Statistics is a branch of mathematics that deals with large amounts of data and the analysis of that data across various industries. Now, if you are looking for career opportunities as a data analyst or data scientist, then knowledge of statistics is very important. Because in most of these interviews, you will encounter statistical questions.
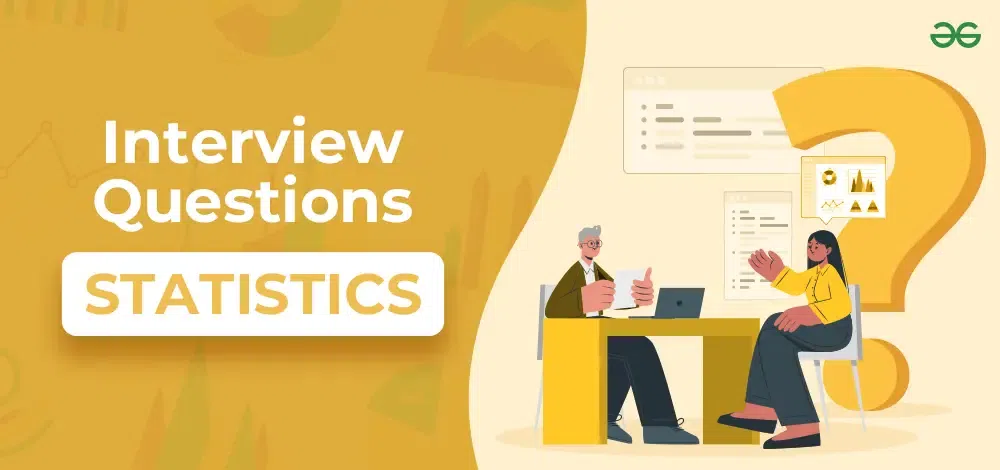
Hence, this blog post aims to delve into some of the most frequently asked interview questions for statistics. Moreover, by the end of this write-up, you will gain comprehensive insights at all levels, ranging from beginners to advanced statistical interview inquiries.
Short Overview of Statistics
As we aware, statistics is a branch of math that deals with the collection of data, data analysis, interpretation of data, and organization of data. Beciaclly, it is used in various fields, including business, economics, government, medicine, science, and the social sciences.
Now, if we talk about statistics types, then it has two types: descriptive statistics and inferential statistics. Descriptive statistics is used to summarize and describe data, while inferential statistics is used to draw conclusions about populations based on samples.
Statistics Interview Questions for Basic Level
1. What is the difference between Descriptive Statistics and Inferential Statistics?
|
These statistics are used to summarize the main features of a Data distribution
| These statistics are used to draw conclusions about a larger population by using sample data
|
Descriptive Statistics relies mostly on graphical representation to get meaningful information
| Inferential statistics relies on Probability Distribution and Mathematical formulas for meaningful conclusions
|
Mean, Median, Mode, Standard Deviation, Range, Histogram, Box Plot, etc.
| Hypothesis Test (t-test, z-test, Chi-square test), ANOVA, confidence interval, etc.
|
Descriptive Statistics does not involve any kind of assumptions about the population.
| Inferential Statistics is often associated with assumptions like Normality, Independence and Random Sampling.
|
Median salary in a university placement record
| The length of flippers for all the Penguins in the world
|
2. Difference between Population and Sample
|
Population is the entirety of the data that we are interested in.
| A sample is the subset of the data that we are interested in.
|
Population’s size is large enough to include every member of every group.
| Sample’s size is relatively smaller.
|
Population represents the complete data about the group we are interested in.
| Sample represents the subset of a population such that it has all the features of the entire population.
|
3. What is Random Sampling? What is its use?
Random Sampling is a process of selecting a subset from a population such that it ensures every member of a group in that population has equal chance of getting selected. Random Sampling is used to:
- it helps in making generalizations about the population
- it helps in reducing bias
- helps in extracting meaningful statistical inferences
4. What is Qualitative Data and Quantitative Data?
- Qualitative Data: Qualitative data cannot be explained in numbers. It is also called Categorical Data. It can be divided into groups and classes. Example: Gender, Color, Age category, etc.
- Quantitative Data: Quantitative data, on the other hand is, numerical data. This gives information about the measure of something and can be used in performing mathematical operations. Example: Sales of a car company, Bitcoin Value, etc.
5. What is meant by Probability Distribution?
Probability Distribution is a function that describes the likelihood of possible outcomes of a random event. That means it tells how likely it is for an event to occur and associates a probability to it.
6. What a nominal data and ordinal data?
- Nominal Data: It is a type of Qualitative Data which has no inherent order of rankings. That means this type of data does not have any numerical significance associated with them. Example: Types of Colors, Animal Species, etc.
- Ordinal Data: It is a type of qualitative data which has a defined order of ranking associated with it. Some group are given more preference over others. Example: Education Level, Likert Scale in Survey response, etc.
7. What is the Central Limit Theorem?
Central Limit Theorem states that:
” The sampling distribution of a sample means approaches normal distribution as the sample size increases irrespective of the shape of Population distribution.”
This theorem holds true for sample size greater than 30. For a Sampling Distribution that follows CLT:
- The sampling mean (
) is equal to population mean (
) - The standard deviation of sample distribution(
) is equal to standard deviation of population distribution (
) divided by square root of sample size ( n ).
8. Explain Skewness in Distribution. Why does it happen?
Skewness in distribution refers to the distortion in the data points of distribution, making the shape asymmetric. There are two types of skewness:
- Left/Negative Skewness: This is when the distribution shape is distorted towards left
- Right/Positive Skewness: This is when the distribution shape is distorted towards right
Skewness happens due to the presence of outliers. Outliers in a dataset decides the direction of skewness (positive or negative).
9. What is Normal Distribution? How is it different from a Uniform Distribution in Terms of Measure of Central Tendency?
|
It is a continuous probability distribution which is symmetric about the mean and having most data occurrence at mean.
| It is a continuous probability distribution where every value within a given range is equally likely to occur.
|

where,
= Normal probability density function
= Mean of the Normal Distribution
= Standard Deviation of Normal Distribution
| 
where,
a = minimum of the distribution
b = maximum of the distribution
x = mean of the distribution
|
It is a bell shaped curve
| It is a rectangular shaped curve
|
For Normal Distribution, mean = median = mode.
| For Uniform Distribution, mean = median = average of maximum and minimum in the distribution, and mode is undefined.
|
10. What is Binomial Distribution?
It is a Discrete probability distribution function that models the number of successes in fixed number of Bernoulli trials, where each trial is either success or failure. The Binomial Distribution function is given as:
, where
n = number of events conducted
p = Probability of the event happening
11. What is an Outlier?
An Outlier is a data point that is significantly different from other data points. Usually, Outliers are present in the extremes of the distribution and stand out as compared to their out data point counterparts.
12. What is the Measure of Center/ Measure of Central Tendency? Explain in brief about it.
Measure of Center/ Measure of Central Tendency is a part of statistics that talks about the “center” of a probability distribution (PD) /dataset. It uses 3 measures of “centers” for it, which are:
- Mean: The average of all the data points present in the dataset.
- Median: The middle data point of the sorted Dataset/PD.
- Mode: The data point which occurs most frequently in a dataset/PD.
13. What is the Measure of Dispersion? Explain in brief about it.
Measure of Dispersion/ Measure of Spread talks about how much distributed the data points are with respect to a single point. Usually, Measure of Dispersion is examined around the mean of the dataset. It explains how “spread out” the data points are around the mean. There are few metrics which tells about the dispersion of a dataset, among which the most used ones are:
- Range: The difference between the minimum and maximum value in the dataset
- Standard Deviation : it is the square root of variance.
- Variance: It is the average of the squared difference of each data point from the mean
14. What is complement rule in probability?
The Complement Rule in Probability states that:
“The probability an event does not occur is one minus the probability of the event occurring”
(Note: The complement Rule holds true for Independent events.)
15. What are Non probability sampling methods? Name a few of them.
Non Probability Sampling methods is based on personal preference of the concerned people. In this type of sampling method, usually sampling is done at the person’s own convenience. Some of the methods are:
- Convenience sample: A probability sampling method where the sample are chosen based on the ease to reach or contact.
- Snowball sample: its a method where initially approached people are given the task to further spread the recruitment of new people, like a snowball pattern.
16. What is Dependent Event and Independent Event?
|
Two events are dependent when the outcome of one event is influence by the outcome of another event.
| Two events are dependent when the outcome of one event does not affect the outcome of another event.
|

| 
|
drawing cards from a deck without replacement
| rolling a fair six-sided die
|
17. What is margin of error?
It is defined as the maximum expected difference between the population parameter and sample estimate.
18. What is the difference between Poisson Distribution and Bernoulli Distribution?
|
A discrete probability distribution used to explain the number of events/ occurrences occurring within a given time period.
| A discrete probability distribution used to model the likelihood of binomial (two) events which are success and failure
|

where,
X = random event
x = number of times the event occurs
e = Euler’s constant (2.718)
= average number of times an event occurs
| 
where, x= 0,1
X = random event
|
Used for independent events that occur at a constant rate
| The events here may or may not be independent.
|
Number of phone calls at a call center in an hour
| Success or failure in a product quality test
|
19. How to check if a Distribution is Normal?
There are many possible ways to check if a distribution is normal or not. Some of them are :
- Histogram: Plot the data distribution into a histogram, if the shape of plot is like a Bell, with highest frequency at the center and melts down on both sides, it is normally distributed.
- QQplot: Plot the distribution as a qqplot. If the data points mostly align along the straight diagonal line, it is normally distributed.
- Measure of Central tendency: For a normal distribution, mean = median = mode.
20. What is Measure of Position? How is it helpful in Descriptive Statistics?
Measure of position is used where we want to determine “where a specific data point or value falls” in a sample or distribution. It is sometimes necessary to know about the relative relation between two data points in terms of their position (like 75th percentile data, etc.). Some of the common measures of Position are:
- Percentile: It is a number below which a certain percentage of data points falls.
- Quartiles: Quartiles divides your data points into 4 quarters, one lower quarter, two middle quarter and one higher quarter.
- Five number summary: It includes the lowest value, the 3 interquartile ranges and the highest value.
21. What are the different types of Probability Sampling methods?
Probability sampling method is a technique of selecting a sample from a population such that each individual of the population has equal chance of getting selected into the sample. This is done by randomly selecting an individual. Some known types of Probability Sampling method are:
- Simple random sampling: In this, every member of a population is selected randomly and has an equal chance of being chosen
- Stratified random sampling: Here, you divide the population into groups and then randomly select a member from each group to be included in the sample.
- Cluster random sampling: in this, you create cluster from the whole population and randomly choose a whole cluster and each member of that cluster as your sample.
- Systematic random sampling: In this, you randomly choose a starting point from an ordered population and then choose a member at equal interval to be included in the sample.
22. What is Confidence level? How is Confidence level related to Width of Confidence interval?
Confidence Level: It is a statistical measure used to estimate the degree of confidence or certainty about an estimation process.
Confidence Interval: It is the range of values explaining the uncertainty surrounding an estimate.
In statistical analysis, The width confidence interval is directly proportional to the confidence level, i.e., as the confidence level increases, the width of confidence interval increases as well.
23. What are the different types of Probability Distributions?
There two types of probability distribution:
- Discrete probability distribution – It is the probability distribution associated with discrete random variable. A discrete random variable is variable that has countable number of possible values. There are several types of discrete probability distribution, out of which some commonly known are listed below:
- Uniform Distribution: It is done for distribution where the likelihood of each event occurring is the same.
- Binomial Distribution: It models the distribution where there are only two likely scenarios – success or failure. And those two events are mutually exclusive i.e., they cannot occur at the same time.
- Bernoulli Distribution: It is also the same as Binomial except this is for a single trial of event whereas Binomial is for repeated trials
- Poisson Distribution: This models the probability of a given number of events in a fixed time interval.
- Continuous Probability Distribution – The probability distribution associated with continuous value, i.e., it cannot be counted as it can take any value within a given range. Some known types of Continuous Probability Distribution are:
- Normal Distribution: It is the most common distribution characterized by its iconic bell shaped curve where the mean is at the center of the shape.
- Exponential Distribution: This describes the probability distribution of a Poisson process. A Poisson process determines the probability of random processes in a time period.
24. What is Hypothesis testing? Where do we use it?
Hypothesis testing is a fundamental part of inferential statistics which is used to help figure out whether an assumption about a population is true of not. This is done so by testing a sample from that particular population. The possible use cases for hypothesis testing are as follows:
- Inference : We can draw conclusions about a possible effect in a population
- Quality testing : Hypothesis testing allows us to evaluate product features and its quality
- Scientific research : We use Hypothesis testing for Scientific purposes where any assumption’s statistical significance is checked.
- Policy Evaluation : We can use hypothesis testing for different policy evaluations too,
25. What is Interquartile range?
Interquartile range (IQR) is the range between its first quartile (Q1 – representing 25% of data points) and third quartile (Q3 – representing 75% of the data points). This is used as a measure of dispersion and sometimes as measure of position as well.
26. What is Conditional Probability? How is it related to Bayes Theorem?
Conditional Probability: It is the probability of one event occurring when another (related to the first event) has already occurred. The formula for Conditional probability is:

Bayes Theorem: It states that for any two event A and B, the probability of A given B is equal to the probability of B given A multiplied by Probability of A divided by Probability of B. It is given as:

Bayes theorem is based on the principles of Conditional probability.
27. Explain the Joint and Marginal Probability.
Joint Probability : It is the probability of two or more events that are happening together. It can represented as the intersection between two or more probabilities.
Marginal Probability: It is the probability of a single random variable in isolation and it is not dependent on any other event.
28. What is the difference Between Probability Mass function and Probability Distribution Function?
|
PMF is used for discrete random variables. It assigns probabilities to individual values of a discrete random variable.
| PDF is used for continuous random variables. It represents the probability density of a continuous random variable over a range of values
|
Since, Discrete probability Distributions are represented as bars or spikes, The sum of all PMF values over all possible values equals 1.
| As Continuous Distributions are represented as a line or a curve, the area under that curve is equal to 1.
|
Tossing a fair coin
| Height of Giraffes
|
29. What is Z-score? How do you calculate it?
A Z-score or Standard score is a statistical measure which helps find out about how many standard deviation above or below the population mean is a data point situated. It is a form of Measure of Position. It is given as:
where,
Z = standard score
x = data point
= mean of the distribution
= standard deviation of the distribution.
To calculate Z-score:
- Subtract the mean of the population from the data point
- Then divide it by the standard deviation of the distribution
based on the result, a Z-score indicates:
- The data point is above the mean if Z-score is positive
- The data point is below the mean if Z-score is negative
- The data point is the mean if Z-score is 0
30. What is meant by standardization? Why do we sometimes standardize Normal Distribution?
Standardization refers to the process of transforming the data into a standard scale. Standardization is done by subtracting the mean and then dividing by standard deviation. It is done so that the data is centered around 0 and has the standard deviation of 1.
Standardization is sometimes implemented on Normal Distribution so that it is transformed into a more standardized scale. This is done so that:
- it is more comparable with respect to the original distribution which will further help in inferring how much the data point varies
- Allows various tests like Z-test and T-test, which largely assumes that the distribution is standardized.
- helps in Outlier Detection.
31. What are Axioms of Probability?
Axioms of Probability are foundations of probability used to assign it to an event. There are 3 axioms of probability which are:
- Probability of any event is a non-negative real number.
- Probability of the entire Sample Space is one
- If there are two mutually exclusive probabilities
and
, we can say that:

32. What is Empirical Rule?
Empirical rule states that for a normal distribution:
- Approximately 68% of the data falls within one standard deviation of the mean.
- Approximately 95% of the data falls within two standard deviations of the mean.
- Approximately 99.7% of the data falls within three standard deviations of the mean.
33. What is the difference Between Null Hypothesis and Alternate Hypothesis?
|
Null Hypothesis ( ) is a statement which is assumed to be true unless proven otherwise
| Alternate hypothesis ( ) is the contradicting statement which is proven true if there’s enough convincing evidence.
|
Represents the default or null assumption that you aim to test against.
| Represents the specific research question or hypothesis you want to investigate and support.
|
Assumes no relation, no effect
| Usually assumes a logical relation between. (<,>,etc)
|
34. What is Standard Error? How is it related to Variance of a Data Distribution?
Standard Error is defined as the amount of variability or uncertainty associated with Sample mean. It helps us understand how much the sample mean is likely to vary from the population mean if we were to take multiple random samples from the same population It is give as:

where,
= population standard deviation
n = the sample size
Standard error is directly proportional to Variance, i.e., as the Variance of a dataset increases, the standard error will increase too.
Statistics Interview Questions for Expert Level
35. What is Sampling Bias? How would you avoid bias in your dataset?
Sampling bias refers to distortion in the composition of a sample collected from a population which leads to results that do not accurately represent the population. To avoid having bias in your dataset, you can implement the following:
- Random Sampling method: This ensures that the sample is representative of the population by giving equal chance of selection for each member in that population.
- Stratified Sampling method: Another way where you divide the population based on certain groups and randomly select a member from the groups for the sample.
- Blinding: Blinding refers to the technique of keeping certain aspects of the experiment hidden from either researcher or the population. if it is hidden from both of them it is called Double Blinding.
36. What is meant by Type I and Type II error? How does it affect your decision making?
Type I error: Type I error occurs when a null hypothesis that is actually true is rejected. This means that the researcher believed that there was a significant effect/relationship when in actuality there wasn’t. In other words, it is a false positive result.
Type II error: Type II error occurs when a null hypothesis that is actually false is not rejected. This means that the researcher believed that there was no significant effect/relationship when in actuality there was. In other words, it is a false negative result.
37. What is Statistical Significance ? How does Hypothesis Testing help prove statistical Significance? Illustrate the steps in doing so.
Statistical significance assesses whether an observed effect or relationship in data is unlikely to have occurred by random chance. This is used in Hypothesis Testing for drawing conclusions about our Null Hypothesis. The results interpreted in your testing is said to be valid and statistically significant when your p-value is less than or equal to the predetermined Significance level (i.e., you reject the null hypothesis). In other case, the The Steps involved in doing so are:
- Formulate your null and alternate hypothesis
- Select a statistical significance value
- perform the test and find out the p-value
- Interpret the result
38. What is p-value? How is its value related to Confidence Interval?
p-value, short for probability value, is a statistical measure which is used to evaluate our hypothesis. If p-value is less than or equal to the pre-determined significance level, the null hypothesis is rejected and we conclude that there is relationship/effect between the variable. The smaller the p-value, the stronger the evidence against null hypothesis.
Confidence interval, on the other hand, is a range of values that is calculated from sample data and is used to estimate an unknown population parameter with certain level of confidence. Wider confidence intervals indicate greater uncertainty about the parameter estimate, while narrower intervals indicate greater precision.
Now, suppose you are checking your hypothesis for a value. If that value is not in the confidence interval range, you have an evidence now that you can use to reject the null hypothesis. This is similar to having a small p-value. In this way, you can use both p-value and Confidence interval to test your hypothesis.
39. What is the difference between t-score and z-score?
|
used when the standard deviation for population mean is unknown
| used when the standard deviation for population mean is known
|
follows a t-distribution with thicker tails.
| follows a standardized normal distribution with mean 0 and standard deviation 1.
|
Less precise than z-scores for small sample sizes due to the variability of the t-distribution.
| More precise for larger sample sizes as the z-distribution has a fixed shape regardless of sample size
|
40. What is One sample test? How is it different from Two Sample test? Give a scenario for each of these types of hypothesis testing?
|
Compare a single sample to a known or hypothesized population parameter. It only involves one sample
| Compare the means, variances, or proportions of two independent samples. It involves two independent samples
|
Null Hypothesis (H0): No significant difference from the population parameter. – Alternative Hypothesis (Ha): Significant difference from the population parameter.
| Null Hypothesis (H0): No significant difference between the two samples. – Alternative Hypothesis (Ha): Significant difference between the two samples.
|
Determine if the average height of a sample of students differs from the known population mean height
| Compare the effectiveness of two teaching methods by analyzing the test scores of students taught using each method
|
41. What are the assumptions made in One sample Z-test?
Following are the assumptions that are made in One sample Z-test:
- Normality : the true population distribution is normal.
- Independence : the observations in your data set are not correlated with each other, they should be independent.
- Known Standard Deviation : the true standard deviation of the population is known.
42. What is the difference between One tailed and Two tailed test?
|
Interested in only one direction of an effect or relationship (greater than or less than)
| Interested in both directions of an effect or relationship (not specifying greater or less than).
|
Null Hypothesis:
states that there is no effect/relationship
Alternate Hypothesis:
States that there is a definite relationship with a specific direction (e.g., population mean > a specific value or population mean < a specific value)
| Null Hypothesis:
states that there is no effect/relationship
Alternate Hypothesis:
States that there is a relationship , but not sure about the direction of it. (e.g., population mean ≠a specific value)
|
Critical region is located along the corresponding direction, in only one tail.
| Critical region is located on both the tails.
|
Testing whether a new drug increases patient recovery time
| Testing whether a new diet plan has an effect on weight loss
|
43. Why is t-test not used for Two sampling test of Proportions?
t-test is designed for normal continuous distributions or numerical data like means, score, etc. Proportions on the other hand represents categorical data. Categorical data cannot be plotted as a continuous distribution and do not follow normality. That is why t-test is not suitable for Proportions test. For that, we can use other tests like Z-test and Chi-square test.
44. What are the assumptions made in Chi-square test?
Following are the assumptions made in Chi-square test:
- Categorical : It is considered that the value is categorical in nature. (like gender, age, education level, etc.)
- Independent : It is considered that the value is independent
- Mutually exclusive : The samples/values should be mutually exclusive, i.e., they belong to only one category
45. What are the assumptions made in t-test hypothesis testing?
The following assumptions are made in a t-test:
- Normality : It is assumed that the data distribution is normal
- Independence : The observation of the two samples are different and not dependent
- Homogeneity of variance : Both have approximately same variance
- Random Sampling : The samples taken are randomly sampled.
46. What are different types of t-test?
The different types of t-test can be categorized into two:
- Based on samples:
- One sample t-test: t-test done where a single sample is used to compare with a known value like population mean. For e.g., Testing if the average score of students in a class differs significantly from the national average score
- Two sample t-test: It is done when comparing a value for two samples. For e.g., Comparing the test scores of students who received tutoring to those who did not to assess if tutoring has an effect.
- Based on Variance:
- Student t-test: When two samples have equal variance, we do student’s t test. For e.g., Comparing the average weights of two groups of mice, assuming that the variances in weights are similar in both groups.
- Welch t-test: When the two samples do not have equal variance, we use this method. For e.g., Comparing the test scores of students from two different schools where the variances in test scores are significantly different.
47. How can you determine if two samples have equal variance?
There are two possible methods to determine if two samples have equal variance:
- Variance rule of Thumb: if the ratio of larger variance to smaller variance is less than 4, it is assumed that the two samples have approximately equal variance.
- F-test: we can also use F-test to check whether the two variance are equal by using F-test, where we define our Null hypothesis to be both variance equal to each other.
48. What is F-test? What are the steps involved in an F-test?
F-test is a statistical test used to compare the variances of two or more groups or samples. This test helps in determining whether there is heteroscedasticity among two samples (they have different variance or not). To perform F-test, the following steps are taken:
- Formulate your Null and Alternate Hypothesis. Here, Null hypothesis is two samples have same variance and alternate hypothesis is they have different variance.
- Find the F-statistics which is calculated as :

- Calculate the degree of freedom for both the samples.
- Degree of freedom for numerator:
, where n1 is the sample size of sample 1 - Degree of freedom for denominator:
, where n2 is the sample size of sample 2
- For the degree of freedoms as calculated, find the F-value for your selected significance level.
- Compare the value with the F-statistics found at step 2.
- Interpret the results. If the F-value is less that the F-statistics, you reject the null hypothesis and conclude that there is heteroscedasticity between the two samples.
49. What is the usage of Box plot in Statistical Analysis?
Box plots are visualization plots that can be considered an important part of statistics. It is helpful in giving the Measure of Center, Measure of Dispersion and Measure of Position for the data distribution. The following are some aspects in which Box plots help:
- Finding out the median
- Detecting Outlier
- Identifying Data Skewness
- Visualizing Data distribution
50. You are given a task to measure the average height of all the trees in the world. How would you approach this problem with the help of statistics?
Lets approach the problem step by step:
- First we define the proper problem statement. Here, it is calculating the height of all the trees in the world.
- Create a sample of the whole population such that the sample is representative of the population
- Find out the descriptive statistics (i.e., measure of central tendency, measure of position and measure of dispersion) for the sample chosen.
- Formulate your hypothesis and choose a significance level. Here your hypothesis would be about the height of the trees in the sample.
- Find the p-value using appropriate test metrics
- compare the p-value and your significance level.
- Interpret the results from it.
51. Explain different types of Sampling Biases in statistics.
Biases refer deviations from the truth that can occur at various stages of the data collection, analysis, or interpretation process. Sampling Bias occurs when a certain group of individuals get preferred/favored to be included in the research, thus making it not representative of the population as whole.
There are 3 types of Sampling Bias in Statistics:
- Selection Bias : Selection bias occurs when the process of selecting individuals for a sample is not random
- Survivorship Bias : Survivorship bias occurs when the analysis or study focuses only on the individuals that have “survived” or made it through a selection or filtering process.
- Undercoverage bias : Undercoverage bias happens when certain segments or subgroups of the population are inadequately represented or excluded from the sample
Conclusion
Well, this is the end of this write-up. Here we have compiled the most-asked interview questions for statistics. To gain all the information about the interview and its related questions. Explore the whole blog post and collect all the ideas about it.
Share your thoughts in the comments
Please Login to comment...