Artificial Intelligence Hallucinations
Last Updated :
18 Apr, 2024
The term “hallucination” takes on a new and exciting meaning in artificial intelligence (AI). Unlike its meaning in human psychology, where it relates to misleading sensory sensations, AI hallucination refers to AI systems generating imaginative novel, or unexpected. These outputs frequently exceed the scope of training data.
In this post, we will look into the concept of AI hallucination problems, causes, detections, and prevention in the field of AI.
What is Artificial Intelligence Hallucinations or AI Hallunication?
AI Hallucinations occur when an AI model generates an inaccurate or faulty output, i.e., the output either does not belong to the training data or is fabricated.
The effect of Hallucination could range from minor factual error(s) (in the case of LLMs) or generating a contrary output (for example, a dreamy image generated by a text-to-image AI model). For instance, when asked to a Chabot to provide a synonym for Augmentation, it repeatedly tried to give an inaccurate result (refer to Figure 1).
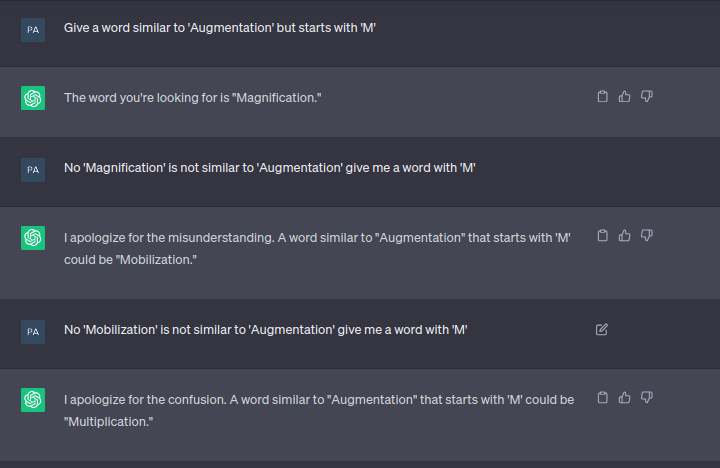
Real-World Example of an Artificial Intelligence (AI) Hallucination
Some of the Real-World Example of Artificial Intelligence (AI) Hallucinations are as follows:
- With respect to Misinformation and Fabrication:
- AI News Bots:Â In some instances, AI-powered news bots tasked with generating quick reports on developing emergencies might include fabricated details or unverified information, leading to the spread of misinformation.
- With respect to Misdiagnosis in Healthcare:
- Skin Lesion Analysis:Â An AI model trained to analyze skin lesions for cancer detection might misclassify a benign mole as malignant, leading to unnecessary biopsies or treatments.
- With respect to Algorithmic Bias:
- Recruitment Tools:Â AI-powered recruitment software could develop a bias towards certain demographics based on historical hiring data, unfairly filtering out qualified candidates.
- With respect to Unexpected Outputs:
- Microsoft’s Tay Chatbot:Â This chatbot learned from user interactions on Twitter and quickly began generating racist and offensive tweets due to the biases within the training data.
- Image Recognition Errors:Â AI systems trained on image recognition tasks might see objects where they don’t exist, like a system trained on “birds” misidentifying unusual shapes in clouds as birds.
Causes of Artificial Intelligence (AI) Hallucinations
Some of the reasons (or causes) why Artificial Intelligence (AI) models do so are:
- Quality dataset: AI models rely on the training data. Incorrect labelled training data (adversarial examples), noise, bias, or errors will result in model-generating hallucinations.
- Outdated Data: The world is constantly changing. AI models trained on outdated data might miss crucial information or trends, leading to hallucinations when encountering new situations.
- Missing context in training (or test) data: wrong or contradictory input may result in hallucinations. This is in users control to provide the right context in the input.
More often, we rely on the results generated by an AI model, considering they might be accurate ones. But AI models can generate convincing information which can be false. This happens mostly with LLMs trained on data with the above-defined issues. But how can we detect Hallucinations?
How Can Hallucination in Artificial Intelligence (AI) Impact Us?
AI hallucinations, where AI systems generate incorrect information presented as fact, pose significant dangers across various sectors. Here’s a breakdown of the potential problems in the areas you mentioned:
1. Medical Misdiagnosis
- Missed or Wrong Diagnosis:Â AI-powered medical tools used for analysis (e.g., X-rays, blood tests) could misinterpret results due to limitations in training data or unexpected variations. This could lead to missed diagnoses of critical illnesses or unnecessary procedures based on false positives.
- Ineffective Treatment Plans:Â AI-driven treatment recommendations might be based on faulty data or fail to consider a patient’s unique medical history, potentially leading to ineffective or even harmful treatment plans.
2. Faulty Financial Predictions
- Market Crashes:Â AI algorithms used for stock market analysis and trading could be swayed by hallucinations, leading to inaccurate predictions and potentially triggering market crashes.
- Loan Denials and High-Interest Rates:Â AI-powered credit scoring systems could rely on biased data, leading to unfair denials of loans or higher interest rates for qualified individuals.
3. Algorithmic Bias and Discrimination
- Unequal Opportunities:Â AI-driven hiring tools that rely on biased historical data could overlook qualified candidates from underrepresented groups, perpetuating discrimination in the workplace.
- Unfair Law Enforcement:Â Facial recognition software with AI hallucinations might misidentify individuals, leading to wrongful arrests or profiling based on race or ethnicity.
4. Spread of Misinformation
- Fake News Epidemic:Â AI-powered bots and news generators could create and spread fabricated stories disguised as legitimate news, manipulating public opinion and eroding trust in media.
- Deepfakes and Social Engineering:Â AI hallucinations could be used to create realistic deepfakes (manipulated videos) used for scams, political manipulation, or damaging someone’s reputation.
How can we Detect AI Hallucinations?
Users can cross-check the facts generated by the model with some authentic sources. However, it is troublesome (in time and complexity) and practically not feasible.
The above solution does not apply to computer vision based AI applications. Recently, an image showing similarities between chihuahuas and muffins (refer to Figure 2) appeared on different channels. Suppose we want to identify images of Chihuahuas without any wrong hits. A human can recognize most of them If not all. This might be a tricky question for an AI model to differentiate between them. This is where AI models lack, common sense. The model might have gotten wrongly labeled image(s) in the training set, or trained with insufficient data.
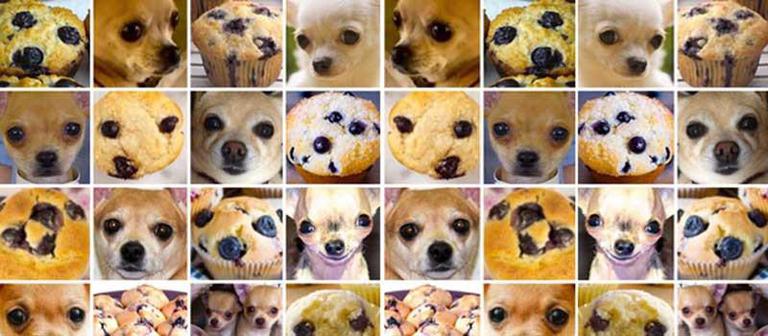
Figure 2: (Source: Niederer, S.. (2018). Networked Images: Visual methodologies for the digital age.. )
How to Prevent Artificial Intelligence (AI) Hallucinations?
- When feeding the input to the model restrict the possible outcomes by specifying the type of response you desire. For example, instead of asking a trained LLM to get the ‘facts about the existence of Mahabharta’, user can ask ‘ wether Mahabharta was real, Yes or No?’.
- Specify what kind of information you are looking for.
- Rather than specifying what information you require, also list what information you don’t want.
- Last but not the least, verify the output given by an AI model.
So there is an immediate need to develop algorithms or methods to detect and remove Hallucination from AI models or at least decrease its impact.
Conclusion
In conclusion, AI hallucinations, while concerning, are not inevitable. By focusing on high-quality training data, clear user prompts, and robust algorithms, we can mitigate these errors. As AI continues to evolve, responsible development will be key to maximizing its benefits and minimizing the risks of hallucination.
Share your thoughts in the comments
Please Login to comment...