Data analysis challenges in the future
Last Updated :
08 Apr, 2024
In the contemporary business landscape, Data Analysis is a crucial asset for businesses across various industries, enabling them to extract valuable insights from the data for informed decision-making. However, that path to successful data analytics is filled with challenges. This article will explore Top Data Analytics Challenges organizations face in different sectors and strategies to overcome them.
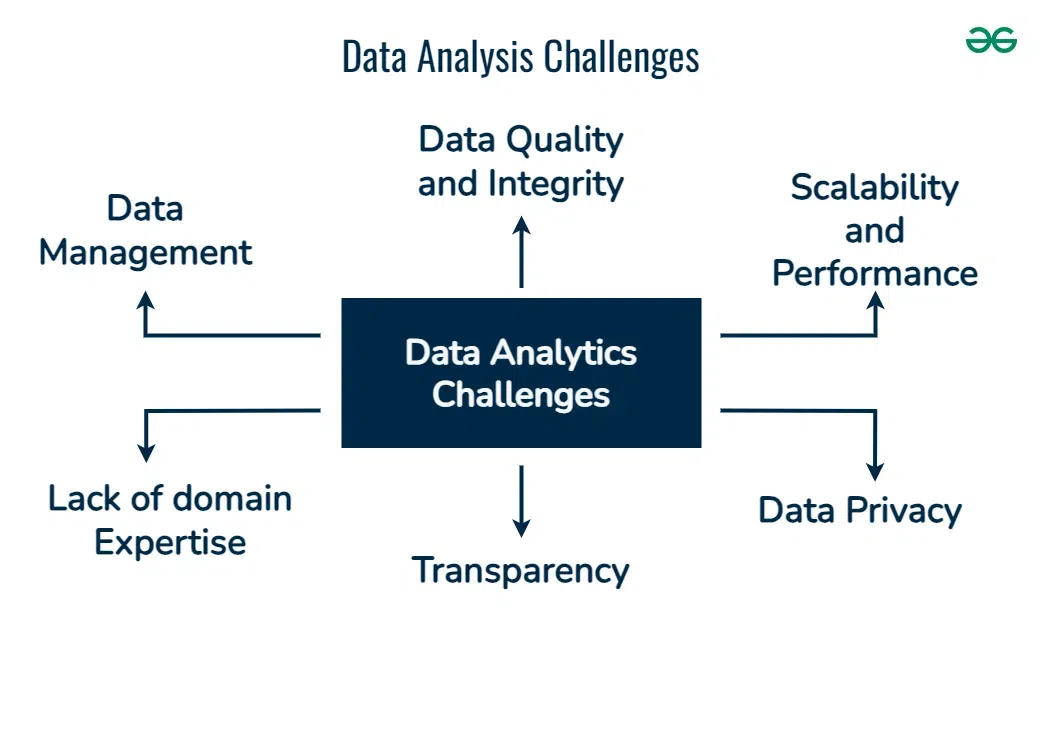
Data Analysis Challenges
When laying out Data Analysis Challenges in a sheet or document, it’s essential to provide clear and structured information to ensure the challenges are easily understandable and actionable. Here’s a suggested layout:
Top 10 Challenges of Data Analytics
Data Analytics has become an important part of modern buisness strategies, offering the promise of valuable insights and informed decision – making. Here are the Top 10 Challenges of Data Analytics we will be discussing below –
Data Integration and Management Challenges
- Overcoming Diverse Data Sources: Finding ways to efficiently combine and utilize data from various sources to gain meaningful insights. Businesses often struggle to combine data from disparate systems and formats to gain meaningful insights.c
- Strategies for Seamless Integration: Developing plans to smoothly incorporate different data sets into existing systems and workflows.
- Recognizing the Shortage of Skilled Data Analysts: Acknowledging the scarcity of qualified professionals capable of interpreting and analyzing data effectively.
- Solutions for Training and Upskilling: Implementing programs to educate and enhance the skills of individuals in data analysis and related fields.
- Implementing Robust Cybersecurity Measures: Putting in place strong defenses and protocols to safeguard data against cyberattacks and breaches.
- Identifying Infrastructure Limitations: Recognizing weaknesses or constraints within existing technological frameworks that hinder data processing and utilization.
- Upgrading and Optimizing Infrastructure: Making necessary improvements to enhance the efficiency and performance of data processing systems and tools.
- Clean and Accurate Data: Ensuring that data sets are free from errors, inconsistencies, and inaccuracies to maintain reliability and trustworthiness.
- Implementing Data Quality Management Processes: Establishing procedures and protocols to maintain high standards of data quality throughout its lifecycle.
Data Security and Privacy Challenges
- Reproducibility Issues: Addressing challenges in replicating research findings or analytical results to ensure reliability and credibility.
- Dealing with Big Data: Managing and analyzing large volumes of data efficiently to extract meaningful insights and avoid overwhelm.
- Ethical Considerations: Contemplating the moral implications and potential consequences of data collection, analysis, and usage.
- Data Privacy: Safeguarding individuals personal information and ensuring compliance with privacy regulations to maintain trust and respect.
- Interpreting Complex Results: Making sense of intricate data analyses and findings, considering nuances and uncertainties to derive accurate interpretations.
- Data Breaches: Ensuring data security to prevent unauthorized access and data breaches is a significant concern.
- Privacy Regulations: Compliance with data privacy regulations such as GDPR, CCPA, etc., while conducting analysis on sensitive data poses challenges.
- Data Anonymization: Balancing the need for data utility with privacy concerns through effective anonymization techniques.
- Data Encryption: Implementing robust encryption methods to protect data in transit and at rest.
- Highlighting the Rising Threats to Data Security: Bringing attention to the increasing risks posed by potential breaches and unauthorized access to sensitive data.
Challenges of Data Analytics in Business
- Aligning Analytics with Business Goals: Ensuring that data analytics efforts are closely aligned with the overarching objectives and strategies of the organization to drive informed decision-making.
- Real-time Analytics: Utilizing data analysis techniques that enable the processing and interpretation of data as it is generated, allowing for immediate insights and actions.
- Scalability Issues: Addressing challenges related to expanding data analytics capabilities to handle larger volumes of data or accommodate growth in the organization’s operations.
- Change Management: Managing the organizational transitions and adjustments necessary to integrate data analytics into existing processes and workflows effectively.
- Cost Management: Controlling expenses associated with data analytics initiatives, including investments in technology, talent, and infrastructure, to optimize resource allocation and maximize ROI.
Ethical and Bias Challenges
- Bias in Data: Identifying and mitigating biases in data collection, analysis, and data interpretation.
- Fairness: Ensuring fairness and equity in algorithms and decision-making processes.
- Transparency: Providing transparency in data analysis methods and results to avoid unethical practices.
- Ethical Use of Data: Adhering to ethical guidelines and principles in handling and analyzing data, especially when dealing with sensitive information.
AI and Machine Learning Challenges
- Interpretability: Understanding and explaining the decisions made by AI and machine learning models.
- Robustness: Ensuring that models perform reliably under different conditions and data distributions.
- Scalability: Scaling machine learning algorithms to handle large volumes of data efficiently.
- Data Bias: Addressing biases in training data that can lead to biased model predictions.
Scalability and Performance Challenges
- Processing Speed: Achieving efficient processing speed when analyzing large-scale data sets.
- Resource Allocation: Optimizing resource allocation for distributed computing environments.
- Scalability: Scaling data analysis techniques to handle increasing data volumes and user demands.
- Real-time Analysis: Performing real-time analysis on streaming data with minimal latency.
Interoperability and Compatibility Challenges
- Integration with Existing Systems: Ensuring compatibility with existing IT infrastructure and systems.
- Data Exchange Standards: Adhering to data exchange standards to facilitate interoperability between different platforms and tools.
- API Compatibility: Ensuring compatibility with various application programming interfaces (APIs) for seamless integration.
Data Governance and Compliance Challenges
- Data Management Policies: Establishing and enforcing data governance policies to ensure data quality, security, and compliance.
- Regulatory Compliance: Adhering to regulatory requirements and standards related to data management, privacy, and security.
- Data Ownership: Defining clear ownership and accountability for data assets within organizations.
Data Visualization and Interpretation Challenges
- Complexity of Visualization: Effectively representing complex data sets and relationships in visualizations.
- Interpretation: Ensuring accurate interpretation of visualization results and avoiding misinterpretation.
- User Engagement: Designing visualizations that engage users and facilitate data-driven decision-making.
Resource Management Challenges
- Cost Optimization: Optimizing resource usage and costs associated with data storage, processing, and analysis.
- Resource Allocation: Efficiently allocating computing resources based on workload demands and priorities.
- Scalability: Scaling resources dynamically to accommodate fluctuating data analysis requirements.
Conclusion
Effective data analysis involves addressing a multitude of challenges spanning from data collection to interpretation and decision-making. These challenges encompass technical, ethical, regulatory, and organizational aspects, requiring comprehensive strategies and solutions. By understanding and proactively addressing these challenges, organizations can harness the full potential of their data assets to drive innovation, make informed decisions, and gain competitive advantages in today’s data-driven world.
Share your thoughts in the comments
Please Login to comment...