What is Quantitative Data Analysis?
Last Updated :
29 Feb, 2024
Quantitative data analysis is like using a magnifying glass to understand numbers better. Quantitative data analysis helps look closely at these numbers to see if there are any interesting patterns or trends hiding in them. In this article, let’s discuss Quantitative Data Analysis in depth.
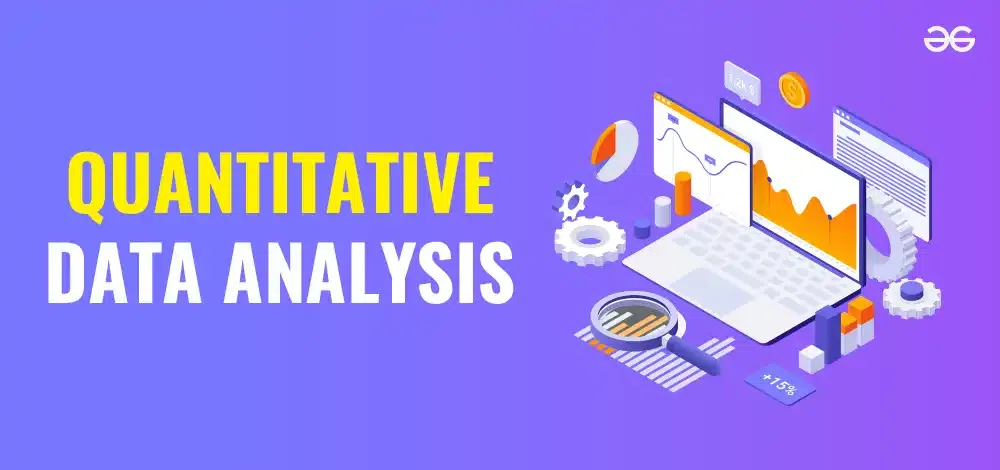
What is Quantitative Data Analysis?
Quantitative data analysis is a method of examining, interpreting, and drawing conclusions from numerical data. It involves the use of statistical techniques and mathematical models to analyze data and identify patterns, trends, and relationships. This type of analysis is common in various fields, including science, business, economic, social sciences, and more.
Quantitative Data Analysis vs Qualitative Data Analysis
Data Analysis is divided into two distinct approaches: quantitative and qualitative. While both contribute significantly to understanding information, they do so in fundamentally different ways. Key differences between Quantitative and Qualitative Data Analysis are below:
Feature |
Quantitative Data Analysis |
Qualitative Data Analysis |
Focus |
Numbers and statistics |
Words, images, non-numerical data |
Data types |
Numbers, measurements, percentages, frequencies |
Interviews, observations, documents, videos, images |
Methods |
Surveys, experiments, observational studies, statistical tests, regression analysis |
Thematic analysis, discourse analysis, grounded theory, narrative analysis |
Strengths |
Objectivity, precision, generalizability |
Rich understanding of experiences, captures emotions and meanings, reveals context |
Weaknesses |
Limited in capturing subjective aspects, overlooks context |
Difficulty generalizing, subjective interpretation, time-consuming analysis |
When to use |
Measuring, comparing, testing hypotheses, generalizing |
Understanding “why” and “how”, exploring experiences, capturing subjective meanings |
To delve more into the depth of differences between them, refer to link.
Why Quantitative Data Analysis is important?
Quantitative data analysis is important because it helps us make sense of the world around us using numbers. It allows us to uncover patterns, trends, and relationships in data, which can lead to valuable insights and informed decision-making. Whether it’s in business, research, or everyday life, quantitative analysis empowers us to identify opportunities, mitigate risks, and optimize processes. Ultimately, it enables us to leverage data to drive success and make better choices.
Steps for Quantitative Data Analysis
- Data Collection: Quantitative data analysis begins with collecting numerical data from various sources such as surveys, experiments, sensors, or databases. The data collected could include anything that can be measured numerically, such as sales figures, website traffic, customer demographics, etc.
- Data Cleaning and Preparation: Before analysis can begin, it’s essential to clean and prepare the data to ensure accuracy and consistency. This involves tasks such as handling missing values, removing outliers, standardizing formats, and structuring the data in a way that facilitates analysis.
- Data Analysis: EDA is a crucial step where analysts explore the data to uncover patterns, trends, and relationships. Techniques such as data visualization, correlation analysis, and dimensionality reduction are used to gain insights into the data.
- Modeling and Prediction: Quantitative data analysis often involves building mathematical models to predict outcomes based on the data. Techniques such as regression analysis, machine learning algorithms, and time series forecasting are used to develop predictive models.
- Interpretation and Reporting: Finally, the results of the analysis are interpreted and reported, often in the form of data visualizations, reports, or presentations. Analysts explain the significance of their findings and provide recommendations based on the insights gained from the analysis.
Methods and Techniques of Quantitative Data Analysis
Quantitative data analysis involves the use of statistical methods to analyze numerical data and draw conclusions. Common methods and techniques used in quantitative data analysis:
- Descriptive Analysis: Descriptive analysis involves summarizing and describing the main features of the data. This includes calculating measures of central tendency (mean, median, mode), measures of dispersion (range, standard deviation), and visualizing the data through histograms, box plots, or scatter plots.
- Hypothesis Testing and Inferential Statistics: In hypothesis testing, researchers formulate hypotheses about relationships between variables and use statistical tests to determine if there is enough evidence to support or reject these hypotheses. Inferential statistics help generalize findings from a sample to a larger population, allowing researchers to make predictions and draw conclusions.
- Cross Tabulations: Cross tabulations, or contingency tables, analyze the association between two categorical variables. They provide a clear overview of how variables relate, helping researchers identify patterns and dependencies within the data.
- Regression Analysis: Regression analysis explores relationships between a dependent variable and one or more independent variables. It quantifies the strength and nature of these relationships, making it a valuable tool for prediction and understanding the impact of variables on the outcome.
- Cohort Analysis: Cohort analysis involves grouping data based on shared characteristics or experiences. It helps researchers track and compare the behavior or outcomes of different cohorts over time, providing insights into trends, patterns, and potential causal relationships.
- Analysis of Variance (ANOVA): ANOVA assesses the variance between group means to determine if there are significant differences. It is particularly useful when comparing means across multiple groups, allowing researchers to identify which groups differ from each other.
- Factor Analysis: Factor analysis explores underlying factors that influence observed variables. It helps researchers identify latent constructs and simplify complex datasets by grouping related variables into common factors, aiding in understanding the underlying structure of the data.
- Monte Carlo Simulation: Monte Carlo simulation involves using random sampling to model and analyze complex systems. It provides a probabilistic approach to assess the impact of uncertainty and variability in different variables, aiding decision-making and risk analysis.
- Cluster Analysis: Cluster analysis categorizes data into groups or clusters based on similarities. It helps identify natural patterns within the data, facilitating the recognition of distinct subgroups or classifications.
- Time Series Analysis:Â Time series analysis examines data collected over sequential time points to identify patterns, trends, and cycles. It is valuable for forecasting future values, understanding temporal dependencies, and making informed decisions based on historical data trends.
Benefits of Quantitative Data Analysis
- Identifying Trends and Patterns: Quantitative analysis helps in uncovering trends and patterns hidden within large datasets.
- Informed Decision Making: It enables making decisions based on factual evidence rather than intuition or guesswork.
- Predictive Insights: By analyzing historical data, it can provide insights into future trends and behaviors.
- Measuring Effectiveness: Quantitative analysis allows measuring the effectiveness of strategies, initiatives, or interventions.
- Risk Assessment: It helps in assessing risks by analyzing numerical data related to various factors.
- Opportunity Discovery: By spotting correlations and relationships in data, it enables discovering opportunities for improvement or growth.
How to Choose the Right Method for your Analysis?
Choosing the right method for quantitative data analysis depends on the nature of the research questions, the type of data, and the objectives of the study.
- Descriptive Questions: If the goal is to summarize and describe your data, descriptive analysis methods such as calculating central tendency, dispersion, and creating visualizations is used.
- Relationship Questions: For questions about relationships between variables, consider regression analysis, correlation analysis, or cross-tabulations.
- Comparison Questions: If you are comparing means across groups, ANOVA or t-tests may be appropriate.
- Continuous Data: Regression analysis, t-tests, and correlation analysis are suitable for analyzing relationships in continuous data.
- Categorical Data: For categorical data, cross-tabulations and chi-square tests are common methods. Cluster analysis may also be useful in grouping similar categories.
- Time Series Data: Time series analysis is essential for studying data collected over time.
- Small Sample Size: With a small sample size, be cautious about complex analyses that may lead to unreliable results. Stick to simpler methods, such as descriptive statistics and basic hypothesis tests.
- Large Sample Size: Large samples allow for more robust analyses, and you can consider more sophisticated methods like factor analysis or structural equation modeling.
- Check Assumptions: Ensure that the chosen method aligns with the assumptions of the statistical test. For example, regression analysis assumes linear relationships between variables.
- Normality and Homogeneity: Some tests, like t-tests and ANOVA, assume normality and homogeneity of variance. Verify these assumptions before proceeding.
Conclusion
Analyzing data in numbers is crucial for making smart decisions based on facts. It helps businesses stand out, find chances to grow, avoid problems, and make things work better. By carefully studying data in a systematic way, experts can discover important information that helps businesses do well.
Quantitative Data Analysis – FAQs
What are some common challenges in quantitative data analysis?
Common challenges include data cleaning and preparation, dealing with missing or incomplete data, selecting appropriate statistical techniques, and interpreting complex results.
How can I improve my skills in quantitative data analysis?
Practice working with real-world datasets, explore different statistical techniques and tools, participate in online courses or workshops, and seek mentorship from experienced analysts or data scientists.
Share your thoughts in the comments
Please Login to comment...