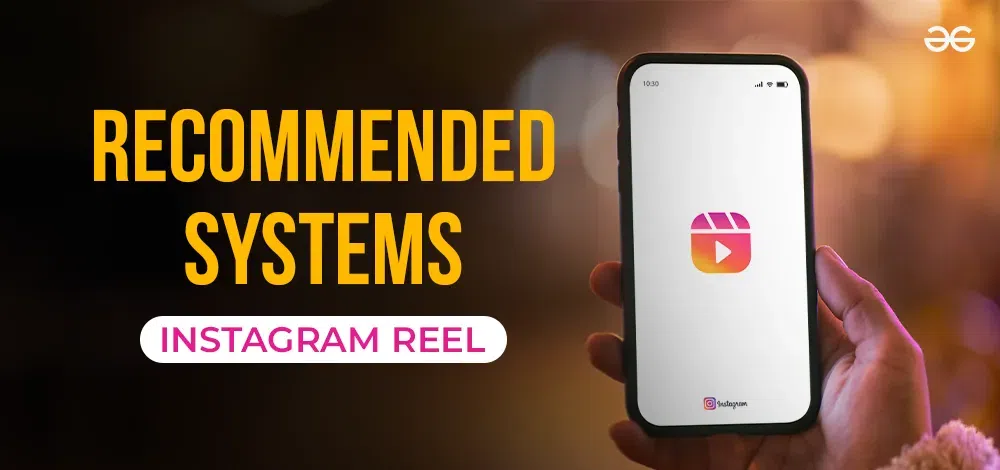
Recommendation Systems
Understanding Instagram Reels
Instagram Reels is a feature that allows users to create and discover short, entertaining videos on the platform. Similar to other short-video platforms which enables users to record and edit 15-second multi-clip videos set to music or other audio clips in the background. It’s a space where creativity flourishes for users or content creators, offering a diverse range of visual content.
The Role of Recommender Systems
Why Recommender Systems? The MAUs (Monthly Active Users) for Instagram are predicted to reach about 2.5 billion by the end of 2024. The measure of how good a platform is by how much it can retain its active users. This simply means how much the users log in to the platform each day and also find enough interesting content to remain. Since different users have personal tastes it becomes necessary to satisfy everyone according to their appetite. To achieve this, an option is to learn about the user’s interest and make personalized suggestions on the content they will see out of the millions of content other users or creators make.
Therefore, Recommender systems are algorithms designed to analyze the user behavior and preferences to recommend content that is likely to be of interest to them. Instagram Reels uses a sophisticated recommender system to enhance user experience by curating a personalized feed of Reels content. Here’s how it works:
1. User Engagement Analysis
Instagram Reels begins by collecting data on user engagement. This includes analyzing the videos users watch, like, comment on, and share. The system takes note of the type of content a user engages with, how long they watch a video, and the interactions that follow. If a user spends more time viewing skits, the recommender system should recommend other new content that tends to be funny. If the user spends time viewing content fashion related, similar content should also be recommended to them.
2. Content Understanding
The recommender system employs advanced analysis techniques to focus more on the content and how users follow. It categorizes videos based on various factors, such as content type, genre, audio used, and visual elements. This could be achieved using the clustering technique and other unsupervised machine-learning techniques. This detailed understanding of content allows the system to identify patterns and correlations between user preferences and specific content characteristics.
3. User Profiling
Instagram Reels creates individual user profiles based on their engagement history and preferences. These profiles are dynamic and evolve as users interact with more content. The system considers factors like the user’s favorite genres, preferred audio tracks, and the creators they follow.
4. Collaborative Filtering
In this recommender system approach, the system utilizes collaborative filtering, a technique that identifies users with similar preferences and recommends content based on what similar users have enjoyed in the past. Instead of just focusing on what a particular user is consuming and bringing more of it, the system recommends new content that was enjoyed by other users with similar profiles. This approach helps in broadening the range of recommendations and introducing users to new and diverse content that aligns with their interests.
5. Real-Time Adaptation
Instagram Reels recommender system is not static but it also continuously adapts to changes in user behavior, ensuring that recommendations stay relevant. If a user’s preferences shift or they start engaging with a new type of content, the system quickly adjusts its recommendations to reflect these changes. This is very useful in avoiding the situation where the user faces born out or gets bored by redundant and over-repeated reels.
The Impact of Reels on Instagram User Experience
The implementation of recommender systems in Instagram Reels has a profound impact on user experience and platform dynamics. Users are presented with a feed of short videos that align closely with their interests, increasing the likelihood of extended engagement on the platform. The personalized nature of the content feed also fosters a sense of connection between users and the platform, enhancing overall satisfaction.
Understanding Instagram Reels from a Technical Perspective
To understand how Instagram reels work from a tech view, let us look particularly at how the Explore feature on the platform works. Instagram has released blogs sometimes to shed light on how their recommender system works on Explore. This is a massive scale of its recommendation system. Due to how the Explore feature is designed to work, the Explore recommendation system stands as one of the largest on Instagram while handling hundreds of millions of daily visitors. The central approach here is leveraging machine learning (ML) and advanced models so that users consistently encounter the most interesting and relevant content.
The Two Towers Neural Networks
Instagram Reels incorporates advanced ML models like Two Towers neural networks. These models enable the recommendation system to efficiently handle billions of content options in real-time. The multi-stage approach to ranking content for users involves retrieval, first-stage ranking, second-stage ranking, and final reranking.
Stages of the Recommender System
Retrieval of Data
The first stage is the retrieval stage which aims to approximate reels that will be highly ranked in later stages. Since there is a massive pool and a vast number of options, a multi-stage funnel approach is used from thousands of candidates and narrowed down to a few hundred. This is a vital stage that employs either heuristics/guessing or sophisticated ML approaches, including real-time and pre-generated sources. Word2Vec algorithm is used by the Two Tower Neural network using arbitrary user features for multi-object retrieval. The item embeddings can then be retained or cached for some time to ensure enough details for retrieval inference.
Instagram Reels also considers a user’s interaction history to retrieve similar items based on past likes, saves, or shares. Filtering out poor-quality items to ensure the retrieved candidates align with the user’s preferences.
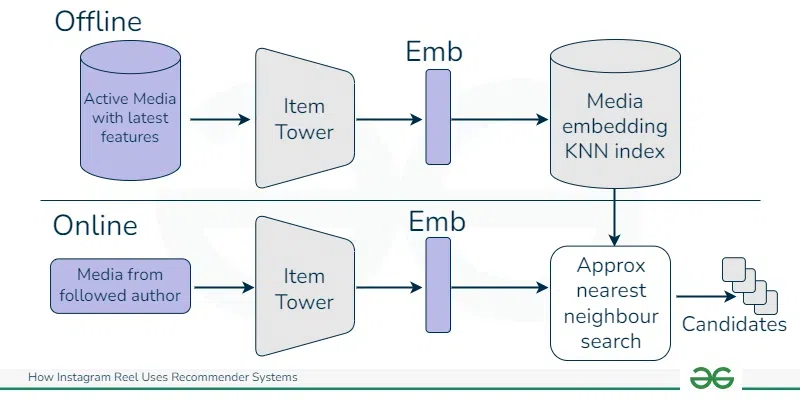
Retriving the Data
Ranking Stages
The ranking stage involves a multi-stage approach, utilizing a first-stage ranker (lightweight model) and a second-stage ranker (heavy model). The Two Tower NN is employed in the first-stage ranking due to its ability to cache data. The goal is to predict the output of the second stage, allowing knowledge distillation from a larger model to a more lightweight one.
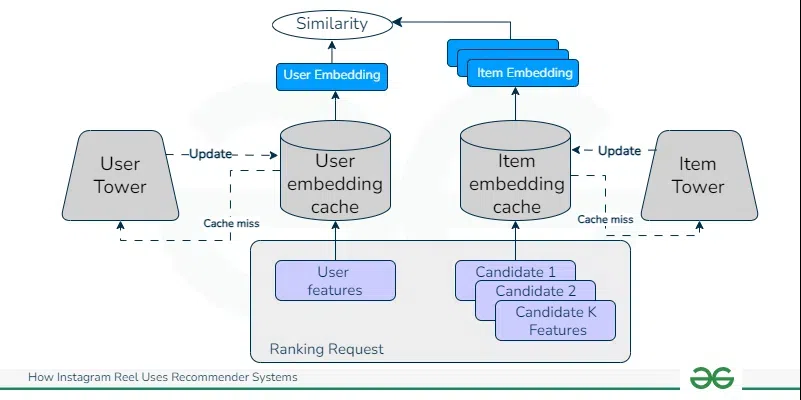
Ranking Stages
MTML Model in Second-Stage Ranking
The second-stage ranker employs a multi-task multi-label (MTML) neural network model, capable of handling powerful user-item interaction features. Predicted probabilities for various engagement events are combined using a value model (VM), representing the value each reel brings to a user.
Final Reranking
To refine the final recommendations, a reranking stage is introduced, considering factors such as content integrity scores and diversity. All this involves a lot of techniques such as fine-tuning the recommender system and adjusting numerous parameters. Instagram employs both Bayesian optimization and offline tuning approaches in particular where the Bayesian optimization allows online tuning, while offline tuning, based on historical data is used to accelerate the process.
Importance of User Feedback
User feedback plays a pivotal role in refining the recommendation system. Instagram values the insights provided by users, utilizing feedback on content preferences and quality to continuously enhance the algorithm. This iterative process ensures that the system aligns closely with user expectations.
Algorithmic Transparency
Balancing algorithmic transparency is crucial for user trust and ethics. Instagram strives to maintain transparency by providing users with insights into how the recommendation system works, ensuring they understand the factors influencing the content they see. This transparency fosters a sense of control and comprehension among users.
Content Moderation for Quality Assurance
We have agreed that quality content is paramount to user experience. Instagram employs robust content moderation strategies to filter out abusive, objectionable, or low-quality content. By actively monitoring and removing content that violates community guidelines, Instagram ensures a safer and more enjoyable platform for users.
User Control Over Content Recommendations
Instagram recognizes the importance of empowering users to control their content recommendations. The platform provides features that allow users to customize their experience, including options to dislike a post and restrict certain content categories. This user-centric approach reinforces Instagram’s commitment to tailoring the platform to individual preferences. This moderates and controls how the contents get to users.
Instagram’s Recommendation System in a Nutshell
Instagram Reels utilizes recommender systems as a key component of its strategy to keep users engaged and entertained. It is a sophisticated blend of machine learning, neural networks, and a multi-stage approach to content curation. By understanding user behavior, analyzing content, and employing real-time adaptation, Instagram uses advanced models and techniques to handle large-scale retrieval and employs a thoughtful ranking process for Reels.
This ensures users are presented with a personalized feed that aligns with their interests. Instagram has proven to be committed to refining its recommendation system and remains a driving force behind user engagement and satisfaction. Since the business of social media continues to grow for top platforms, the role of recommender systems in shaping user interactions is likely to become even more prominent.
How Instagram Reel Uses Recommender Systems – FAQ’s
What makes Instagram Reels stand out in content recommendations?
Instagram Reels leverages advanced recommender systems at an unprecedented scale. It is one of the largest, handling hundreds of millions of daily visitors while employing advanced techniques like Two Towers neural networks and a multi-stage ranking approach.
How does Instagram address the “cold start problem” for new users?
Instagram intelligently generates content for new users by evaluating connections and trending media items. For users with sparse engagement graphs, Instagram evaluates their connections’ accounts to generate content.
What role does the Two Towers neural network play in retrieval?
The Two Towers neural network facilitates efficient retrieval with cacheable user and item embeddings. In retrieval, Two Towers extends Word2Vec, allowing the system to use arbitrary features.
How does Instagram ensure content quality in user interaction history retrieval?
Instagram applies a rule-based approach to filter out poor-quality items from a user’s interaction history. It selectively chooses items based on user interactions to ensure that the retrieved candidates align with the user’s preferences.
What is the significance of multi-stage ranking in Instagram’s recommendation system?
Multi-stage ranking allows Instagram to balance precision and computational intensity. Using a two-stage approach — a lightweight model for the first stage and a heavier model for the second — Instagram can efficiently rank more candidates while maintaining high-quality recommendations.
How does Instagram fine-tune its recommender system for optimal performance?
Instagram employs both Bayesian optimization and offline tuning for parameter optimization. Bayesian optimization allows online tuning, specifying parameters and optimization objectives, while offline tuning leverages historical data to understand the correlation between offline and online metrics.
Share your thoughts in the comments
Please Login to comment...