Deep learning, a machine learning subset, has prevailed in various areas. Deciphering complex data patterns and representations autonomously is its strength. The symbiotic relationship between task difficulty, dataset dimensions, and neural network design determines deep learning machine learning needs. Deep learning extracts complex traits using multi-layered neural networks. The dynamic interaction between standard machine learning approaches and multi-layered deep neural networks illustrates this relationship.
In this article we will explore the ideal approach mix relies on the aim, dataset size, and neural network architecture. The synergy between machine learning and deep learning continues to innovate, providing unique solutions for many applications.
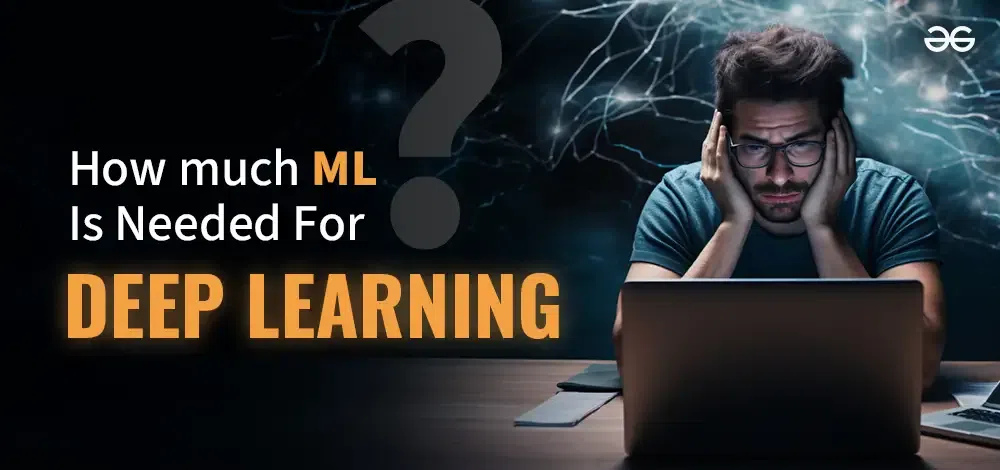
ML needed for Deep Learning
Understanding Deep and Machine Learning
Machine learning (ML) and deep learning (DL) give AI unique skills. With “machine learning” techniques, computers can find patterns and make judgments without scripting. Data-driven algorithms improve with time. In contrast, deep learning uses brain-like artificial neural networks.
Support vector machines, decision trees, and linear regression models are machine learning methods. Qualities-based algorithms forecast outcomes and uncover data patterns. Instead, deep learning mimics brain complexity with multi-layered neural networks. Artificial neurons, or nodes, process information hierarchically. The depth and configuration of these networks matter. Traditional machine learning models with fewer layers are suitable for fundamental patterns. Deep learning models may find complex data correlations and patterns due to their deep brain design.
Deep learning network layers extract hierarchical information to help the model recognize patterns at different abstraction levels. Deep learning excels at audio, picture, and natural language processing, where minute details and contextual nuances matter. Finally, deep learning solves pattern recognition and abstraction problems with advanced neural networks. Different machine learning algorithms exist.
Impact of Deep Leraning in Machine Learning
These transformative technologies wield substantial influence across industries. Deep Learning’s impact is pronounced in image recognition and NLP, reshaping fields like autonomous vehicles and speech recognition.
On the other hand, Machine Learning’s versatile influence is evident in credit scoring and healthcare predictions, showcasing its adaptability and widespread application. Together, they redefine predictive analysis and contribute to the evolution of technology in diverse sectors.
- Task Complexity: Machine learning’s role in task completion depends on complexity. Simple pattern-based jobs only need a little machine learning. Simple language and image recognition may not require sophisticated models. More powerful machine learning techniques emerge when tasks get more complex and need complex interactions. Such scenarios require deep learning, which uses multi-layered neural networks to analyze complex patterns. The job complexity dictates the amount of machine learning needed; therefore, simple or sophisticated methods are chosen.
- Dataset Size: Deep learning dataset size impacts machine learning amount. Deep learning models like big data. The variety of data helps these models identify complex patterns, enhancing accuracy and performance. Machine learning processes and extracts data from big datasets to train complex neural networks. Classic machine learning algorithms may function better for small datasets. With little data, deep learning algorithms may fail to generalize. Therefore, simpler machine learning models may work better. A problem’s dataset size substantially influences the decision between deep learning and classical machine learning.
- Neural Network Architecture: Neural network architecture affects machine learning depth. Shallow neural networks and traditional machine learning algorithms may work for simple data structures. Shallow networks excel at simple data structures. Complex characteristics or hierarchical representations require deep neural networks. Deep networks with many hidden layers record complex data connections. Deep networks may learn hierarchical features and show intricate patterns and representations that shallow networks or common machine-learning models cannot. The job complexity and data structures dictate whether to employ shallow or deep networks, highlighting the importance of network depth in machine learning.
Uses of Machine and Deep Learning
These advanced technologies have wide applications in diverse domains. Machine Learning, adept at credit scoring and healthcare predictions, showcases versatility, while Deep Learning, excelling in image recognition and NLP, revolutionizes tasks like speech recognition and plays a vital role in autonomous vehicles. Both technologies contribute significantly to advancements in predictive analysis, transforming various industries with their unique capabilities.
Uses of Machine Learning
ML can adapt to diverse tasks and methodologies. ML models outperform regression by predicting numerical values from characteristics. Such models predict stock prices in finance. Forecasts assist investors in navigating financial markets. As in healthcare, regression models predict patient outcomes. Doctors can tailor treatment plans with this tool to improve patient care. These ML models classify input data well. Classification models improve email spam detection and cybersecurity. Classifying emails as spam or real strengthens digital defences. Natural language processing sentiment analysis employs categorization models. These models classify textual sentiments to expose human input. This application allows companies to track public opinion and alter strategies across industries.
Uses of Deep Learning
Machine learning subset deep learning excels at image recognition, NLP, and autonomous car development. Deep learning, especially convolutional neural networks, rules picture recognition. These networks learn hierarchical characteristics to classify pictures. In healthcare, security, and entertainment, deep learning in picture identification has enhanced automated system accuracy and efficiency. Deep learning models like RNNs and transformers transformed NLP. These algorithms’ human-like language comprehension and generation have revolutionized language translation and sentiment analysis. Deep learning in NLP improves chatbots, virtual assistants, and language translation. Deep learning helps autonomous automobiles recognize and respond to complicated visual and auditory stimuli. Deep learning algorithms analyze sensor and video data to assist autonomous vehicles in navigating, making decisions, and protecting passengers and pedestrians. Deep learning in autonomous autos advances intelligent transportation systems.
How Deep Learning balances Machine Laerning?
Deep learning or classical machine learning depends on task and resource needs. Considerations for that choice:
- Task Nature : Structured data suits traditional ML models. Decision trees for patterns and linear regression for numerical predictions perform well with tabular data. Interpretability helps stakeholders understand decision-making. Deep learning models thrive at unstructured text, audio, and images. Image recognition and natural language processing benefit from CNNs and RNNs’ ability to extract subtle patterns from complex datasets.
- Interpretability: Transparency makes classical ML models amenable to interpretability. Forecasts are easier to grasp using decision trees and linear model decision pathways. Applications that require transparency and decision-making benefit from this. For stakeholders who value forecast creation, their internal workings may be unclear.
- Computational Resources: Classical or deep learning depends on computational resources. Conventional ML models operate well with little computation. Without considerable resources, decision trees and linear models operate. However, building deep learning models, especially big neural networks, needs many computing resources. GPUs and parallel computing boost deep learning model training efficiency. Using available resources, deep learning can outperform traditional ML in resource-intensive problems.
- Extension to Small Datasets: Because they are less likely to overfit and can make good generalizations with sparse data, classical machine learning techniques might work better with smaller datasets, for deep learning models to generalize well, they frequently need big datasets. They might find it difficult to identify different patterns and might not function as well as more straightforward machine learning models on smaller datasets if there is not enough data.
- Training time: Machine learning models are easier to train than deep learning models, making them preferable for time-sensitive situations. Slower than other big neural network training approaches. Deep learning model training is computationally expensive.
- Application Highlights: In business (credit rating) and healthcare (patient outcome prediction), interpretability and transparency are crucial. Works well with sophisticated, unstructured data processing applications like chatbot natural language comprehension and autonomous car photo recognition. These differences show how machine learning and deep learning have various strengths and factors, making them ideal for different tasks and applications.
Examples of Machine learning and Deep learning
Machine Learning scores credit well using financial data and traditional methods. Deep Learning technologies like RNNs and transformer models boost Siri and Google Assistant speech recognition. These examples show how Machine Learning and Deep Learning improve finance and speech technology.
1. Machine Learning Examples
Traditional ML models excel in credit scoring and more. ML algorithms assess creditworthiness using several factors. Use credit, income, debt, and other financial variables to anticipate loan payback. These systems use decision trees, ensembles, and logistic regression. Traditional ML models are transparent and interpretable, making them ideal for credit scoring, where stakeholders need explicit decision-making insights.
- Forecasts for Healthcare : Machine learning algorithms enhance patient outcomes and treatment planning. Predictive models predict health using demographics, tests, and medical history. ML algorithms can predict sickness and help doctors improve patient outcomes.
- Banking Fraud Detection: Banking uses machine learning to detect fraud rapidly. Machine learning algorithms find fraud and irregularities in user activity, transaction trends, and previous data. To avoid financial fraud and protect customers, banks utilize machine learning (ML) models to spot anomalous spending or transactions that contradict a user’s history.
- Systems for E-Commerce Recommendations: Machine learning algorithms make individualized product recommendations based on browsing history, preferences, and behavior on e-commerce platforms, improving user experience.
On user interactions, previous purchases, and comparable user profiles, online retailers recommend products using machine learning (ML). Consumer engagement and conversions rise.
2. Deep Learning Examples
Deep learning works with unstructured data and complex patterns like speech recognition. Recurrent neural networks and transformer models altered voice recognition. Siri and Google Assistant use deep learning to understand speech. Accents, tones, and linguistic distinctions are captured by these models. Deep learning can develop hierarchical characteristics for complex, hard-to-explain patterns.
Deep learning for speech recognition works for transcription, voice-activated devices, voice-controlled systems, and virtual assistants. Deep learning methods learn to transform speech into text using large speech datasets. Deep learning designs improve speech recognition, making voice-activated system interactions more natural.
- Self-Driving Car Image Recognition: Deep Learning excels at visual detection, notably in autonomous cars. Convolutional Neural Networks (CNNs) let cars recognize and react to objects, people, and road conditions by evaluating camera and sensor data. Deep learning models let self-driving cars identify traffic signs, navigate difficult situations, and make quick visual decisions.
- Medical Image Analysis: Deep Learning transforms medical diagnosis by evaluating complex medical imagery. Convolutional and recurrent deep neural networks can identify medical imaging patterns and anomalies to aid diagnosis and treatment. Deep learning models used in medical imaging, such as MRI or CT scans, can assist doctors in making educated patient care decisions by detecting anomalies, tumors, or early illness indications.
- Transformer Model Language Translation: Deep Learning—especially transformer models—has revolutionized language translation by understanding and producing human-like language. These programs’ capacity to understand linguistic intricacies and context improves translation accuracy and context. Google Translate uses transformer models to provide accurate, contextual translations in many languages, increasing global communication and reducing language barriers.
- Face-ID Security Systems: Deep Learning improves security and authentication in facial recognition technology. Deep neural networks provide unique face signatures for identity verification. Applications include unlocking devices, safeguarding sensitive places, and improving biometric security. Speech recognition systems demonstrate how deep learning can catch complex patterns in unstructured data, unlike credit scoring. This supplementary strategy can be employed in many contexts depending on data and task complexity.
Conclusion
Several factors affect AI deep learning machine learning requirements. The best method depends on task difficulty, dataset size and kind, and neural network design. For smaller jobs with organized data and interpretability, traditional machine learning may work. Deep learning excels with unstructured data workloads with computing resources. Choosing machine learning or deep learning is strategic after understanding the task or application’s goals and limits. As they advance, machine learning and deep learning may create more creative solutions across domains.
Share your thoughts in the comments
Please Login to comment...