Data Science in Sustainable Agriculture
Last Updated :
15 Mar, 2024
Data Science plays a crucial role in sustainable agriculture, offering modern solutions to enhance environmental stewardship and optimize agricultural practices. By utilizing advanced analytics and technology, farmers can make informed decisions based on data, leading to more efficient resource management and reduced environmental impact. This article explores How Data Science is changing farming for the better and How Sustainable agriculture is affected by Data Science.
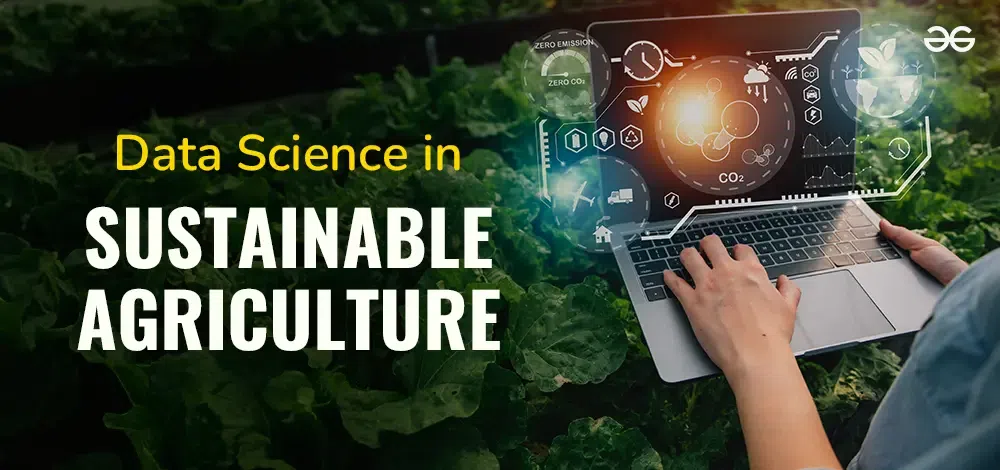
The intersection of Data Science and Sustainable Farming
The intersection of Data Science and sustainable farming occurs where data-driven insights intersect with agricultural practices. Farmers can integrate data from diverse sources, such as sensors, satellites, and weather stations, to monitor crop health, optimize irrigation, and minimize chemical usage. This integration facilitates more efficient and environmentally friendly farming techniques. Implementing data science in agriculture yields various benefits, including improved resource efficiency, enhanced productivity, and reduced environmental footprint. By analyzing extensive datasets, farmers can identify patterns, predict crop yields, and optimize inputs. This results in higher yields and healthier crops while simultaneously minimizing waste and resource consumption.
Real-World Examples of Data Science Applications
Real-world applications of Data Science in agriculture include precision farming and predictive analytics. Precision farming employs GPS-guided machinery and data analytics to optimize field-level management practices. Predictive analytics forecasts pest outbreaks and disease occurrences, enabling proactive measures to safeguard crops and minimize losses.
Tools and Technologies Powering Sustainable Agriculture
Several tools and technologies drive sustainable agriculture, including remote sensing, IoT sensors, machine learning algorithms, and cloud computing. Remote sensing technologies like drones and satellites provide high-resolution imagery for monitoring crop health, while IoT sensors gather data on soil moisture and nutrient levels. Machine learning algorithms analyze this data to offer actionable insights and recommendations.
Key Roles of Data Science in Agriculture
- Data Collection and Analysis: Data Science facilitates the collection and analysis of agricultural data from various sources, including sensors, satellites, and weather stations, enabling farmers to make informed decisions.
- Precision Farming: Data science enables precision farming by providing farmers with insights to optimize resource allocation, minimize waste, and enhance crop yield and quality.
- Predictive Analytics: Data Science enables predictive analytics to forecast crop diseases, pest infestations, and extreme weather events, empowering farmers to take proactive measures to protect their crops.
- Market Analysis: Data science aids in market analysis by analyzing pricing data, consumer preferences, and global market dynamics, allowing farmers to make strategic decisions regarding crop selection and production.
Applications of Data Science in Agriculture
- Crop monitoring and management
- Soil health assessment
- Weather prediction and management
- Pest and disease detection
- Yield prediction and optimization
- Supply chain management
- Market forecasting
- Farm automation and robotics
Real-World Examples Overflowing with Applications of Data Science in Agriculture
- Using satellite imagery and machine learning algorithms to monitor crop health and detect disease outbreaks.
- Employing IoT sensors to collect data on soil moisture and nutrient levels, optimizing irrigation and fertilization practices.
- Leveraging weather data and predictive analytics to anticipate extreme weather events and mitigate risks to crops.
- Implementing blockchain technology to track the provenance of agricultural products, ensuring transparency and traceability throughout the supply chain.
Challenges in Agricultural Data Science
- Data Privacy: Ensuring the security and privacy of agricultural data, particularly when sharing data with third parties or across platforms.
- Interoperability: Integrating data from diverse sources and systems to ensure compatibility and seamless communication between different technologies.
- Access to Technology: Providing access to data science tools and technologies, particularly for small-scale farmers in developing regions with limited resources and infrastructure.
- Data Quality: Ensuring the accuracy, reliability, and consistency of agricultural data collected from various sources, such as sensors and IoT devices.
Benefits of Data Science in Agriculture
- Improved resource efficiency : Data Science helps farmers use resources like water, fertilizer, and pesticides more efficiently. By analyzing data about the soil health, weather pattern and crop growth.
- Enhanced productivity and crop yield : Using data science tools, farmers can better understand their crops needs and able to optimize their growing conditions. This will helps plants and higher yields.
- Reduced environmental impact : By using tools more efficiently and making smarter decisions, data science helps reduce the environmental impact of agriculture.
- Minimized waste and resource usage : Data Science helps farmers identify areas where resources are being wasted and find ways to minimize waste.
- Enhanced decision-making and risk management: Data Science provides farmers with valuable insights and predictions that help them make better decisions and manage risks more effectively.
Future Trends in Data Science for Agriculture
Future trends in agricultural data science involve advanced technologies such as artificial intelligence, blockchain, and robotics. These technologies are poised to further enhance sustainability practices, improve supply chain transparency, and automate routine tasks, leading to more efficient and resilient agricultural systems.
Conclusion
Data science plays a pivotal role in transforming agriculture into a more sustainable and efficient industry. By harnessing the power of data analytics, machine learning, and predictive modeling, farmers can optimize resource management, increase productivity, and mitigate risks, ultimately contributing to a greener and more resilient food system for future generations.
Data Science in Sustainable Agriculture – FAQ’s
How does Data Science help farmers improve crop yield?
Data Science enables farmers to monitor crop health, optimize resource allocation, and mitigate risks, leading to higher yields and healthier crops.
What are some challenges in implementing Data Science in agriculture?
Challenges include data privacy concerns, interoperability issues, access to technology, and ensuring data quality and reliability.
What are the benefits of precision farming enabled by Data Science?
Precision farming optimizes resource use, minimizes environmental impact, and enhances productivity by tailoring actions to specific areas within fields based on data-driven insights.
Share your thoughts in the comments
Please Login to comment...