In the fast-paced and complex world of modern business, effective supply chain management is crucial for success. The traditional methods of managing supply chains are no longer sufficient to meet the demands of today’s dynamic market. This is where data science comes into play, revolutionizing Supply Chain Management and unlocking new levels of efficiency, transparency, and resilience.
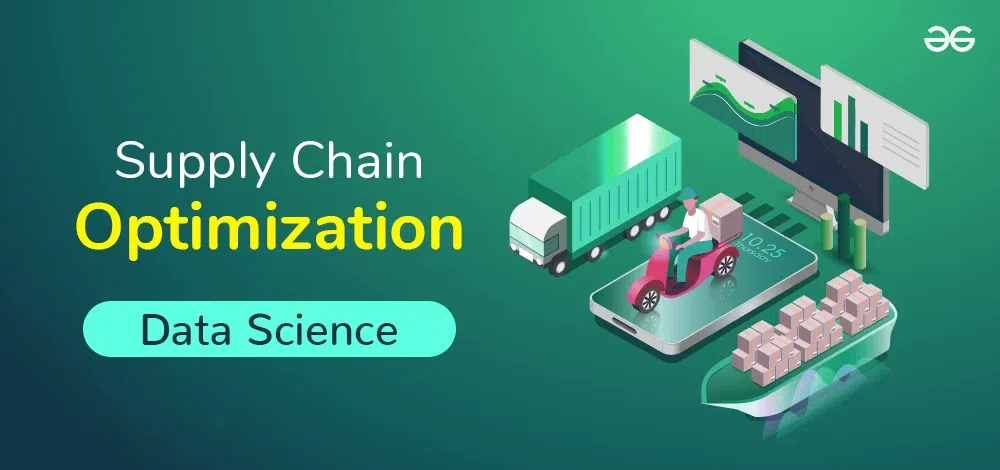
Supply Chain Optimization
In this article, we will explore How Data Science works in supply chain optimization and Various ways data science is reshaping the landscape of supply chain optimization.
Understanding Data Science in Supply Chain
Data science involves the extraction of valuable insights and knowledge from large sets of data. In the context of supply chains, data science integrates advanced analytics, machine learning, and artificial intelligence to enhance decision-making processes and streamline operations. By leveraging the power of data, businesses can gain a competitive edge and optimize their supply chain in various ways. Let’s delve into the ways data science is reshaping the landscape of supply chain optimization.
Demand Forecasting
One of the primary applications of data science in supply chain management is demand forecasting. Predicting consumer demand with accuracy allows organizations to optimize inventory levels, reduce excess stock, and prevent stockouts. Machine learning algorithms analyze historical sales data, market trends, and external factors to generate precise demand forecasts. This foresight empowers businesses to optimize inventory levels, reduce excess stock, and avert potential stockouts.
Inventory Management
Efficient inventory management is a key aspect of supply chain optimization. Data science helps organizations optimize stock levels by identifying patterns, seasonal trends, and fluctuations in demand. This not only minimizes holding costs but also ensures that products are readily available to meet customer demands.
Supplier Relationship Management
Data science plays a pivotal role in enhancing supplier relationship management by assessing supplier performance, identifying potential risks, and improving collaboration. Analyzing supplier data helps organizations make informed decisions about sourcing, negotiate better deals, and build more resilient supply chains.
Route Optimization
Transportation costs significantly impact supply chain efficiency. Data science algorithms can analyze historical shipping data, traffic patterns, and weather conditions to optimize transportation routes. The result is not only cost reduction but also improved delivery speed and reliability.
Real-time Visibility
Real-time data analytics provide end-to-end visibility across the supply chain. With the help of sensors, IoT devices, and advanced analytics, organizations can track the movement of goods, monitor inventory levels, and respond promptly to disruptions. This real-time visibility improves decision-making and minimizes the impact of unforeseen events.
Risk Management
Anticipating and mitigating risks is critical in supply chain management. Data science helps identify potential risks by analyzing historical data and external factors. By implementing predictive analytics, businesses can develop strategies to address disruptions such as natural disasters, geopolitical events, or supply chain interruptions.
Sustainable Practices
Data science can also contribute to sustainability in supply chain operations. By analyzing environmental impact data, organizations can identify opportunities to reduce carbon footprint, optimize packaging, and choose more eco-friendly transportation options. This not only aligns with corporate social responsibility goals but also meets the growing demand for sustainable and ethical business practices.
Collaboration and Communication
Effective collaboration is essential in complex supply chains involving multiple stakeholders. Data science facilitates communication and collaboration by providing a centralized platform for sharing real-time information. This enhances coordination between suppliers, manufacturers, and distributors, reducing delays and improving overall supply chain efficiency.
Personalized Customer Experiences
Data-driven insights enable organizations to understand customer preferences and behavior. This information can be leveraged to create personalized customer experiences, from tailored product recommendations to efficient order fulfillment. A personalized approach not only increases customer satisfaction but also strengthens brand loyalty.
Regulatory Compliance
Supply chain operations are often subject to various regulations and compliance standards. Data science helps organizations stay compliant by monitoring and analyzing relevant regulatory changes. This ensures that supply chain processes align with legal requirements, minimizing the risk of fines or disruptions due to non-compliance.
Dynamic Pricing Strategies
Data science enables organizations to implement dynamic pricing strategies based on real-time market conditions, demand fluctuations, and competitor pricing. This agile pricing approach optimizes revenue and profit margins while ensuring competitiveness in the market.
Post-event Analysis
After significant events, such as product launches or promotions, data science can be used to conduct post-event analysis. By examining the data generated during and after the event, organizations can gain insights into the effectiveness of their strategies and identify areas for improvement in future operations.
Employee Productivity and Well-being
Data science is not limited to optimizing external processes; it can also be applied to enhance internal operations. Analyzing employee productivity data can help organizations identify bottlenecks, streamline workflows, and improve overall efficiency. Furthermore, it can contribute to employee well-being by identifying factors that impact job satisfaction and engagement.
Technology Integration
Data science plays a crucial role in integrating emerging technologies into supply chain operations. This includes the integration of blockchain for transparent and secure transactions, the Internet of Things (IoT) for real-time tracking, and robotic process automation for streamlining repetitive tasks. These technologies, when integrated with data science, create a powerful ecosystem that fosters innovation and efficiency.
Continuous Improvement
Data-driven insights empower organizations to adopt a culture of continuous improvement. By analyzing performance metrics and key performance indicators (KPIs), businesses can identify areas for optimization, make data-driven decisions, and continually refine their supply chain processes.
Data Science Project Ideas for Supply Chain Optimization:
Predictive Maintenance: Utilize machine learning algorithms to predict equipment failures and maintenance needs in the supply chain. By analyzing sensor data and historical maintenance records, organizations can implement proactive maintenance strategies, reducing downtime and optimizing equipment lifespan.
- Optimized Procurement Strategy: Develop algorithms to optimize procurement decisions by considering factors such as supplier reliability, pricing trends, and quality metrics. This project aims to enhance supplier selection processes and negotiate favorable terms, ultimately reducing costs and improving supply chain efficiency.
- Supply Chain Network Design: Leverage data science techniques to optimize the design of supply chain networks. By analyzing factors like transportation costs, demand patterns, and facility capacities, organizations can redesign their networks to minimize transportation costs, improve service levels, and enhance overall efficiency.
- Demand Sensing and Shaping: Implement machine learning models to sense demand in real-time and shape it through targeted marketing campaigns or dynamic pricing strategies. By analyzing social media data, sales trends, and consumer behavior, organizations can anticipate demand shifts and adjust their strategies accordingly to maximize revenue and minimize stockouts.
- Warehouse Optimization: Develop algorithms to optimize warehouse operations, including inventory placement, picking routes, and storage allocation. By analyzing historical data on order volumes, SKU characteristics, and order processing times, organizations can streamline warehouse operations, reduce labor costs, and improve order fulfillment efficiency.
- Reverse Logistics Optimization: Apply data science techniques to optimize reverse logistics processes, including product returns, refurbishment, and recycling. By analyzing return patterns, product condition data, and repair costs, organizations can develop strategies to minimize returns, maximize asset recovery, and reduce waste in the supply chain.
Future of Data Science in Supply Chain
The future of data science in the supply chain is promising, with continued advancements in technology and analytics poised to drive further innovation. Some key trends and developments to watch for include:
- Advanced Predictive Analytics: The use of advanced predictive analytics techniques, such as deep learning and reinforcement learning, will enable more accurate demand forecasting, risk prediction, and decision optimization in the supply chain.
- Integration of IoT and Big Data: The integration of IoT devices and big data platforms will enable real-time monitoring and analysis of supply chain operations, providing greater visibility and agility to respond to changes and disruptions promptly.
- Blockchain for Supply Chain Transparency: Blockchain technology will play a significant role in enhancing supply chain transparency and traceability, enabling secure and immutable record-keeping of transactions and product movements throughout the supply chain.
- AI-driven Autonomous Supply Chains: AI-driven autonomous supply chains will become a reality, with intelligent algorithms orchestrating end-to-end supply chain processes, from demand forecasting to inventory management to transportation optimization, with minimal human intervention.
- Emphasis on Sustainability and Ethical Sourcing: Data science will be leveraged to drive sustainability and ethical sourcing initiatives, enabling organizations to trace the origins of products, assess environmental impacts, and optimize supply chain processes to minimize carbon footprint and promote ethical practices.
Conclusion
In conclusion, the integration of data science in supply chain management is a game-changer for businesses seeking to optimize their operations. From demand forecasting to real-time visibility and risk management, data science provides a comprehensive toolkit for improving efficiency, reducing costs, and enhancing overall supply chain resilience. As technology continues to advance, the role of data science in supply chain optimization will only become more pronounced, enabling businesses to stay competitive in an ever-evolving global marketplace.
The Role of Data Science in Supply Chain Optimization – FAQ’s
How can data science help in reducing supply chain costs?
Data science can identify cost-saving opportunities through optimized inventory management, route optimization, procurement strategies, and predictive maintenance, ultimately reducing overall supply chain costs.
What are the challenges of implementing data science in supply chain management?
Challenges include data quality issues, integration of disparate data sources, talent shortages in data science and analytics, and organizational resistance to change.
How does data science improve supply chain resilience?
Data science enables organizations to anticipate and mitigate risks by analyzing historical data and external factors, allowing for proactive risk management and resilience-building strategies.
What skills are required for a career in data science in the supply chain?
Skills include proficiency in programming languages (e.g., Python, R), statistical analysis, machine learning techniques, data visualization, and domain knowledge in supply chain management.
How can small businesses leverage data science in their supply chain operations?
Small businesses can start by implementing basic analytics tools to analyze sales data, optimize inventory levels, and improve demand forecasting. As they grow, they can explore more advanced data science techniques to further enhance their supply chain efficiency.
Share your thoughts in the comments
Please Login to comment...