NLP | Categorized Text Corpus
Last Updated :
26 Nov, 2021
If we have a large number of text data, then one can categorize it to separate sections.
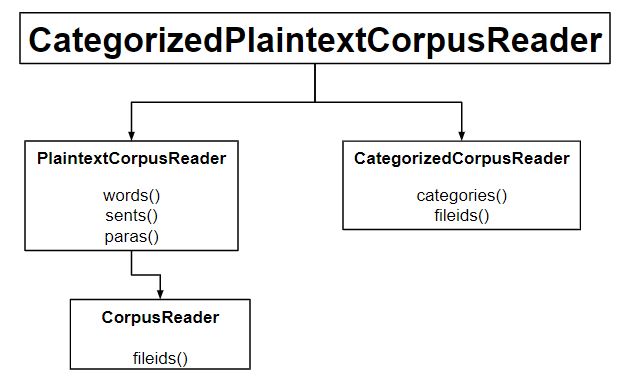
Code #1 : Categorization
Python3
from nltk.corpus import brown
brown.categories()
|
Output :
['adventure', 'belles_lettres', 'editorial', 'fiction', 'government',
'hobbies', 'humor', 'learned', 'lore', 'mystery', 'news', 'religion',
'reviews', 'romance', 'science_fiction']
How to do categorize a corpus?
Easiest way is to have one file for each category. The following are two excerpts from the movie_reviews corpus:
- movie_pos.txt
- movie_neg.txt
Using these two files, we’ll have two categories – pos and neg.
Code #2 : Let’s categorize
Python3
from nltk.corpus.reader import CategorizedPlaintextCorpusReader
reader = CategorizedPlaintextCorpusReader(
'.' , r 'movie_.*\.txt' , cat_pattern = r 'movie_(\w+)\.txt' )
print ( "Categorize : " , reader.categories())
print ( "\nNegative field : " , reader.fileids(categories = [ 'neg' ]))
print ( "\nPositive field : " , reader.fileids(categories = [ 'pos' ]))
|
Output :
Categorize : ['neg', 'pos']
Negative field : ['movie_neg.txt']
Positive field : ['movie_pos.txt']
Code #3 : Instead of cat_pattern, using in a cat_map
Python3
from nltk.corpus.reader import CategorizedPlaintextCorpusReader
reader = CategorizedPlaintextCorpusReader(
'.' , r 'movie_.*\.txt' , cat_map = { 'movie_pos.txt' : [ 'pos' ],
'movie_neg.txt' : [ 'neg' ]})
print ( "Categorize : " , reader.categories())
|
Output :
Categorize : ['neg', 'pos']
Share your thoughts in the comments
Please Login to comment...