Overview of Style Transfer (Deep Harmonization)
Last Updated :
16 Jul, 2020
Since humans have started educating themselves of the surrounding world, painting has remained the salient way of expressing emotions and understanding. For example, the image of the tiger below has the content of a tiger from real-world tigers. But notice the style of texturing and colouring is way dependent on the creator.
What is Style Transfer in Neural Networks?
Suppose you have your photograph (P), captured from your phone. You want to stylize your photograph as shown below.
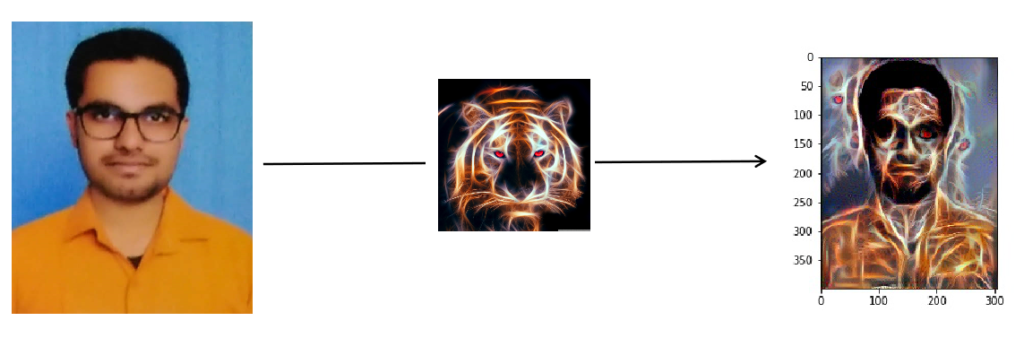
This process of taking the content of one image (P) and style of another image (A) to generating an image (X) matching content of P and style of A is called Style Transfer or Deep Harmonization. You cannot obtain X by simply overlapping P and A.
Architecture & Algorithm
Gatys et al in 2015 showed that it is possible to separate content and style of an image and hence possible to combine content and style of different images. He used a convolutional neural network (CNN), called vgg-19 (vgg stands for Visual Geometric Group) which is 19 layers deep (with 16 CONV layers and 3 FC layers).
vgg-19 is pre-trained on ImageNet dataset by Standford Vision Lab of Stanford University. Gatys used average pooling and no FC layers.
Pooling is typically used to reduce the spatial volume of feature vectors. This helps to reduce the amount of computations. There are 2 types of pooling as depicted below:
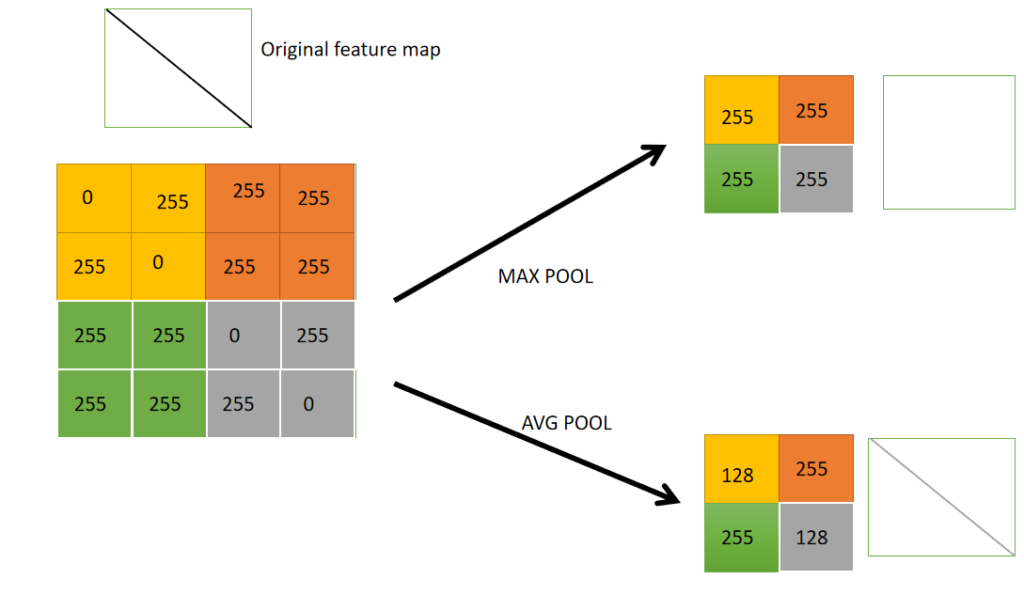
Pooling Process
Losses in Style Transfer:
- Content Loss
Let us select a hidden layer (L) in vgg-19 to calculate the content loss. Let p: original image and x: generated image. Let Pl and Fl denote feature representations of the respective images corresponding to layer L. Then the content loss will be defined as:
- Style Loss
For this, we first have to calculate Gram Matrix. Calculation of correlation between different filters/ channels involves the dot product between the vectorized feature maps i and j at layer l. The matrix thus obtained is called Gram Matrix (G). Style loss is the square of difference between the Gram Matrix of the style image with the Gram Matrix of generated Image.

- Total Loss
is defined by the below formula (with α and β are hyperparameters that are set as per requirement).
The generated image X, in theory, is such that the content loss and style loss is least. That means X matches both the content of P and style of A at the same time. Doing this will generate the desired output.
Note: This is very exciting new field made possible due to hardware optimizations, parallelism with CUDA (Compute Unified Device Architecture) and Intel’s hyperthreading concept.
Code & Output
You can find the
entire code, data files and outputs of Style Transfer (bonus for sticking around : It has code for audio styling as well!) here
__CA__’s Github Repo.
Share your thoughts in the comments
Please Login to comment...