ML | Text Generation using Gated Recurrent Unit Networks
Last Updated :
21 Mar, 2024
This article will demonstrate how to build a Text Generator by building a Gated Recurrent Unit Network. The conceptual procedure of training the network is to first feed the network a mapping of each character present in the text on which the network is training to a unique number. Each character is then hot-encoded into a vector which is the required format for the network.
The data for the described procedure is a collection of short and famous poems by famous poets and is in a .txt format. It can be downloaded from here.
Step 1: Importing the required libraries
Python3
from __future__ import absolute_import, division,
print_function, unicode_literals
import numpy as np
import tensorflow as tf
from keras.models import Sequential
from keras.layers import Dense, Activation
from keras.layers import LSTM
from keras.optimizers import RMSprop
from keras.callbacks import LambdaCallback
from keras.callbacks import ModelCheckpoint
from keras.callbacks import ReduceLROnPlateau
import random
import sys
|
Step 2: Loading the data into a string
Python3
cd C:\Users\Dev\Desktop\Kaggle\Poems
with open ( 'poems.txt' , 'r' ) as file :
text = file .read()
print (text)
|
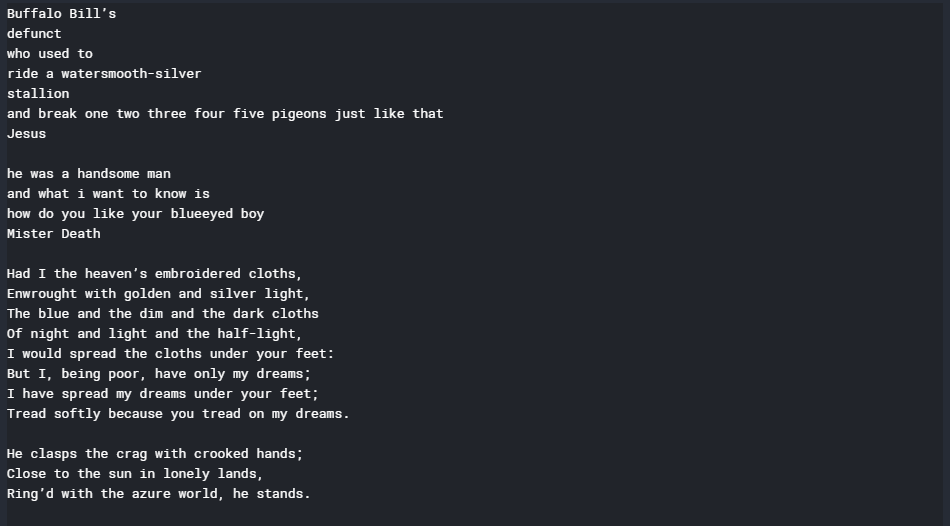
Step 3: Creating a mapping from each unique character in the text to a unique number
Python3
vocabulary = sorted ( list ( set (text)))
char_to_indices = dict ((c, i) for i, c in enumerate (vocabulary))
indices_to_char = dict ((i, c) for i, c in enumerate (vocabulary))
print (vocabulary)
|

Step 4: Pre-processing the data
Python3
max_length = 100
steps = 5
sentences = []
next_chars = []
for i in range ( 0 , len (text) - max_length, steps):
sentences.append(text[i: i + max_length])
next_chars.append(text[i + max_length])
X = np.zeros(( len (sentences), max_length, len (vocabulary)), dtype = np. bool )
y = np.zeros(( len (sentences), len (vocabulary)), dtype = np. bool )
for i, sentence in enumerate (sentences):
for t, char in enumerate (sentence):
X[i, t, char_to_indices[char]] = 1
y[i, char_to_indices[next_chars[i]]] = 1
|
Step 5: Building the GRU network
Python3
model = Sequential()
model.add(GRU( 128 , input_shape = (max_length, len (vocabulary))))
model.add(Dense( len (vocabulary)))
model.add(Activation( 'softmax' ))
optimizer = RMSprop(lr = 0.01 )
model. compile (loss = 'categorical_crossentropy' , optimizer = optimizer)
|
Step 6: Defining some helper functions which will be used during the training of the network
Note that the first two functions given below have been referred from the documentation of the official text generation example from the Keras team.
a) Helper function to sample the next character:
Python3
def sample_index(preds, temperature = 1.0 ):
preds = np.asarray(preds).astype( 'float64' )
preds = np.log(preds) / temperature
exp_preds = np.exp(preds)
preds = exp_preds / np. sum (exp_preds)
probas = np.random.multinomial( 1 , preds, 1 )
return np.argmax(probas)
|
b) Helper function to generate text after each epoch
Python3
def on_epoch_end(epoch, logs):
print ()
print ( '----- Generating text after Epoch: % d' % epoch)
start_index = random.randint( 0 , len (text) - max_length - 1 )
for diversity in [ 0.2 , 0.5 , 1.0 , 1.2 ]:
print ( '----- diversity:' , diversity)
generated = ''
sentence = text[start_index: start_index + max_length]
generated + = sentence
print ( '----- Generating with seed: "' + sentence + '"' )
sys.stdout.write(generated)
for i in range ( 400 ):
x_pred = np.zeros(( 1 , max_length, len (vocabulary)))
for t, char in enumerate (sentence):
x_pred[ 0 , t, char_to_indices[char]] = 1.
preds = model.predict(x_pred, verbose = 0 )[ 0 ]
next_index = sample_index(preds, diversity)
next_char = indices_to_char[next_index]
generated + = next_char
sentence = sentence[ 1 :] + next_char
sys.stdout.write(next_char)
sys.stdout.flush()
print ()
print_callback = LambdaCallback(on_epoch_end = on_epoch_end)
|
c) Helper function to save the model after each epoch in which loss decreases
Python3
filepath = "weights.hdf5"
checkpoint = ModelCheckpoint(filepath, monitor = 'loss' ,
verbose = 1 , save_best_only = True ,
mode = 'min' )
|
d) Helper function to reduce the learning rate each time the learning plateaus
Python3
reduce_alpha = ReduceLROnPlateau(monitor = 'loss' , factor = 0.2 ,
patience = 1 , min_lr = 0.001 )
callbacks = [print_callback, checkpoint, reduce_alpha]
|
Step 7: Training the GRU model
Python3
model.fit(X, y, batch_size = 128 , epochs = 30 , callbacks = callbacks)
|
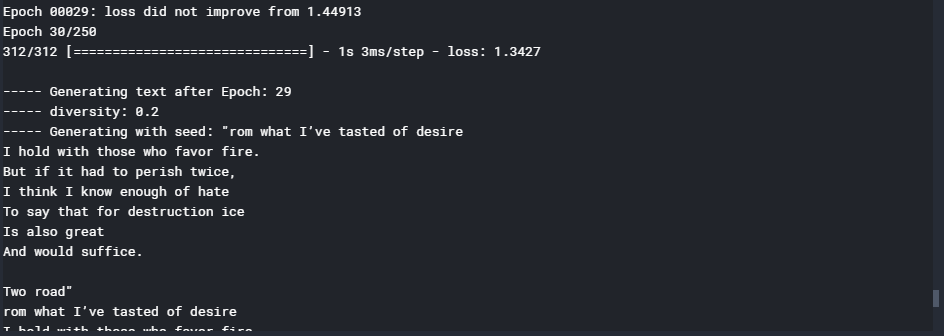
Step 8: Generating new and random text
Python3
def generate_text(length, diversity):
start_index = random.randint( 0 , len (text) - max_length - 1 )
generated = ''
sentence = text[start_index: start_index + max_length]
generated + = sentence
for i in range (length):
x_pred = np.zeros(( 1 , max_length, len (vocabulary)))
for t, char in enumerate (sentence):
x_pred[ 0 , t, char_to_indices[char]] = 1.
preds = model.predict(x_pred, verbose = 0 )[ 0 ]
next_index = sample_index(preds, diversity)
next_char = indices_to_char[next_index]
generated + = next_char
sentence = sentence[ 1 :] + next_char
return generated
print (generate_text( 500 , 0.2 ))
|
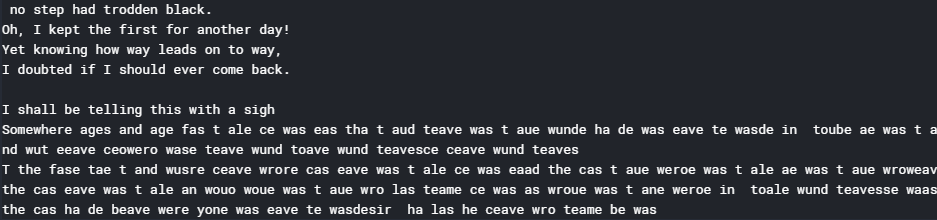
Note: Although the output does not make much sense now, the output can be significantly improved by training the model for more epochs.
Share your thoughts in the comments
Please Login to comment...