As we know Natural language processing (NLP) is an exciting area that has grown at some stage in time, influencing the junction of linguistics, synthetic intelligence (AI), and computer technology knowledge.
This article takes you on an in-depth journey through the history of NLP, diving into its complex records and monitoring its development. From its early beginnings to the contemporary improvements of NLP, the story of NLP is an intriguing one that continues to revolutionize how we interact with generations.
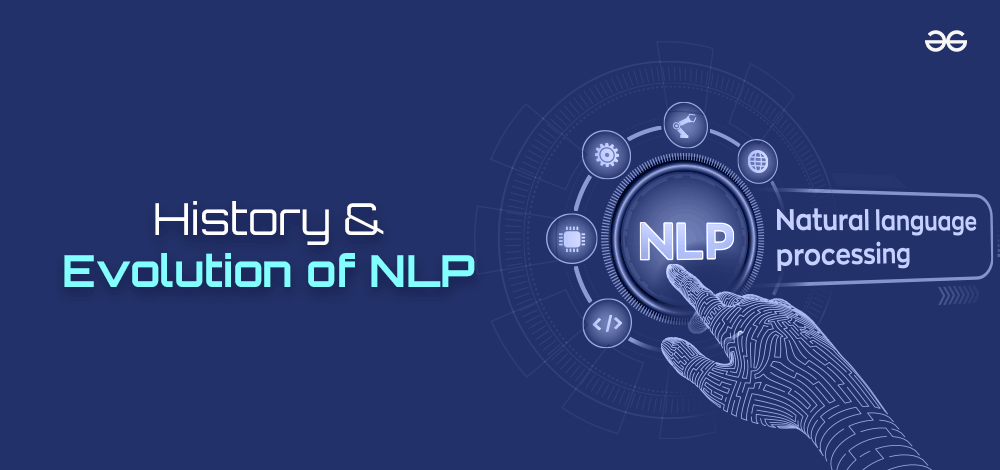
History and Evolution of NLP
History of Natural Language Processing (NLP)
Natural Language Processing (NLP) is a field of computer science and artificial intelligence (AI) concerned with the interaction between computers and human language. Its core objective is to enable computers to understand, analyze, and generate human language in a way that is similar to how humans do. This includes tasks like:
- Understanding the meaning: Being able to extract the meaning from text, speech, or other forms of human language.
- Analyzing structure: Recognizing the grammatical structure and syntax of language, including parts of speech and sentence construction.
- Generating human-like language: Creating text or speech that is natural, coherent, and grammatically correct.
Ultimately, NLP aims to bridge the gap between human communication and machine comprehension, fostering seamless interaction between us and technology.
History of Natural Language Processing (NLP)
The history of NLP (Natural Language Processing) is divided into three segments that are as follows:
The Dawn of NLP (1950s-1970s)
In the 1950s, the dream of effortless communication across languages fueled the birth of NLP. Machine translation (MT) was the driving force, and rule-based systems emerged as the initial approach.
How Rule-Based Systems Worked:
These systems functioned like complex translation dictionaries on steroids. Linguists meticulously crafted a massive set of rules that captured the grammatical structure (syntax) and vocabulary of specific languages.
Imagine the rules as a recipe for translation. Here’s a simplified breakdown:
- Sentence Breakdown: The system would first analyze the source language sentence and break it down into its parts of speech (nouns, verbs, adjectives, etc.).
- Matching Rules: Each word or phrase would be matched against the rule base to find its equivalent in the target language, considering grammatical roles and sentence structure.
- Rearrangement: Finally, the system would use the rules to rearrange the translated words and phrases to form a grammatically correct sentence in the target language.
Limitations of Rule-Based Systems:
While offering a foundation for MT, this approach had several limitations:
- Inflexibility: Languages are full of nuances and exceptions. Rule-based systems struggled to handle idioms, slang, and variations in sentence structure. A slight deviation from the expected format could throw the entire translation off.
- Scalability Issues: Creating and maintaining a vast rule base for every language pair was a time-consuming and laborious task. Imagine the immense effort required for just a handful of languages!
- Limited Scope: These systems primarily focused on syntax and vocabulary, often failing to capture the deeper meaning and context of the text. This resulted in translations that sounded grammatically correct but unnatural or even nonsensical.
Despite these limitations, rule-based systems laid the groundwork for future NLP advancements. They demonstrated the potential for computers to understand and manipulate human language, paving the way for more sophisticated approaches that would emerge later.
The Statistical Revolution (1980s-1990s)
- A Shift Towards Statistics: The 1980s saw a paradigm shift towards statistical NLP approaches. Machine learning algorithms emerged as powerful tools for NLP tasks.
- The Power of Data: Large collections of text data (corpora) became crucial for training these statistical models.
- Learning from Patterns: Unlike rule-based systems, statistical models learn patterns from data, allowing them to handle variations and complexities of natural language.
The Deep Learning Era (2000s-Present)
- The Deep Learning Revolution: The 2000s ushered in the era of deep learning, significantly impacting NLP.
- Artificial Neural Networks (ANNs): These complex algorithms, inspired by the human brain, became the foundation of deep learning advancements in NLP.
- Advanced Architectures: Deep learning architectures like recurrent neural networks and transformers further enhanced NLP capabilities. Briefly mention these architectures without going into technical details.
The Advent of Rule-Based Systems
The 1960’s and 1970’s witnessed the emergence of rule-primarily based systems inside the realm of NLP. Collaborations among linguists and computer scientists precipitated the development of structures that trusted predefined policies to analyze and understand human language.
The aim became to codify linguistic recommendations, at the side of syntax and grammar, into algorithms that would be completed by way of computer systems to machine and generate human-like text.
During this period, the General Problem Solver (GPS) received prominence. They had been developed with the resources of Allen Newell and Herbert A. Simon; in 1957, GPS wasn’t explicitly designed for language processing. However, it established the functionality of rule-based total systems by showcasing how computers must solve issues with the use of predefined policies and heuristics.
What are the current Challenges in the field of NLP?
The enthusiasm surrounding rule-primarily based systems definitely changed into tempered by the realization that human language is inherently complicated. Its nuances, ambiguities, and context-established meanings proved hard to capture virtually through rigid recommendations. As a result, rule-based NLP structures struggled with actual worldwide language applications, prompting researchers to discover possible techniques. While statistical models represented a sizable leap forward, the actual revolution in NLP got here with the arrival of neural networks. Inspired by the form and function of the human mind, neural networks have developed incredible capabilities in studying complicated styles from statistics.
In the mid-2010s, the utility of deep learning strategies, especially recurrent neural networks (RNNs) and lengthy short-time period reminiscence (LSTM) networks, triggered significant breakthroughs in NLP. These architectures allowed machines to capture sequential dependencies in language, permitting more nuanced information and era of text. As NLP persisted in strengthening, moral troubles surrounding bias, fairness, and transparency became more and more prominent. The biases discovered in training information regularly manifested in NLP models raise worries about the functionality reinforcement of societal inequalities. Researchers and practitioners started out addressing those issues, advocating for responsible AI improvement and the incorporation of moral considerations into the fabric of NLP.
The Evolution of Multimodal NLP
Multimodal NLP represents the subsequent frontier in the evolution of herbal language processing. Traditionally, NLP focused, in preference, on processing and understanding textual records.
However, the appearance of multimedia-rich content material on the net and the proliferation of devices organized with cameras and microphones have propelled the need for NLP structures to address an extensive style of modalities at the side of pictures, audio, and video.
- Image Captioning: One of the early programs of multimodal NLP is image captioning, wherein models generate textual descriptions for photos. This challenge calls for the model to now not only successfully understand items inside a photograph but also understand the context and relationships among objects. The integration of visible facts with linguistic know-how poses a considerable assignment; however, it opens avenues for added immersive applications.
- Speech-to-Text and Audio Processing: Multimodal NLP extends its attainment into audio processing, with applications beginning from speech-to-textual content conversion to the evaluation of audio content material. Speech recognition systems, ready with NLP abilities, permit more herbal interactions with devices through voice instructions. This has implications for accessibility and usefulness, making technology extra inclusive for humans with varying levels of literacy.
- Video Understanding: As the amount of video content on the net keeps growing, there may be a burgeoning need for NLP structures to recognize and summarize video data. This entails now not only first-class-recognizing devices and moves inside movies but also knowledge of the narrative shape and context. Video information opens doors to programs in content fabric recommendation, video summarization, and even sentiment evaluation based totally on visible and auditory cues.
- Social Media Analysis: Multimodal NLP becomes especially relevant within the context of social media, wherein users share a vast range of content material fabric, which includes text, pictures, and movement pictures. Analyzing and understanding the sentiment, context, and capability implications of social media content material calls for NLP structures to be gifted in processing multimodal information. This has implications for content material cloth moderation, logo tracking, and trends evaluation on social media platforms.
The Emergence of Explainable AI in NLP
As NLP models become increasingly complicated and powerful, there may be a developing call for transparency and interpretability. The black-box nature of deep mastering models, especially neural networks, has raised issues about their selection-making tactics. In response, the sphere of explainable AI (XAI) has won prominence, aiming to shed light on the internal workings of complicated models and make their outputs more understandable to customers.
- Interpretable Models: Traditional devices studying models, which include choice timber and linear models, are inherently extra interpretable because of their particular illustration of policies. However, as NLP embraced the power of deep studying, mainly with models like BERT and GPT, interpretability has ended up being a big task. Researchers are actively exploring techniques to decorate the interpretability of neural NLP without sacrificing their ordinary performance.
- Attention Mechanisms and Interpretability: The interest mechanism, an essential component of many logo-new NLP models, performs a pivotal position in determining which components of the input collection the version makes an area of expertise at some point of processing. Leveraging interest mechanisms for interpretability entails visualizing the attention weights and showcasing which words or tokens contribute more significantly to the version’s choice. This gives precious insights into how the model processes information.
- Rule-based Totally Explanations: Integrating rule-based totally reasons into NLP includes incorporating human-comprehensible regulations alongside the complex neural community architecture. This hybrid approach seeks balance between the expressive energy of deep mastering and the transparency of rule-primarily based structures. By imparting rule-based reasons, customers can gain insights into why the version made a particular prediction or choice.
- User-Friendly Interfaces: Making AI systems reachable to non-professionals calls for person-friendly interfaces that gift model outputs and causes cleanly and intuitively. Visualization gear and interactive interfaces empower clients to explore model behavior, understand predictions, and verify the reliability of NLP programs. Such interfaces bridge the space between technical experts and prevent-users, fostering a more inclusive and informed interaction with AI.
- Ethical Considerations in Explainability: The pursuit of explainable AI in NLP is intertwined with moral issues. Ensuring that factors aren’t the most effective and accurate but are unbiased and truthful is important. Researchers and practitioners have to navigate the sensitive balance between version transparency and the capability to reveal touchy records. Striking this balance is vital for building acceptance as accurate within AI structures and addressing problems related to duty and equity.
The Evolution of Language Models
Language models form the spine of NLP, powering programs starting from chatbots and digital assistants to device translation and sentiment analysis. The evolution of language models reflects the non-forestall quest for extra accuracy, context cognisance, and green natural language information.
In the early days of NLP, notice the dominance of rule-based systems trying to codify linguistic policies into algorithms. However, the restrictions of these structures in handling the complexity of human language paved the manner for statistical trends. Statistical techniques, along with n-gram models and Hidden Markov Models, leveraged massive datasets to grow to be privy to styles and probabilities, improving the accuracy of language processing obligations.
Word Embeddings and Distributed Representations
The advent of phrase embeddings, along with Word2Vec and GloVe, marked a paradigm shift in how machines constitute and understand words. These embeddings enabled phrases to be represented as dense vectors in a non-forestall vector region, capturing semantic relationships and contextual data. Distributed representations facilitated more excellent nuanced language expertise and stepped forward the overall performance of downstream NLP responsibilities.
The mid-2010s witnessed the rise of deep learning in NLP, with the software of recurrent neural networks (RNNs) and prolonged short-time period memory (LSTM) networks. These architectures addressed the stressful conditions of taking pictures of sequential dependencies in language, allowing models to method and generate textual content with a higher understanding of context. RNNs and LSTMs laid the basis for the following improvements in neural NLP.
In 2017, the advent of the Transformer shape by using Vaswani et al. They marked a contemporary leap forward in NLP. Transformers, characterized via manner of self-attention mechanisms, outperformed previous factors in numerous language obligations.
The Transformer structure has grown to be the cornerstone of the latest trends, allowing parallelization and green studying of contextual facts at some stage in lengthy sequences.
BERT and Pre-educated Models
Bidirectional Encoder Representations from Transformers (BERT), introduced with the aid of Google in 2018, verified the strength of pre-schooling big-scale language models on massive corpora. BERT and subsequent models like GPT (Generative Pre-educated Transformer) completed super performance via studying contextualized representations of words and terms. These pre-professional models, first-class-tuned for unique duties, have turned out to be the pressure behind breakthroughs in understanding natural language.
The evolution of language models persisted with enhancements like XLNet, which addressed boundaries to taking snapshots in a bidirectional context. XLNet delivered a permutation language modeling goal, allowing the model to remember all feasible versions of a sequence. This method similarly progressed the know-how of contextual data and examined the iterative nature of advancements in language modeling.
Ethical Considerations in NLP: A Closer Look
The fast development in NLP has added transformative adjustments in numerous industries, from healthcare and finance to training and enjoyment. However, with splendid power comes first-rate duty, and the ethical issues surrounding NLP have emerged as an increasing number of essentials.
- Transparency and Accountability: The black-discipline nature of a few advanced NLP models poses demanding situations related to transparency and obligation. Users might also moreover need help understanding why a version made a specific prediction or selection. Enhancing transparency includes imparting reasons for model outputs and permitting customers to realize the choice-making manner. Establishing clean traces of responsibility is equally important, making sure that developers and companies take responsibility for the ethical implications of their NLP packages.
- Bias in NLP Models: One of the primary moral concerns in NLP revolves around the capability bias present in education statistics and its impact on model predictions. If schooling records show present societal biases, NLP models may inadvertently perpetuate and make the biases more substantial. For example, biased language in ancient texts or news articles can lead to biased representations in language models, influencing their outputs.
- Fairness and Equity: Ensuring fairness and fairness in NLP programs is a complex assignment. NLP trends should be evaluated for their overall performance at some point by excellent demographic agencies to pick out and mitigate disparities. Addressing problems associated with equity entails now not only refining algorithms but also adopting a holistic approach that considers the numerous views and testimonies of customers.
Conclusion
The data and development of NLP constitute humanity’s extraordinary undertaking to bridge the space between computers and human language. From rule-primarily based systems to the transformational potential of neural networks, each step has helped shape the triumphing landscape of sophisticated NLP trends.
As we approach new opportunities, it’s critical to navigate destiny with moral issues, making sure that the advantages of NLP are used ethically for the welfare of society. As we get to the lowest of the tapestry of NLP, we find ourselves not at the realization but at the beginning of an exciting period wherein the synergy between human language and artificial intelligence continues to evolve.
Share your thoughts in the comments
Please Login to comment...