A/B Testing in Product Management
Last Updated :
06 Feb, 2024
A/B testing, also known as split testing, is a scientific experiment used by product managers to compare two or more versions of a variable and see which one performs better. These variables can be anything from a button design to a feature layout to an entire marketing campaign.
Product managers are constantly seeking ways to optimize user experience and drive product success. A/B testing, a powerful technique in product management, has emerged as a valuable tool for making data-driven decisions and validating product improvements. This article delves into the world of A/B testing, exploring its significance, methodology, and best practices to empower product managers in leveraging this technique for informed decision-making and delivering products that resonate with users.
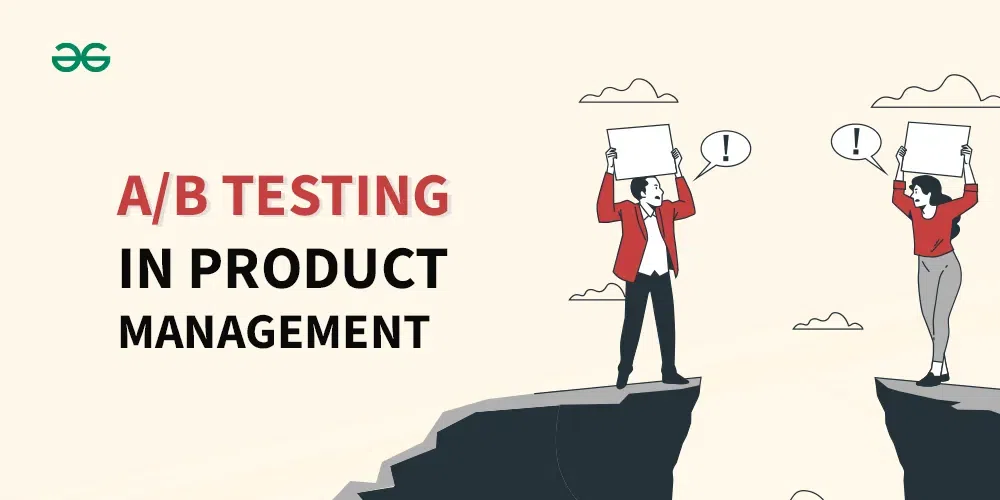
A/B Testing in Product Management
What is A/B Testing?
A/B testing, also known as split testing, is a scientific experiment used by product managers to compare two or more versions of a variable and see which one performs better. These variables can be anything from a button design to a feature layout to an entire marketing campaign. The goal is to gather data-driven insights to make informed decisions about which version will resonate better with users and ultimately achieve your desired outcomes.
Example: Think of it like running a controlled experiment in a restaurant. You want to understand which menu design, one with pictures or detailed descriptions, leads to more orders. To find out, you randomly show each menu to a portion of your customers and track which one leads to more orders. This data then helps you make the informed decision of which menu to implement for all your customers.
Breakdown of the key steps:
- Define your hypothesis: What do you think will happen if you change something? Be specific about the expected impact on a measurable metric (e.g., conversion rate, user engagement).
- Create variations: Develop different versions of the variable you want to test (e.g., two different button designs, two landing page layouts). Ensure they are identical except for the element being tested.
- Split your audience: Randomly show each variation to a portion of your user base. This ensures unbiased results.
- Collect data: Track relevant metrics, such as clicks, sign-ups, or purchases, for each variation over a predetermined period.
- Analyze the results: Use statistical analysis to determine which variation performed significantly better based on your chosen metric.
- Implement the winner: If a variation proves statistically superior, roll it out to the entire user base. If not, iterate and test further based on your learnings.
Importance of A/B Testing for Product Managers:
As a product manager, your goal is to build products that users love and use. A/B testing provides invaluable data to support these efforts:
- Data-driven decision making: It eliminates guesswork and relies on hard data to guide your product roadmap and feature development.
- Increased user engagement: By testing different options, you can optimize features and interfaces for better user experience and higher engagement.
- Improved conversion rates: A/B testing can help you optimize marketing campaigns, website layouts, and calls to action to drive more conversions and growth.
- Reduced risk: Testing mitigates the risk of implementing changes that might negatively impact user behavior.
- Continuous improvement: A/B testing fosters a culture of experimentation and iteration, allowing you to constantly refine your product for maximum impact.
How Product Managers Use A/B Testing?
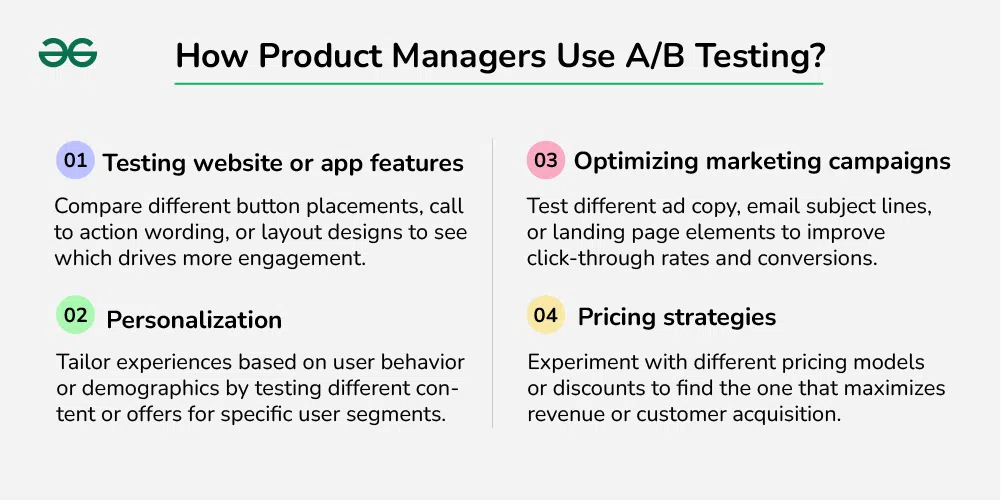
How Product Managers Use A/B Testing?
Product managers can apply A/B testing to various aspects of their work, including:
- Testing website or app features: Compare different button placements, call to action wording, or layout designs to see which drives more engagement.
- Optimizing marketing campaigns: Test different ad copy, email subject lines, or landing page elements to improve click-through rates and conversions.
- Personalization: Tailor experiences based on user behavior or demographics by testing different content or offers for specific user segments.
- Pricing strategies: Experiment with different pricing models or discounts to find the one that maximizes revenue or customer acquisition.
When to Start Using A/B Testing in Product Management?
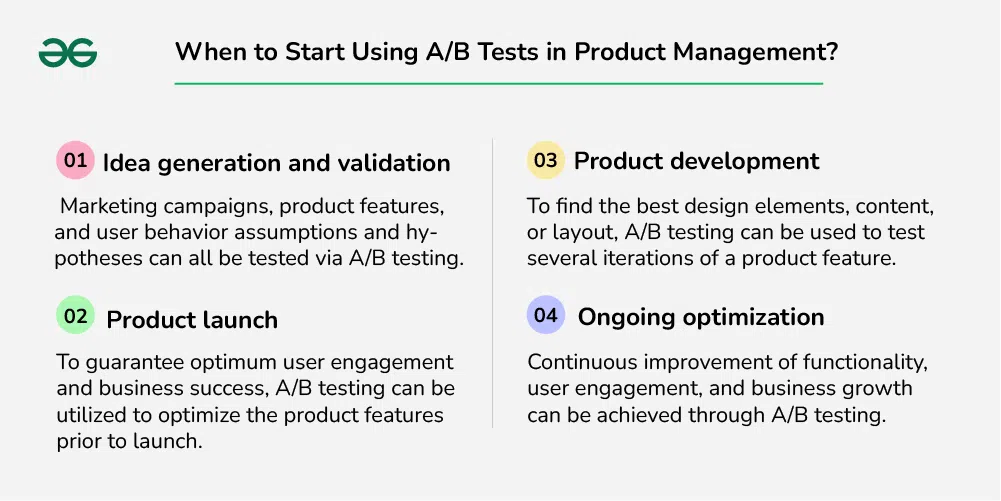
When to Start Using A/B Tests in Product Management?
A/B testing can be applied at different phases of the lifecycle of product management, including:
- Idea generation and validation: Marketing campaigns, product features, and user behavior assumptions and hypotheses can all be tested via A/B testing.
- Product launch: To guarantee optimum user engagement and business success, A/B testing can be utilized to optimize the product features prior to launch.
- Product development: To find the best design elements, content, or layout, A/B testing can be used to test several iterations of a product feature.
- Ongoing optimization: Continuous improvement of functionality, user engagement, and business growth can be achieved through A/B testing.
When should you not use A/B Testing:
While A/B testing is beneficial at every stage of the product development process, there are some situations in which it makes no sense to use it. The first is when there are insufficient users or transactions to conduct a statistically meaningful test; this is frequently the case with corporate firms with low user and/or transaction volumes but substantial contract values.
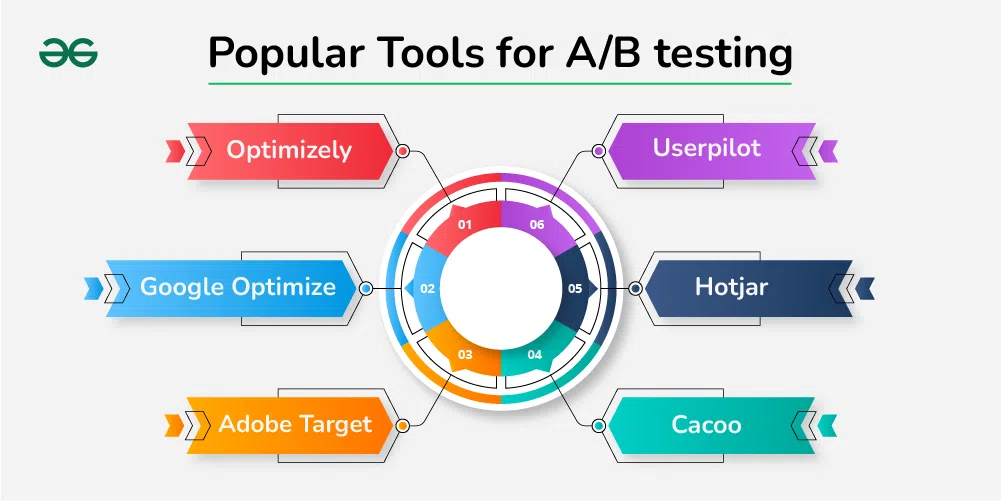
Popular Tools for A/B testing:
- Optimizely
- Google Optimize
- Adobe Target
- Userpilot
- Hotjar
- Cacoo
Types of A/B Testing:
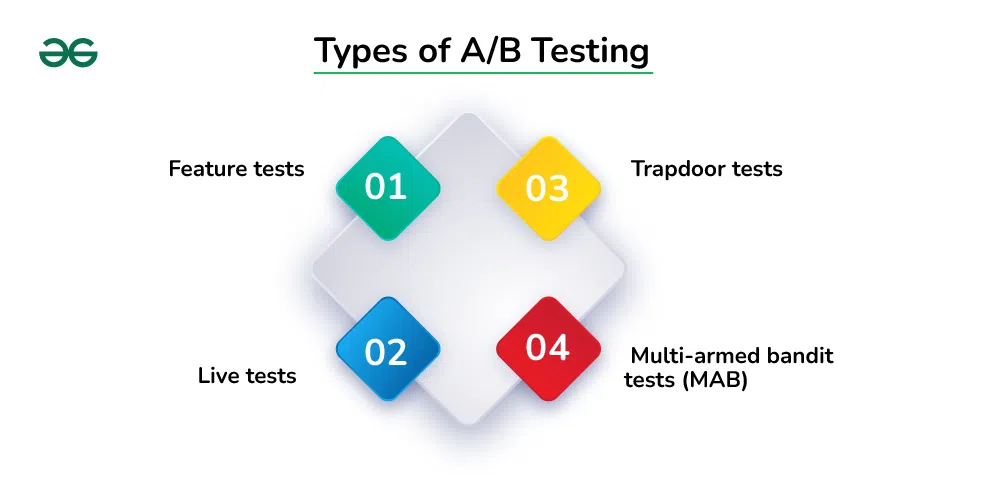
Types of A/B Testing
There are various A/B testing methods tailored to different situations and goals. Here’s a breakdown of the four you mentioned:
1. Feature Tests
What they test: These tests focus on evaluating the impact of introducing new features or redesigning existing ones. They isolate the new feature or flow on a specific group of users while the original version remains available to the rest.
Benefits
- Minimize risk of disruptive changes: By testing with a limited audience, you can identify potential issues and iterate before wider rollout.
- Measure feature-specific impact: Isolate the effects of the new feature from other changes, offering clear evaluation.
- Gather user feedback: Early exposure allows for valuable user feedback to refine the feature before broader release.
Example: Testing a new “Add to Cart” button design on a portion of your e-commerce website users to see if it increases conversion rates.
2. Live Tests
What they test: These tests involve launching experimental changes directly to a segment of your real user base, within the live production environment.
Benefits
- Real-world data: Observe how users interact with the changes in their actual usage context, providing the most realistic data.
- Faster results: Testing with larger user groups can shorten test duration compared to smaller, isolated tests.
- Easier rollout: If successful, the change is already live for a portion of users, simplifying full rollout.
Example: Testing a new homepage layout on a percentage of your website visitors to see if it improves website engagement metrics.
3. Trapdoor Tests
What they test: These tests target users who have opted out of participating in A/B testing. This allows you to observe their behavior without the influence of experimental variations.
Benefits
- Control group comparison: Provides a neutral reference point for evaluating the impact of your A/B tests on the broader user base.
- Identify baseline behavior: Understand how users interact with the existing version before introducing changes.
- Validate test results: Compare results from the main test group with the trapdoor group to verify that the experimental variations are truly causing observed changes.
Example: Testing a new search algorithm while still showing the original results to non-participating users, allowing you to compare their search behavior and measure the effectiveness of the new algorithm.
4. Multi-armed Bandit Tests
What they test: These tests employ machine learning algorithms to dynamically allocate users to different variations in real-time, based on their behavior and predicted outcome.
Benefits
- Continuous optimization: The algorithm constantly learns and adapts, optimizing allocation to the best-performing variation over time.
- Efficient resource allocation: Users are directed to the most relevant variation for them, maximizing conversion rates or other target metrics.
- Reduced testing duration: Faster convergence on the optimal variant compared to traditional A/B testing methods.
Example: A news website uses a multi-armed bandit test to personalize article recommendations for each user, dynamically offering different content based on their past reading preferences and predicted engagement.
Choosing the right type of A/B test depends on your specific goals, resources, and user base. Combine these methods for deeper insights and ensure your testing strategy aligns with your product and business objectives.
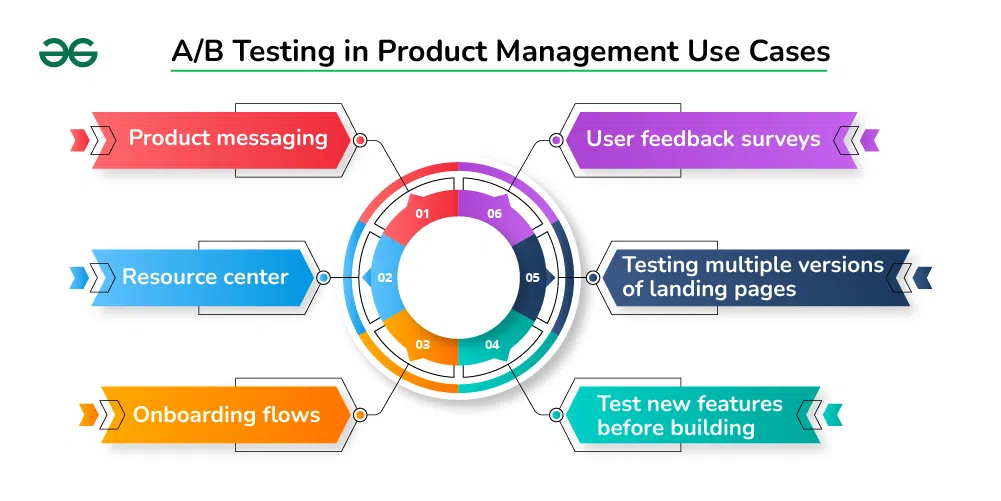
A/B Testing in Product Management Use Cases
In a SaaS product, A/B testing has several applications. You may evaluate how well your instructional materials, in-app messages, and other product features work.
- Product messaging: Present different iterations of your product’s messaging and observe which ones users respond to the best. Check for copy length and design. You might also experiment with other formats; for example, you can determine which technique is best if the group receiving a pop-up notification for a feature has a higher adoption rate than the group receiving introduction tooltips.
- Resource center: You can learn what makes your consumers learn more efficiently by putting your instructional materials to the test.
- Onboarding flows: When it comes to onboarding, you want to minimize time to value and accelerate users’ “aha” moments. A/B testing is a great way to find out which patterns work best, which is important information to know in order to increase user engagement. By understanding how various in-app experiences assist users in hitting milestones more quickly, you can adjust your onboarding process appropriately.
- User feedback surveys: Try a variety of question formats to determine which ones your clients respond to the best. Determine which combination of survey durations and techniques to trigger them—in-app or by email—will result in the most completed surveys.
- Testing multiple versions of landing pages: The most common method for this is most likely A/B testing. A landing page’s A/B testing entails conducting several tests and contrasting two marginally altered versions of the same page to see which has a higher bounce rate.
- Test new features before building: Fake door testing is a technique that differs slightly from standard A/B testing but may also be viewed as a subset of it. The distinction is that you simply wish to test a new feature before releasing it; you are not comparing two varieties.The plan is to act as though the feature has just been released in order to gauge consumer interest.
Tips and Best Practices for A/B Testing:
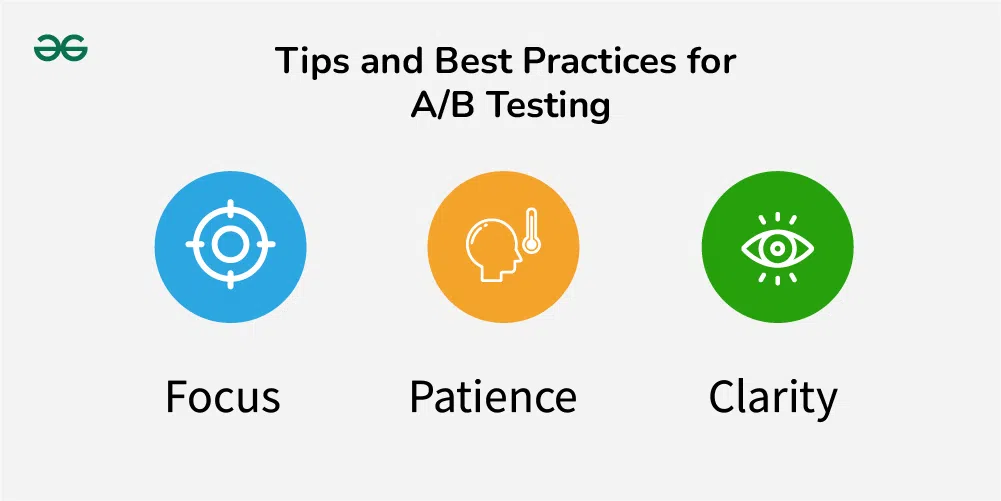
Tips and Best Practices for A/B Testing
- Focus: Resist the urge to test everything at once. Choose one specific element you want to optimize and test variations of that element only. This keeps your results clear and reduces confounding variables.
- Patience: Don’t rush! Run your tests for a sufficient length of time to gather enough data for statistically significant results. This ensures your conclusions are reliable and not due to random fluctuations.
- Clarity: Be crystal clear about your hypotheses and success metrics before you start. What are you hoping to achieve? How will you measure success? Defining these upfront guides your test design and interpretation of results.
Common Challenges in A/B Testing:
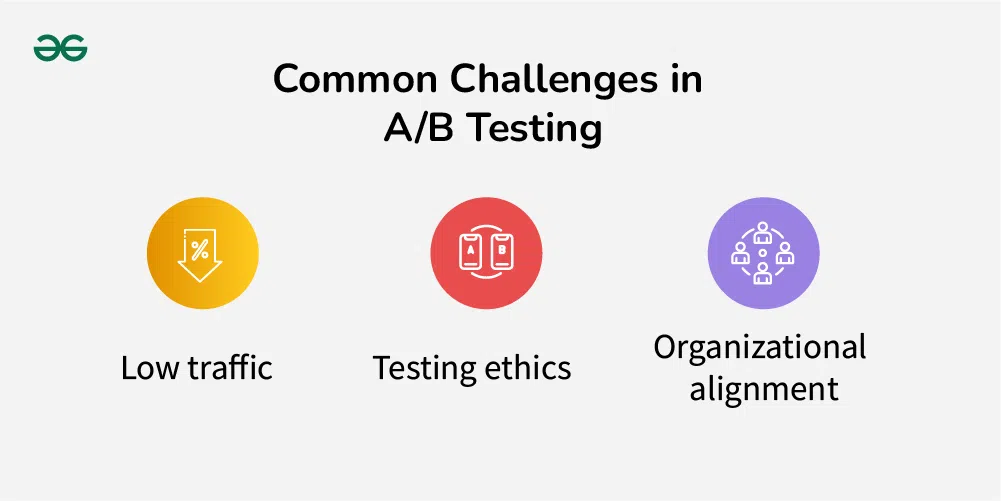
Common Challenges in A/B Testing
- Low traffic: If your website or app doesn’t have enough visitors, gathering statistically significant data becomes difficult. Consider smaller scale tests or alternative research methods like user interviews.
- Testing ethics: Tread carefully with A/B testing. Avoid dark patterns or manipulative tactics that exploit users. Focus on ethical methods that genuinely improve user experience and website performance.
- Organizational alignment: A/B testing requires team buy-in and shared priorities. Ensure everyone involved understands the goals, values ethical testing practices, and contributes to interpreting and implementing results.
Conclusion: A/B Testing
A/B testing is not just a tools, it’s a mindset. It’s the relentless pursuit of data-driven understanding, the constant questioning and learning that fuels innovation. By embracing A/B testing, you become an alchemist, transforming uncertainty into insights, intuition into evidence, and ultimately, your website or app into a goldmine of user satisfaction and business success.
FAQs on A/B Testing:
What is A/B testing of a product?
A/B testing, sometimes referred to as split testing, contrasts two iterations of an idea to see which produces the greatest outcomes. You can test the UI as a whole or just color changes.
What is the A/B testing method?
A/B testing is a technique for contrasting two iterations of a webpage or app to see which one works better. It is also referred to as split testing or bucket testing.
Do product managers do A/B testing?
Product managers may create user-friendly products by utilizing A/B testing. The use of A/B testing has numerous advantages, such as: Product managers or marketers can target and test extremely precise elements. The outcomes are clear and simple to evaluate.
What is A/B testing in agile?
Two variations of things (such web pages, headlines, or call-to-action buttons) are tested against one another and their performances are evaluated in A/B testing, also known as split test or split testing. This indicates that a portion of the target group gets shown both variant A and variant B (played out randomly).
What is A/B testing with example?
A/B testing, also called split testing, is a type of randomized experimentation in which two or more iterations of a variable (web page, page element, etc.) are simultaneously shown to various website visitor segments in order to ascertain which version has the greatest influence and influences business metrics.
Share your thoughts in the comments
Please Login to comment...