Type I and Type II Errors
Last Updated :
28 Mar, 2024
Type I and Type II Errors are central for hypothesis testing in general, which subsequently impacts various aspects of science including but not limited to statistical analysis. False discovery refers to a Type I error where a true Null Hypothesis is incorrectly rejected. On the other end of the spectrum, Type II errors occur when a true null hypothesis fails to get rejected.
In this article, we will discuss Type I and Type II Errors in detail, including examples and differences.
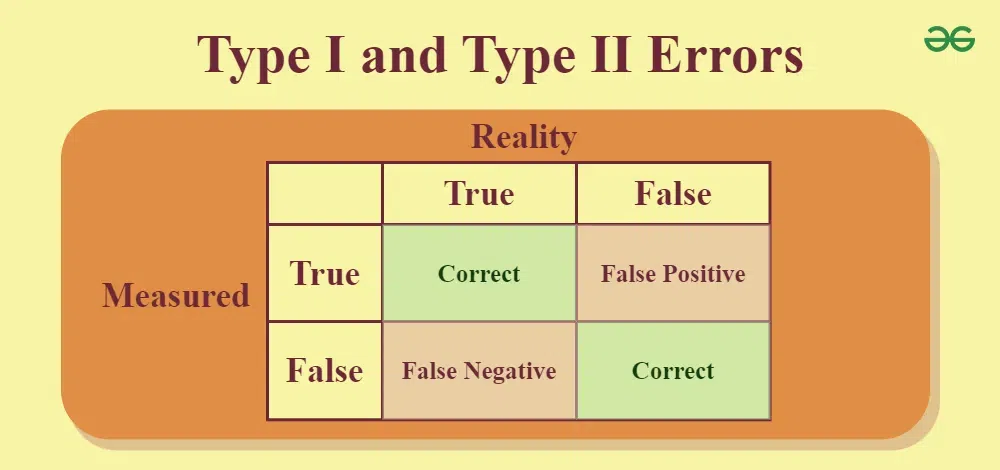
Type I and Type II Error in Statistics
In statistics, Type I and Type II errors represent two kinds of errors that can occur when making a decision about a hypothesis based on sample data. Understanding these errors is crucial for interpreting the results of hypothesis tests.
What is Error?
In the statistics and hypothesis testing, an error refers to the emergence of discrepancies between the result value based on observation or calculation and the actual value or expected value.
The failures may happen in different factors, such as turbulent sampling, unclear implementation, or faulty assumptions. Errors can be of many types, such as
- Measurement Error
- Calculation Error
- Human Error
- Systematic Error
- Random Error
In hypothesis testing, it is often clear which kind of error is the problem, either a Type I error or a Type II one.
What is Type I Error (False Positive)?
Type I error, also known as a false positive, occurs in statistical hypothesis testing when a null hypothesis that is actually true is rejected. In other words, it’s the error of incorrectly concluding that there is a significant effect or difference when there isn’t one in reality.
In hypothesis testing, there are two competing hypotheses:
- Null Hypothesis (H0): This hypothesis represents a default assumption that there is no effect, no difference, or no relationship in the population being studied.
- Alternative Hypothesis (H1): This hypothesis represents the opposite of the null hypothesis. It suggests that there is a significant effect, difference, or relationship in the population.
A Type I error occurs when the null hypothesis is rejected based on the sample data, even though it is actually true in the population.
What is Type II Error (False Negative)?
Type II error, also known as a false negative, occurs in statistical hypothesis testing when a null hypothesis that is actually false is not rejected. In other words, it’s the error of failing to detect a significant effect or difference when one exists in reality.
A Type II error occurs when the null hypothesis is not rejected based on the sample data, even though it is actually false in the population. In other words, it’s a failure to recognize a real effect or difference.
Suppose a medical researcher is testing a new drug to see if it’s effective in treating a certain condition. The null hypothesis (H0) states that the drug has no effect, while the alternative hypothesis (H1) suggests that the drug is effective.
If the researcher conducts a statistical test and fails to reject the null hypothesis (H0), concluding that the drug is not effective, when in fact it does have an effect, this would be a Type II error.
Type I and Type II Errors – Table
The table given below shows the relationship between True and False:
Error Type |
Description |
Also Known As |
When It Occurs |
Type I |
Rejecting a true null hypothesis |
False Positive |
You believe there is an effect or difference when there isn’t |
Type II |
Failing to reject a false null hypothesis |
False Negative |
You believe there is no effect or difference when there is |
Type I and Type II Errors Examples
Examples of Type I Error
Some of examples of type I error include:
- Medical Testing: Suppose a medical test is designed to diagnose a particular disease. The null hypothesis (H0) is that the person does not have the disease, and the alternative hypothesis (H1) is that the person does have the disease. A Type I error occurs if the test incorrectly indicates that a person has the disease (rejects the null hypothesis) when they do not actually have it.
- Legal System: In a criminal trial, the null hypothesis (H0) is that the defendant is innocent, while the alternative hypothesis (H1) is that the defendant is guilty. A Type I error occurs if the jury convicts the defendant (rejects the null hypothesis) when they are actually innocent.
- Quality Control: In manufacturing, quality control inspectors may test products to ensure they meet certain specifications. The null hypothesis (H0) is that the product meets the required standard, while the alternative hypothesis (H1) is that the product does not meet the standard. A Type I error occurs if a product is rejected (null hypothesis is rejected) as defective when it actually meets the required standard.
Examples of Type II Error
Using the same H0 and H1, some examples of type II error include:
- Medical Testing: In a medical test designed to diagnose a disease, a Type II error occurs if the test incorrectly indicates that a person does not have the disease (fails to reject the null hypothesis) when they actually do have it.
- Legal System: In a criminal trial, a Type II error occurs if the jury acquits the defendant (fails to reject the null hypothesis) when they are actually guilty.
- Quality Control: In manufacturing, a Type II error occurs if a defective product is accepted (fails to reject the null hypothesis) as meeting the required standard.
Factors Affecting Type I and Type II Errors
Some of the common factors affecting errors are:
- Sample Size: In statistical hypothesis testing, larger sample sizes generally reduce the probability of both Type I and Type II errors. With larger samples, the estimates tend to be more precise, resulting in more accurate conclusions.
- Significance Level: The significance level (α) in hypothesis testing determines the probability of committing a Type I error. Choosing a lower significance level reduces the risk of Type I error but increases the risk of Type II error, and vice versa.
- Effect Size: The magnitude of the effect or difference being tested influences the probability of Type II error. Smaller effect sizes are more challenging to detect, increasing the likelihood of failing to reject the null hypothesis when it’s false.
- Statistical Power: The power of Statistics (1 – β) dictates that the opportunity for rejecting a wrong null hypothesis is based on the inverse of the chance of committing a Type II error. The power level of the test rises, thus a chance of the Type II error dropping.
How to Minimize Type I and Type II Errors
To minimize Type I and Type II errors in hypothesis testing, there are several strategies that can be employed based on the information from the sources provided:
- Minimizing Type I Error
- To reduce the probability of a Type I error (rejecting a true null hypothesis), one can choose a smaller level of significance (alpha) at the beginning of the study.
- By setting a lower significance level, the chances of incorrectly rejecting the null hypothesis decrease, thus minimizing Type I errors.
- Minimizing Type II Error
- The probability of a Type II error (failing to reject a false null hypothesis) can be minimized by increasing the sample size or choosing a “threshold” alternative value of the parameter further from the null value.
- Increasing the sample size reduces the variability of the statistic, making it less likely to fall in the non-rejection region when it should be rejected, thus minimizing Type II errors.
Difference between Type I and Type II Errors
Some of the key differences between Type I and Type II Errors are listed in the following table:
Aspect |
Type I Error |
Type II Error |
Definition |
Incorrectly rejecting a true null hypothesis |
Failing to reject a false null hypothesis |
Also known as |
False positive |
False negative |
Probability symbol |
α (alpha) |
β (beta) |
Example |
Concluding that a person has a disease when they do not (false alarm) |
Concluding that a person does not have a disease when they do (missed diagnosis) |
Prevention strategy |
Adjusting the significance level (α) |
Increasing sample size or effect size (to increase power) |
Conclusion – Type I and Type II Errors
In conclusion, type I errors occur when we mistakenly reject a true null hypothesis, while Type II errors happen when we fail to reject a false null hypothesis. Being aware of these errors helps us make more informed decisions, minimizing the risks of false conclusions.
People Also Read:
FAQs on Type I and Type II Errors
What is Type I Error?
Type I Error occurs when a null hypothesis is incorrectly rejected, indicating a false positive result, concluding that there is an effect or difference when there isn’t one.
What is an Example of a Type 1 Error?
An example of Type I Error is that convicting an innocent person (null hypothesis: innocence) based on insufficient evidence, incorrectly rejecting the null hypothesis of innocence.
What is Type II Error?
Type II Error happens when a null hypothesis is incorrectly accepted, failing to detect a true effect or difference when one actually exists.
What is an Example of a Type 2 Error?
An example of type 2 error is that failing to diagnose a disease in a patient (null hypothesis: absence of disease) despite them actually having the disease, incorrectly failing to reject the null hypothesis.
What is the difference between Type 1 and Type 2 Errors?
Type I error involves incorrectly rejecting a true null hypothesis, while Type II error involves failing to reject a false null hypothesis. In simpler terms, Type I error is a false positive, while Type II error is a false negative.
What is Type 3 Error?
Type 3 Error is not a standard statistical term. It’s sometimes informally used to describe situations where the researcher correctly rejects the null hypothesis but for the wrong reason, often due to a flaw in the experimental design or analysis.
Share your thoughts in the comments
Please Login to comment...