It is critical to assess the practical importance or real-world impact of the findings in addition to the statistical significance of the findings when doing research or analyzing experimental data. The idea of effect magnitude is then relevant in this situation. Researchers can quantify and discuss the application of their findings by using the standardized measure of effect size to describe the size of the observed effect.
Estimating and understanding effect sizes rely heavily on effect size formulae. These equations are intended to condense the magnitude of differences between groups or the strength and direction of the link between variables. Researchers can increase the reproducibility of their findings, better understand the significance of their discoveries, and make wise judgments by measuring the effect size.
What is Effect Size?
The Concept of “effect Size ” in statistics measures the extent or strength of a relationship between two variables or the distinction between two groups. It indicates how much a particular therapy, intervention, or factor influences a desired result. The effect size is helpful because it enables academics and practitioners to understand the practical importance or real-world worth of their findings.
What is Effect Size Formula?
We use Cohen’s D method to compute how closely two variables are related:
Effect Size = (M1 – M2)/SD
where,
- M1 is the mean of the first population group,
- M2 is the mean of the second population group, and
- SD is the standard deviation.
Interpretation of Effect Size
Effect Sizes: Using standardized criteria, effect sizes can be divided into three categories: small, medium, and big. Numerous definitions of minor, medium, and large effects may be applicable depending on the circumstance and the research topic.
In addition to statistical significance, effect size also contributes to assessing the practical importance or worth of the results. A result may not always have a big impact size even if it is statistically significant, and the opposite is also true. Both statistical and practical significance must be considered while examining data.
Types of Effect Size
Effect Sizes are classified into so many types, The intention of each of which is to measure the relationship between two variables. The most used types of effect sizes are:
- Cohen’s d
- Pearson’s r
- Odds Ratio
- Phi Coefficient
Let’s discuss these types in detail as follows:
Cohen’s d
The standardized difference between two means is measured in this.
Cohen’s d = M1 – M2 / SD
Where,
- d is the effective size,
- M1 is the mean of the first population group,
- M2 is the mean of the second population group, and
- SD is the standard deviation.
- SD = √{(SD12 + SD22) ⁄ 2}
- Where SD1 and SD2 are the Standard deviation of first and second popilation group.
Pearson’s r
It Calculates how strongly two variables are correlated linearly.
Pearson’s r = cov(X,Y)/(SDx × SDy)
Where,
- cov(X,Y) is covarience between Xand Y,
- SDx is standard deviation of X, and
- SDy is standard deviation of Y.
Odds Ratio
This calculates the likelihood that an event will occur in one group vs another and is given as follows:
Odds Ratio = (a/b)/(c/d)
Where a,b,c, and d are frequencies of 2 × 2 table.
Phi Coefficient
This gauges how strongly two binary variables are related, and mathematically given by:
Phi Coefficient = (ad – bc) / √{(a+b)(c+d)(a+c)(b+d)}
Where a,b,c, and d are frequencies of 2 × 2 table.
Solved Examples of Effect Size Formula
Example 1: Two groups of students’ test results were compared in a study. Group B received an average score of 85 whereas Group A received an average score of 80. The pooled standard deviation was calculated as 10. Determine the effect size using Cohen’s d.
Answer:
Given: M1 = 80, M2 = 85, and SD = 10
Using the formula, d = (M1 – M2) / SD
⇒ d = (80 – 85) / 10
⇒ d = -5 / 10= -0.5
The effect size, in this case, is -0.5.
Example 2: A standardized anxiety scale was used in a study to compare the anxiety levels of two groups. Group X scored an average of 35, whereas Group Y scored an average of 40. The calculated pooled standard deviation was 6.5. Using Cohen’s d, determine the effect magnitude.
Answer:
Given: M1 = 35, M2 = 40, and SD = 6
Using the formula, d = (M1 – M2) / SD
⇒ d = (35 – 40) / 6.5
⇒ d = -5 / 6.5≈ -0.769
The effect size for this study is approximately -0.769.
Example 3: Consider two groups of students, Group A and Group B, with the following marks in a GFG contest. Determine the effect size using Cohen’s d.
Answer:
Step 1: Firstly we have to determine Mean of Two Groups
By adding up the marks for each group and dividing by the total number of students, we can first determine the mean for each group:
Group A’s mean (M1) is (78 + 82 + 85 + 90 + 73) / 5 = 81.6
Group B’s mean (M2) is (65 + 70 + 68 + 75 + 72) / 5 = 70
Step 2:Here we are suppose to calculate the standard deviation.
For Group A: Varience Of Group A: 33.840
Group A’s standard deviation (SDx) is 5.817.
For Group B: Varience Of Group B: 11.6
Group B’s standard deviation (SDy) is 3.406.
Step 3: calculating pooled standard deviation (SD)
Using the formula for the pooled standard deviation
SD = √{(SD12 + SD22) ⁄ 2}
⇒ SD ≈ 4.77
Step 4: Determining the effect size using Cohen’s d.
For Cohen’s d: Difference = M1 – M2 = 81.6 – 70 = 11.6
d = Difference / SD = 11.6 /4.7665 = 2.434
The effect size, for this comparison of Group A and Group B mathematics exam scores is roughly 2.434 .
Example 4: Determine Pearson’s r using the information below:
- X: 1, 2, 3, 4, 5
- Y: 2, 4, 6, 8, 10
Answer:
As 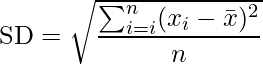
Thus, SDx = 3, and SDy = 6
and 
⇒ cov(X, Y) = 4
And, r = cov(X,Y)/(SDx × SDy)
⇒ r = 4/{3 × 6}
⇒ r = 4/18
⇒ r = 0.222 . . .
Example 5: For the following information, determine the odds ratio:
- Group 1: 20 victories, 30 failures
- Group 2: 20 failures, 30 triumphs
Answer:
Odd Ratios of information is; OR = (20/30) / (30/20) = 4/3
Example 6: Determine the phi coefficient for the given information:
a = 10, b = 20, c = 30, d = 40
Answer:
phi = (1040 – 2030) / sqrt((10 + 20 )(30 + 40 )(10 + 30)*(20 +))phi = 0.22.
FAQs on Effect Size Formula
Q1: Which Effect Size is Ideal?
Answer:
The study’s setting will determine a suitable effect size. Generally speaking, an impact size of 0.2 is regarded as minor, 0.5 as medium, and 0.8 or above as big.
Q2: How should Effect Size be Interpreted?
Answer:
The study’s context affects how effect size should be interpreted. A larger effect size typically denotes a stronger correlation between two variables or a greater gap between two groups.
Q3: What distinguishes Statistical Significance from Effect Size?
Answer:
Effect size calculates the size of the difference between two groups or the strength of the correlation between two variables, as opposed to statistical significance, which evaluates the likelihood that the observed difference or relationship is not the result of chance.
Q4: Can Effect Size Have a Bad Effect?
Answer:
Effect size can, in fact, be bad. The two groups being compared are more similar than distinct when the effect magnitude is negative.
Q5: What Benefit Does Employing Effect Size Provide?
Answer:
The advantage of using effect size is that it provides a reliable way to gauge how much two groups differ or how strongly two variables are associated. This makes it possible for researchers to evaluate the effects of different interventions or treatments in a variety of trials.
Q6: What drawback Exists with regard to Effect Size?
Answer:
The drawback of effect size is that it is unable to tell us whether the observed difference or association is statistically significant.
Share your thoughts in the comments
Please Login to comment...