Bagging vs Boosting in Machine Learning
Last Updated :
01 Jun, 2022
As we know, Ensemble learning helps improve machine learning results by combining several models. This approach allows the production of better predictive performance compared to a single model. Basic idea is to learn a set of classifiers (experts) and to allow them to vote. Bagging and Boosting are two types of Ensemble Learning. These two decrease the variance of a single estimate as they combine several estimates from different models. So the result may be a model with higher stability. Let’s understand these two terms in a glimpse.
- Bagging: It is a homogeneous weak learners’ model that learns from each other independently in parallel and combines them for determining the model average.
- Boosting: It is also a homogeneous weak learners’ model but works differently from Bagging. In this model, learners learn sequentially and adaptively to improve model predictions of a learning algorithm.
Let’s look at both of them in detail and understand the Difference between Bagging and Boosting.
Bagging
Bootstrap Aggregating, also known as bagging, is a machine learning ensemble meta-algorithm designed to improve the stability and accuracy of machine learning algorithms used in statistical classification and regression. It decreases the variance and helps to avoid overfitting. It is usually applied to decision tree methods. Bagging is a special case of the model averaging approach.
Description of the Technique
Suppose a set D of d tuples, at each iteration i, a training set Di of d tuples is selected via row sampling with a replacement method (i.e., there can be repetitive elements from different d tuples) from D (i.e., bootstrap). Then a classifier model Mi is learned for each training set D < i. Each classifier Mi returns its class prediction. The bagged classifier M* counts the votes and assigns the class with the most votes to X (unknown sample).
Implementation Steps of Bagging
- Step 1: Multiple subsets are created from the original data set with equal tuples, selecting observations with replacement.
- Step 2: A base model is created on each of these subsets.
- Step 3: Each model is learned in parallel with each training set and independent of each other.
- Step 4: The final predictions are determined by combining the predictions from all the models.
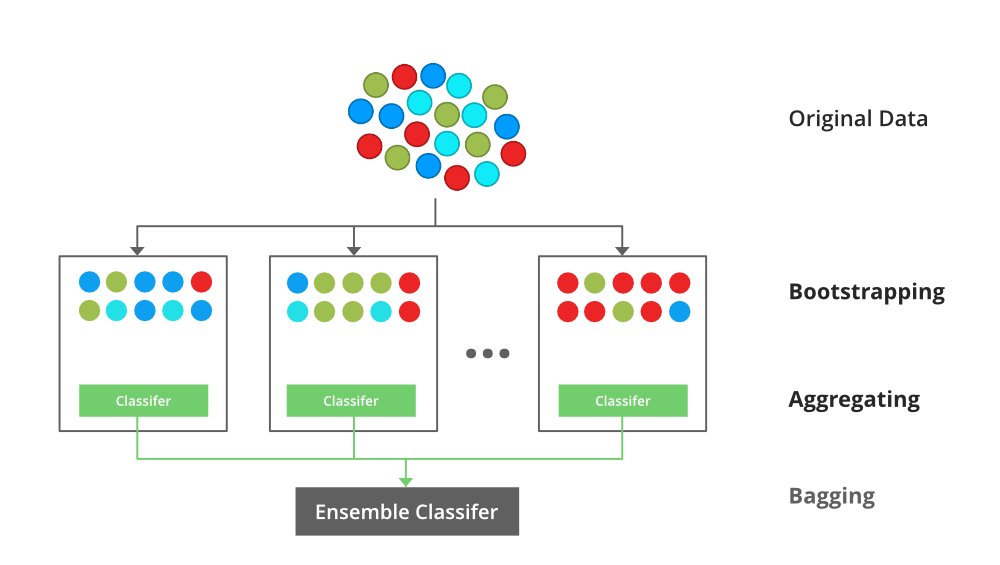
An illustration for the concept of bootstrap aggregating (Bagging)
Example of Bagging
The Random Forest model uses Bagging, where decision tree models with higher variance are present. It makes random feature selection to grow trees. Several random trees make a Random Forest.
To read more refer to this article: Bagging classifier
Boosting
Boosting is an ensemble modeling technique that attempts to build a strong classifier from the number of weak classifiers. It is done by building a model by using weak models in series. Firstly, a model is built from the training data. Then the second model is built which tries to correct the errors present in the first model. This procedure is continued and models are added until either the complete training data set is predicted correctly or the maximum number of models is added.
Boosting Algorithms
There are several boosting algorithms. The original ones, proposed by Robert Schapire and Yoav Freund were not adaptive and could not take full advantage of the weak learners. Schapire and Freund then developed AdaBoost, an adaptive boosting algorithm that won the prestigious Gödel Prize. AdaBoost was the first really successful boosting algorithm developed for the purpose of binary classification. AdaBoost is short for Adaptive Boosting and is a very popular boosting technique that combines multiple “weak classifiers” into a single “strong classifier”.
Algorithm:
- Initialise the dataset and assign equal weight to each of the data point.
- Provide this as input to the model and identify the wrongly classified data points.
- Increase the weight of the wrongly classified data points and decrease the weights of correctly classified data points. And then normalize the weights of all data points.
- if (got required results)
Goto step 5
else
Goto step 2
- End
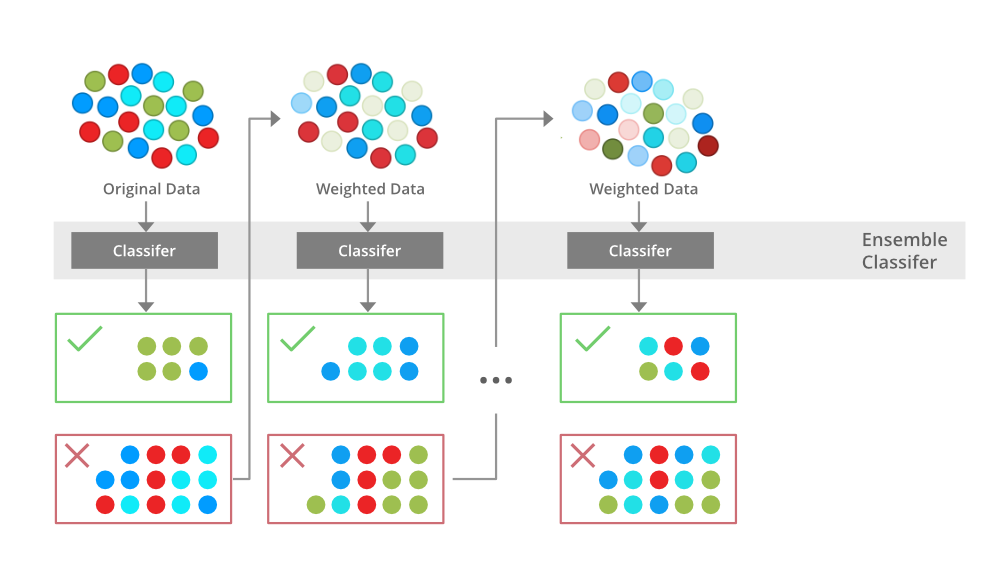
An illustration presenting the intuition behind the boosting algorithm, consisting of the parallel learners and weighted dataset.
To read more refer to this article: Boosting and AdaBoost in ML
Similarities Between Bagging and Boosting
Bagging and Boosting, both being the commonly used methods, have a universal similarity of being classified as ensemble methods. Here we will explain the similarities between them.
- Both are ensemble methods to get N learners from 1 learner.
- Both generate several training data sets by random sampling.
- Both make the final decision by averaging the N learners (or taking the majority of them i.e Majority Voting).
- Both are good at reducing variance and provide higher stability.
Differences Between Bagging and Boosting
S.NO
|
Bagging
|
Boosting
|
1. |
The simplest way of combining predictions that belong to the same type. |
A way of combining predictions that belong to the different types. |
2. |
Aim to decrease variance, not bias. |
Aim to decrease bias, not variance. |
3. |
Each model receives equal weight. |
Models are weighted according to their performance. |
4. |
Each model is built independently. |
New models are influenced by the performance of previously built models. |
5. |
Different training data subsets are selected using row sampling with replacement and random sampling methods from the entire training dataset. |
Every new subset contains the elements that were misclassified by previous models. |
6. |
Bagging tries to solve the over-fitting problem. |
Boosting tries to reduce bias. |
7. |
If the classifier is unstable (high variance), then apply bagging. |
If the classifier is stable and simple (high bias) the apply boosting. |
8. |
In this base classifiers are trained parallelly. |
In this base classifiers are trained sequentially. |
9 |
Example: The Random forest model uses Bagging. |
Example: The AdaBoost uses Boosting techniques |
Share your thoughts in the comments
Please Login to comment...