Role of Domain Knowledge in Data Science
Last Updated :
29 Feb, 2024
Data science for business differs significantly from academic or scientific modeling. In a business context, the focus isn’t solely on identifying interesting patterns for intellectual curiosity. Instead, it’s about finding patterns that drive actionable insights for decision-making. This shift in perspective changes how data scientists collaborate with their teams. Colleagues seeking insights from data aren’t concerned about technical intricacies or theoretical nuances. They want relevant answers to their work and contribute to their decision-making process. They expect data scientists to grasp the commercial significance of their inquiries and understand the context in which they arise. In this article, we will take a closer look at the role of domain knowledge in data science.
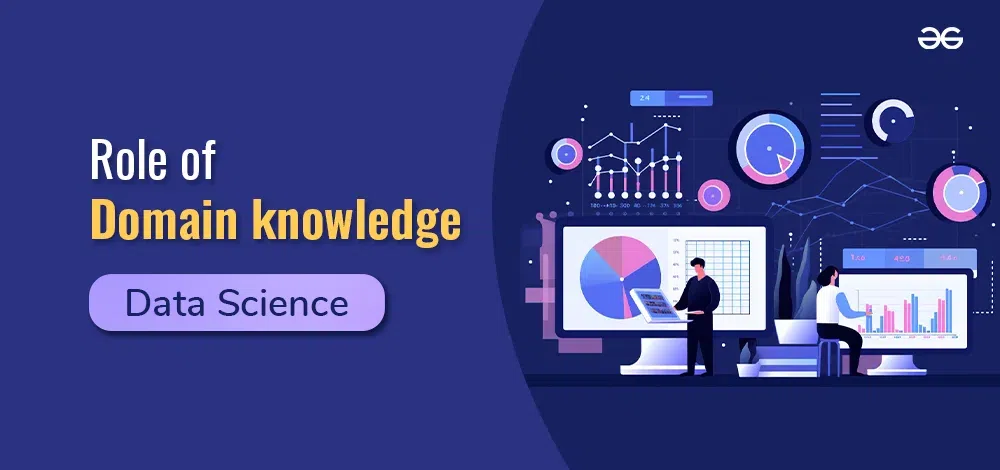
Role of Domain Knowledge
What is Domain Knowledge (Data Science)?
Domain knowledge is a thorough understanding of a particular field, such as healthcare or finance, acquired through experience and education. It entails being familiar with the rules, details, and unique aspects of that field. Domain expertise is crucial in data science. It functions similarly to a map in guiding you through the data jungle. Accurate data analysis is difficult without it. For instance, in the medical field, being familiar with medical jargon and protocols facilitates accurate patient data interpretation.
Similarly, knowing market patterns and economic concepts enables finance professionals to make sound judgments based on financial facts. Domain knowledge is more than simply knowing information; it is about comprehending how things function in a given field. It provides the foundation for data analysis, helping to derive relevant insights and solve real-world challenges.
Responsibilities of a Domain Expert
Domain specialists play an important role in the effective integration of AI technologies into enterprises. Their specialist knowledge and thorough grasp of industry dynamics allow them to define the exact aims and objectives that AI solutions should fulfill. Domain experts can provide useful insights into the types of data needed for analysis and model creation by working closely with data scientists. While data scientists excel at developing algorithms and models, domain specialists provide practical insights into how these models might affect real-world business activities. They have the contextual knowledge required to assess if AI technologies effectively improve operational efficiency, decision-making processes, and revenue growth.
Furthermore, domain experts serve as a bridge between business stakeholders and technological teams. By converting difficult technical jargon into insightful explanations that are relatable to decision-makers, they close the communication gap. This guarantees that AI projects closely match overarching business goals in addition to being technically sound.
Role of Domain Knowledge in Data Science
Regardless of company or sector, a thorough grasp of domain-specific complexities enables data scientists to identify trends, extract insights, and make sound judgments. The following pointers show the value of domain expertise in data science. Let’s take a closer look at them!
- Contextual Understanding: Domain expertise gives data scientists the background context they need to precisely and meaningfully evaluate data. The intricacies of industry-specific procedures, jargon, and laws must be understood whether evaluating financial transactions, medical information, or customer engagement indicators. For instance, in the finance sector, it is essential to comprehend risk management techniques, financial instruments, and regulatory compliance frameworks in order to effectively identify market trends and investment opportunities.
- Feature Engineering: Data scientists can perform meticulous feature engineering, a crucial part of data preprocessing, with the help of domain expertise. With an understanding of the underlying mechanisms and relationships in the domain, they are able to recognize and extract pertinent features from raw data. This method involves preserving the intrinsic predictive value of the data while transforming it into a format that can be modeled. For instance, in cybersecurity, it is possible to extract crucial data for anomaly detection and intrusion prevention systems by comprehending network protocols and threat vectors.
- Data Interpretation: Data scientists can accurately assess data insights and make meaningful conclusions when they have domain expertise. They can use their in-depth understanding of industry dynamics to find significant patterns, anomalies, and correlations in the data. Making wise choices and implementing strategic projects require this discernment. For instance, in the pharmaceutical industry, evaluating data from clinical trials requires knowledge of regulatory requirements, therapeutic efficacy metrics, and drug development processes.
- Model Selection and Evaluation: When choosing the right modeling tools and assessing their efficacy, domain expertise is essential. Experts in a particular domain can identify which models best capture the underlying complexity of the data while meeting the particular analytical goals. Furthermore, they can assess the models effectiveness and make iterative improvements by utilizing domain-specific evaluation metrics. For instance, in marketing analytics, the selection of predictive models for customer segmentation and targeting is influenced by knowledge of market dynamics and consumer behavior.
- Effective Communication: Domain knowledge improves communication between data scientists and domain experts. This also promotes mutual understanding among the teams. By providing stakeholders with results and recommendations in a language they can understand, data scientists support organizational alignment and assist decision-makers. Domain specialists, on the other hand, offer vital ideas and criticism that improve the analytical process and make the findings more relevant.
- Innovation and Problem Solving: Domain expertise fosters innovative thinking and creative problem-solving in data science tasks. Data scientists are able to offer creative solutions for challenging issues by fusing their domain-specific knowledge with their abilities in data analysis. The analytical approaches are strengthened and new avenues for innovation and value creation are made possible by this multidisciplinary approach.
How Can One Acquire Domain Expertise?
Although being a domain specialist in data science is a rare accomplishment, it is critical for analytics and decision making. Previously, obtaining subject expertise necessitated years of actual experience in professions comparable to data scientists or other equivalent occupations. However, the terrain has shifted, presenting new opportunities for building competence.
While practical training remains vital, specialized courses have been developed to train individuals in particular fields. Because the job is so complex and diverse, there are fewer domain-specific degrees than there are data science courses. The path to become a domain expert is more complicated than it was in the past, when the curriculum for prospective data scientists was more straightforward.
It entails exploring multiple subjects and understanding their complexities, which is difficult to encompass in a curriculum that is standardized. Although there aren’t many courses specifically for domain experts, there is a big need for them. In data science, domain specialists are essential because they offer context and insights that are necessary for making well-informed decisions.
Prospective subject matter experts have to look for chances for in-depth instruction and practical experience in the field of their choice. This could entail seeking advanced education in particular fields, working with professionals in the industry, or taking part in projects that are relevant to your field.
Ways to Improve Domain Knowledge
- Research Industry Topics: Exploring industry topics through extensive research is similar to laying a solid foundation for expanding your knowledge. This fundamental stage entails reviewing a wide range of sources, including credible websites, industry reports, and academic papers. By painstakingly gathering these sources and structuring your findings, you not only retain useful information but also ensure accurate reference. This systematic approach enables you to quickly recall relevant information when needed, increasing your understanding of key industry concepts and trends.
- Enroll in Relevant Courses: Taking educational classes related to your line of work is a systematic and complete strategy to broaden your knowledge. These courses, whether offered by traditional educational institutions or online, provide insightful information and practical skills that you may apply in your workplace. Completing these courses not only broadens your knowledge, but also provides you with acceptable credentials that raise your market value. Investing in a formal education can help you progress your career by widening your skill set and displaying a dedication to continuous learning and professional development.
- Interview Industry Experts: Speaking with seasoned employees and business executives provides invaluable insights and perspectives. It’s crucial to show these experts that you comprehend their field of expertise and treat them with respect when communicating with them. By taking thorough notes during these discussions, you might be able to compile a helpful reference that will enable you to absorb more of the knowledge provided by experts.
- Network with Industry Professionals: Developing a robust network within your field is essential for having a better career. You can attend industry events and workshops to network with others who share your interests. It also helps to stay up with current trends. Active networking also helps you build connections that could result in future job possibilities. These people can also be helpful in collaborating for your upcoming projects.
- Identify Industry Challenges and Opportunities: Spotting market opportunities allows you to use your talents and imagination to produce new ideas and contribute to your industry. By remaining vigilant and adaptable, you may brand yourself as someone who faces challenges head on and thinks strategically, directing your career to long-term success and advancement.
- Document and Organize Your Research: To effectively manage and retrieve your research, you must keep thorough records of your findings. You can organize information methodically and monitor your learning process by using tools for note-taking, databases, and spreadsheets. Your organized documentation of your insights makes a priceless resource for analysis and future reference. Updating and backing up your research data on a regular basis guarantees its accessibility and integrity, protecting against loss or inaccurate information.
- Create a Detailed Industry Map: Understanding your industry requires determining who is involved, what they do, and how they interact. Seeing the big picture helps you understand how everything fits together, allowing you to improve things and generate new ideas. Staying flexible and informed in a changing job market requires updating your understanding as things change. This smart approach to understanding your industry allows you to make better decisions and capitalize on opportunities for long-term success.
The Integral Role of Domain knowledge in Data Science
Domain knowledge is essential in data science. It helps understand data in specific areas, enabling better analysis and problem-solving. With domain expertise, data scientists can ask the right questions, find important patterns, and draw useful conclusions. This collaboration with experts leads to better communication and more accurate models, driving success in data science projects.
Enhance Data Analysis with Domain Knowledge
- Contextual Insight: Domain expertise enhances data analysis with essential context, facilitating the interpretation of data within certain businesses. Comprehending the complexities of the market enables analysts to arrive at well-informed recommendations and findings.
- Efficient Problem-Solving: Including domain knowledge improves data analysis problem-solving. More effectively identifying pertinent factors and patterns enables analysts to provide focused and perceptive studies.
- Informed Decision-Making: Knowledge of the domain enables analysts to make well-informed recommendations that are in line with industry problems. Analysts offer practical insights that successfully support corporate objectives by taking contextual elements into account.
How Domain Knowledge Contributes to Formulating Accurate and Relevant Problems?
Domain knowledge is essential for crafting accurate and relevant problems by providing a comprehensive understanding of the domain’s intricacies. With this expertise, individuals can define problems that align with industry specifics, identifying key variables and challenges. Additionally, domain knowledge helps anticipate biases in data, ensuring formulated problems are feasible and realistic. Leveraging this knowledge refines problem statements, addressing pressing issues effectively and leading to actionable insights for decision-making.
Optimizing Data Collection Through Domain Experts
Using domain experts to improve data collection means taking advantage of their specific knowledge to improve the relevance and quality of the data. Organizations can customize data gathering procedures to obtain essential domain-specific insights by enlisting the expertise of subject matter experts.
To ensure that the definition of variables, sources, and collecting techniques are in line with industry standards, experts provide guidance in these areas. This partnership guarantees more thorough and reliable data, enabling enterprises to get useful insights for well-informed decision-making.
Impact of Domain Knowledge on Data Preprocessing and Feature Engineering
- Domain Insight: Domain knowledge informs data preprocessing, helping to identify meaningful attributes and streamline the cleaning process for more efficient transformation.
- Targeted Feature Selection: Domain experts select relevant features based on their understanding of domain dynamics, enhancing model performance by focusing on informative variables.
- Improved Interpretability: Domain expertise ensures the relevance of engineered features, making model outcomes clearer and more trustworthy to stakeholders.
Re-evaluating The Necessity of Domain Knowledge
Reviewing the need for domain knowledge reaffirms its importance in data science. Beyond technical skills, understanding specific industries aids accurate problem-solving and effective analysis. It refines data collection and collaboration with industry experts, aligning projects with industry goals for better insights and solutions in evolving data environments.
Conclusion
In data science, domain expertise is essential for guiding analysts through the intricacies of various industries. It clarifies complex datasets, enabling perceptive interpretations. This knowledge not only increases the analysis’s accuracy but also reveals opportunities and hidden patterns. Furthermore, domain knowledge encourages data science innovation. In conjunction with technical expertise, it facilitates the creation of customized solutions for problems unique to a given industry.
Share your thoughts in the comments
Please Login to comment...