Mahotas – Getting Border of labels
Last Updated :
24 Feb, 2023
In this article we will see how we can get the borders of the labels in the labelled image in mahotas. For this we are going to use the fluorescent microscopy image from a nuclear segmentation benchmark. We can get the image with the help of command given below
mahotas.demos.nuclear_image()
Below is the nuclear_image
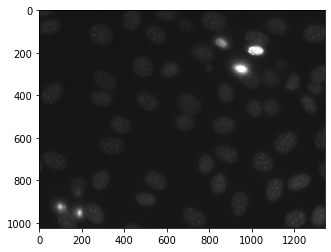
In order to do this we will use mahotas.labeled.borders method
Syntax : mahotas.labeled.borders(labelled_image)
Argument : It takes numpy.ndarray object as argument i.e labelled image
Return : It returns numpy.ndarray object i.e labelled image with only labels border
Note : The input of the this should be the filtered image object which is labelled
In order to filter the image we will take the image object which is numpy.ndarray and filter it with the help of indexing, below is the command to do this
image = image[:, :, 0]
Example 1 :
Python3
import mahotas
import numpy as np
from pylab import imshow, show
import os
f = mahotas.demos.load( 'nuclear' )
f = f[:, :, 0 ]
f = mahotas.gaussian_filter(f, 4 )
f = (f> f.mean())
labeled, n_nucleus = mahotas.label(f)
print ( "Labelled Image" )
imshow(labelled)
show()
relabeled = mahotas.labelled.borders(labeled)
print ( "Labels with border" )
imshow(relabelled)
show()
|
Output :
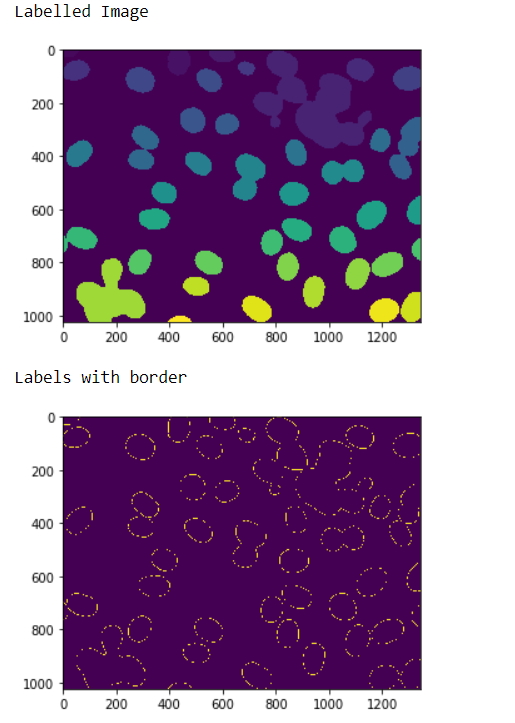
Example 2 :
Python3
import numpy as np
import mahotas
from pylab import imshow, show
img = mahotas.imread( 'dog_image.png' )
img = img[:, :, 0 ]
gaussian = mahotas.gaussian_filter(img, 15 )
gaussian = (gaussian > gaussian.mean())
labelled, n_nucleus = mahotas.label(gaussian)
print ( "Labelled Image" )
imshow(labelled)
show()
relabeled = mahotas.labelled.borders(labelled)
print ( "Labels with border" )
imshow(relabelled)
show()
|
Output :
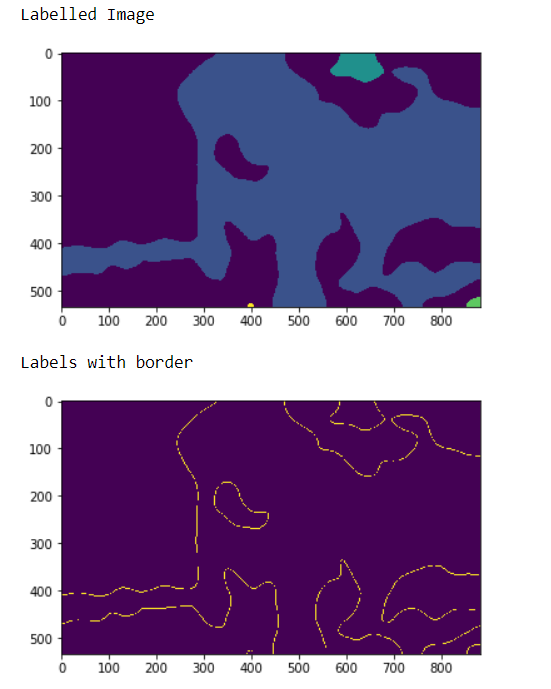
Share your thoughts in the comments
Please Login to comment...