How to choose the Right Data Analysis Technique?
Last Updated :
23 Apr, 2024
Suppose you have explored various types of data analysis techniques. In that case, we are sure you might scratched your head around the questions such as “How to choose an accurate data analytics method?”, or “How to choose the right data analysis technique?” To choose the right technique, you need to determine aspects like the type of your data, your business goal, resource availability, time limitations, audience, and more. Don’t worry, we got you covered.
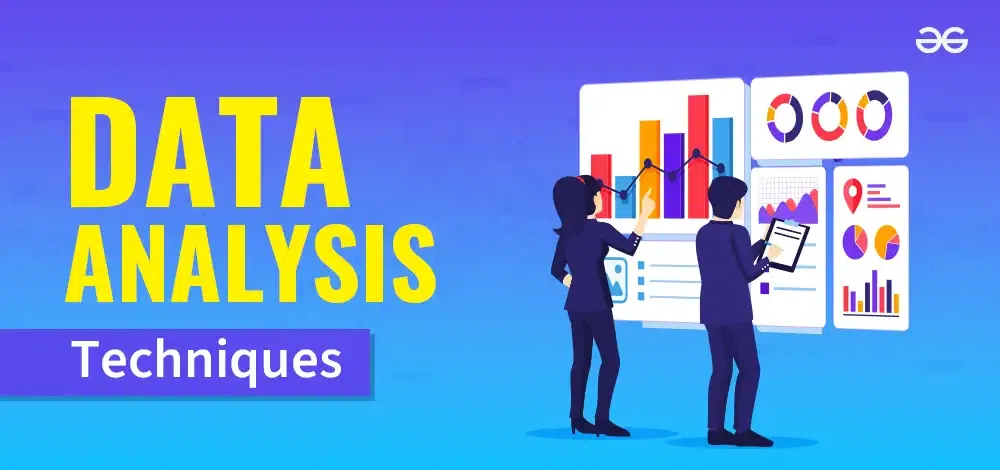
How to choose the Right Data Analysis Technique?
In this post, we will look into the characteristics of a good data analytics technique and how to use these characteristics to choose the right data analysis technique.
When to use which Data Analytics Technique?
Here’s a table summarizing when to use each data analytics technique:
Data Analytics Technique |
Description |
When to Use |
Descriptive Analytics |
Summarizing and interpreting historical data to identify patterns, trends, and relationships. |
– To understand historical trends and patterns – To provide context and background information |
Diagnostic Analytics |
Examining data to understand the cause of events and identify the factors that influence outcomes. |
– To investigate the reasons behind specific events or outcomes – To identify relationships between variables |
Predictive Analytics |
Using historical data to forecast future events and outcomes. |
– To forecast future trends and patterns – To make data-driven decisions and strategic planning |
Prescriptive Analytics |
Recommending actions and strategies to achieve specific objectives and optimize outcomes. |
– To optimize decision-making and resource allocation – To identify the best course of action to achieve desired outcomes |
Characterstics of a Good Data Analytics Technique
Data analytics is essential for businesses to make informed decisions in a constantly evolving market. However, specific challenges may arise during this procedure. We highlight common challenges and potential solutions to help make the right decision.
1. Handle Increasing Threats to Data Security
With an increasing volume of data, businesses can observe threats to data security, such as cyberattacks or data breaches.
Solution:
- Implement robust encryption protocols to ensure the sensitive data is secured even with the potential of unauthorized access.
- Conduct consistent security audits to identify potential vulnerabilities.
2. Effective Data Quality Management
Businesses need to implement strong measures to maintain the standard data quality and prevent the possibility of data degradation over a particular time.
Solution:
- Implement data regulations to boost accountability and data quality ownership across departments.
- Audits in specific intervals to check data quality procedures will ensure adherence to established quality standards.
3. Diverse Data Sources
Businesses often struggle with data integration from various sources, such as social media, customer databases, IoT services, etc. Here, the real challenge is streamlining standard data format, structure, originality, and more.
Solution:
- Deploy advanced data integration-based platforms to ensure compatibility with overall databases.
- Adopt or create a standard data format for storing quality data, and train your team to meet with the format for seamless integration.
4. Handling Large Data Volumes
As businesses collect data more than ever before, managing and processing these vast volumes can become overwhelming. This is particularly challenging when data analytics require real-time processing.
Solution:
- Invest in salable clous storage solutions that can grow with the needs of the business and handle large volumes of adtaa efficiency.
- Use big data processing frameworkssucha s Apache Hadoop or Apache Spark to efficiently process large datasets.
Guidelines for Choosing the Right Data Analytics Technique
- Define the Objective: The first step in choosing the right data analytics technique is to clearly define the objective of the analysis. Are you looking to understand historical trends, diagnose the factors influencing an outcome, predict future events, or prescribe the best course of action?
- Understand the Data: Before selecting a data analytics technique, it is crucial to understand the nature and characteristics of the data. Is the data structured or unstructured? What is the size of the dataset? Are there any missing values or outliers?
- Consider the Complexity: Consider the complexity of the analysis and the level of expertise required. Descriptive and diagnostic analytics techniques are generally simpler and require basic statistical and data visualization skills. In contrast, predictive and prescriptive analytics techniques are more complex and require advanced machine learning and optimization skills.
- Evaluate the Trade-offs: Each data analytics technique has its strengths and weaknesses. For example, while machine learning algorithms can provide accurate predictions, they may require a large amount of data and computational resources. On the other hand, statistical methods may be less accurate but more interpretable and easier to implement.
- Consider the Business Context: Finally, consider the business context and the specific requirements of the stakeholders. What are the key business questions and decisions that need to be addressed? What are the constraints and limitations?
Choosing the Right Data Analytics Technique
After exploring varied types of data analysis, the next common question arises: How to choose an accurate data analytics method? To choose the right technique, you need to determine aspects like the type of your data, your business goal, resource availability, time limitations, audience, and more.
- Define Your Problem: Before you begin with the data analysis process, every individual must identify or define the current issues or objectives for which you are considering to evaluate specific data.
- Collect Data: Next, consider collecting the data required for the detailed evaluation. Remember, these data should be accurate, relevant, and complete based on your defined objective.
- Choose The Right Technique: Based on your goal, consider evaluating data through predictive, diagnostic, time series, regr ession, quantity, quality, cohort analysis, and more. However, ensure the selected method solves, represents, represents insights, and aids in analyzing data accurately.
- Evaluate Your Data: Based on your selected analysis technique, analyze insightful data and validate the results to ensure the accuracy of your database.
- Interpret The Results: Dig deeper into the results of the data analysis process to validate, interpret, and compare insights. Make informed decisions and ensure relevance to the database for better operations.
Conclusion
Choosing the right data analysis technique is important to enhance the decision-making process and gain a competitive edge. Whether you offer services or products, every business requires organized data, and evaluating insights accurately can help identify varied aspects to drive growth, satisfy customers, strategize marketing efforts, and manage other operations.
Share your thoughts in the comments
Please Login to comment...