Null Space and Nullity of a Matrix
Last Updated :
11 Jan, 2023
Null Space and Nullity are concepts in linear algebra which are used to identify the linear relationship among attributes.
Null Space:
The null space of any matrix A consists of all the vectors B such that AB = 0 and B is not zero. It can also be thought as the solution obtained from
AB = 0 where A is known matrix of size
m x n
and B is matrix to be found of size
n x k
. The size of the null space of the matrix provides us with the number of linear relations among attributes.
A generalized description:
Let a matrix be
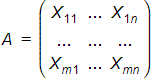
and there is one vector in the null space of A, i.e,
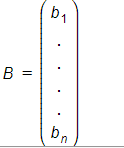
then B satisfies the given equations,
The idea –
1. AB = 0 implies every row of A when multiplied by B goes to zero.
2. Variable values in each sample(represented by a row) behave the same.
3. This helps in identifying the linear relationships in the attributes.
4. Every null space vector corresponds to one linear relationship.
Nullity:
Nullity can be defined as the number of vectors present in the null space of a given matrix. In other words, the dimension of the null space of the matrix
A is called the nullity of A. The number of linear relations among the attributes is given by the size of the null space. The null space vectors
B can be used to identify these linear relationship.
Rank Nullity Theorem:
The rank-nullity theorem helps us to relate the nullity of the data matrix to the rank and the number of attributes in the data. The rank-nullity theorem is given by –
Nullity of A + Rank of A = Total number of attributes of A (i.e. total number of columns in A)
Rank:
Rank of a matrix refers to the number of linearly independent rows or columns of the matrix.
Example with proof of rank-nullity theorem:
Consider the matrix A with attributes {X1, X2, X3}
1 2 0
A = 2 4 0
3 6 1
then,
Number of columns in A = 3
R1 and R3 are linearly independent.
The rank of the matrix A which is the
number of non-zero rows in its echelon form are 2.
we have,
AB = 0
Then we get,
b1 + 2*b2 = 0
b3 = 0
The null vector we can get is
The number of parameter in the general solution is the dimension
of the null space (which is 1 in this example). Thus, the sum of
the rank and the nullity of A is 2 + 1 which
is equal to the number of columns of A.
This rank and nullity relationship holds true for any matrix.
Python Example to find null space of a Matrix:
from sympy import Matrix
A = [[ 1 , 2 , 0 ], [ 2 , 4 , 0 ], [ 3 , 6 , 1 ]]
A = Matrix(A)
NullSpace = A.nullspace()
NullSpace = Matrix(NullSpace)
print ( "Null Space : " , NullSpace)
print (A * NullSpace)
|
Output:
Null Space : Matrix([[-2], [1], [0]])
Matrix([[0], [0], [0]])
Python Example to find nullity of a Matrix:
from sympy import Matrix
A = [[ 1 , 2 , 0 ], [ 2 , 4 , 0 ], [ 3 , 6 , 1 ]]
A = Matrix(A)
NoC = A.shape[ 1 ]
rank = A.rank()
nullity = NoC - rank
print ( "Nullity : " , nullity)
|
Output:
Nullity : 1
Share your thoughts in the comments
Please Login to comment...