Cluster Random Sampling, also known as Cluster Sampling, is a method where we start by picking a whole group and then study everyone within that group. It’s not like simple random sampling, where we select people one by one.
In cluster random sampling, these groups are what we focus on. This article takes you through cluster sampling, explaining what it is, the different types, how it works, and where it’s commonly used.
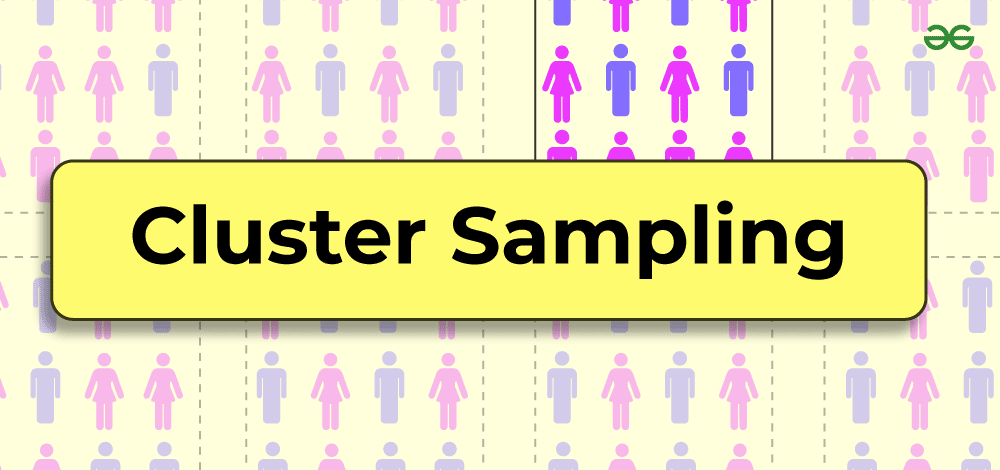
What is Random Sampling?
Random sampling is a way of picking a group of people or things from a bigger group so that everyone in the big group has the same chance of being picked. The main idea is to make sure the group we pick is a fair and unbiased representation of the big group.
Some of the main benefits of random sampling are:
- Less Biased: When everyone has the same chance, we make sure that personal likes or other things don’t mess up the results.
- More Accurate: Picking a fair group helps us get better and more dependable answers about the whole big group.
- Useful Math: Random sampling lets us use math to make smart guesses based on the data.
Read More, Random Sampling.
What is Cluster Sampling?
Cluster sampling is a method of sampling in statistics and research where the entire population is divided into smaller, distinct groups or clusters.
Instead of selecting individual members from the population, researchers randomly choose some of these clusters to include in the study. Then, all members within the selected clusters are surveyed or studied.
Examples of Cluster Random Sampling
Some common examples of Cluster Random Sampling are:
- Performance of Student Studying in Schools
- Evaluating Public Health Programs in Rural Areas
Let’s discuss these examples in details as follows:
Performance of Student Studying in Schools
Imagine assessing the reading skills of fifth-graders in a city. Directly visiting every student across all schools is time-consuming and expensive. Instead, we can:
- Divide the city into clusters: Group schools by district, geographic proximity, or similar demographics.
- Randomly select a few clusters: Choose a representative sample of schools from each group.
- Sample students within chosen clusters: Test a random sample of fifth-graders within each selected school.
By analyzing the reading skills of students in the chosen clusters, we can draw inferences about the reading proficiency of fifth-graders across the entire city with improved efficiency and cost-effectiveness.
Evaluating Public health programs in Rural areas:
Consider assessing the effectiveness of a new vaccination program in remote villages. Reaching every village for individual interviews might be challenging. Instead, we can opt for cluster sampling :
- Group villages into clusters: Based on geographic proximity, accessibility or population size, divide the villages into manageable groups.
- Randomly select a few clusters: Choose a representative sample of clusters from the whole set.
- Sample households within chosen clusters: Survey households within each selected cluster to gather data on vaccination coverage and program awareness.
By analyzing the data from sampled households in chosen clusters valuable insights into the program’s reach and impact across the larger rural population can be studied.
When to Use Cluster Random Sampling ?
Cluster Random Sampling should be used for when the below scenarios are :
- Widely spread out Population: Population spread over large geographic makes directly reaching individuals impractical.
- Limited resources: Saves time, budget and resources compared to individual sampling.
- Natural groupings exist: Preserves inherent population structure for accurate representation.
- Administrative convenience: Clusters align with existing data or organizational structures.
- Cost-effectiveness : Sampling clusters saves travel and logistical expenses.
The formula for cluster random sampling involves two stages.
First, calculate the average cluster size (ACS) which is the total number of elements divided by the total number of clusters. Then, multiply ACS with the number of clusters. Formula below:
n = ACS × Number of Clusters
Where n is the desired sample size. This formula ensures a representative and unbiased sample from the entire population through the randomized selection of clusters.
Types of Cluster Random Sampling
There are various types Cluster Random Sampling such as:
- Single-Stage Cluster Sampling
- Two-Stage Cluster Sampling
- Multi-Stage Cluster Sampling
- Systematic Cluster Sampling
Let’s dicuss about these types in detail as follows.
Single-Stage Cluster Sampling
In Single-Stage Cluster Sampling, the entire population is divided into clusters and a random sample of clusters is directly selected for inclusion in the sudy.
After selecting clusters, all individuals within the chosen clusters are included in the sample without further randomization at the individual level. It is relatively simple to implement but may not be as efficient as other methods.
Two-Stage Cluster Sampling
As the name suggests Two-Stage Cluster Sampling includes two stages. In the first stage, a random sample of clusters is selected and in second stage, a random sample of elements is chosen from within each selected cluster.
Two-stage cluster sampling is more efficient than single-stage cluster sampling and is commonly used when there is a hierarchy in the population.
Multi-Stage Cluster Sampling
Multi-stage cluster sampling involves more than two stages of sampling. It combines elements of various cluster sampling methods to create a complex sampling design.
This approach is used when there are multiple levels of clustering or when different methods are needed to ensure an efficient and representative sample.
Systematic Cluster Sampling
In systematic cluster sampling, clusters are arranged in a list or sequence, and a random starting point is selected. Then, clusters are sampled at regular intervals from the starting point until the desired sample size is achieved.
This method is straightforward and can be more efficient than simple random sampling when clusters are organized in a logical sequence.
Steps to Cluster Random Sampling
To perform cluster random sampling, we ca use the following process:
- Step 1: Clearly identify the entire group of interest.
- Step 2: Divide the population into distinct and mutually exclusive clusters.
- Step 3: Use a random sampling technique to choose specific clusters.
- Step 4: Include all individuals within the selected clusters in the sample.
- Step 5: Apply the study’s methodology to collect data from all elements within selected clusters.
- Step 6: Analyze collected data considering the cluster structure.
- Step 7: Communicate study findings including details about the cluster random sampling method used.
While performing cluster random sampling, please keep the following points in your mind.
- Aim for internal homogeneity within each selected cluster.
- Ensure randomness throughout the sampling process.
Cluster Random Sampling vs Simple Random Sampling
The key difference between Cluster Random Sampling and Simple Random Sampling are:
Aspects
|
Cluster Random Sampling
|
Simple Random Sampling
|
Definition
|
Divides the population into clusters and selects entire clusters for the sample.
|
Selects individual elements directly from the entire population with an equal chance of inclusion.
|
Process
|
Population divided into clusters; random clusters selected; all members in chosen clusters included.
|
Each individual in the population has an equal probability of being directly selected.
|
Advantages
|
- Cost-effective for large populations.
- Logistically convenient for naturally occurring clusters.
- Administrative efficiency in certain scenarios.
|
- Provides highly representative samples.
- Reduces bias and allows for precise statistical inferences.
- Simplifies data analysis.
|
Efficiency
|
More efficient for large populations or when natural groupings exist.
|
Ensures maximum representativeness.
|
Cost
|
Can be cost-effective, particularly when reaching individual elements is expensive.
|
Resource-intensive for large populations.
|
Advantages of Cluster Sampling
Common advantages of Cluster Random Sampling are:
- Cost-Effective: Cluster random sampling is often more cost-effective than other sampling methods especially when the population is large and geographically dispersed.
- Logistical Convenience: It is logistically convenient particularly when the population naturally forms clusters or groups.
- Administrative Efficiency: In studies involving administrative units like schools, districts or neighborhoods selecting entire clusters can be more administratively efficient compared to sampling individuals.
- Time-Efficient: Cluster sampling can save time, especially when resources are limited.
- Maintains Population Structure: When there are natural groupings or structures within the population, cluster random sampling helps maintain this structure in the sample providing a representative subset.
Disadvantages of Cluster Sampling
Common disadvantages of Cluster Random Sampling are:
- Less Precision: Cluster random sampling may lead to less precision compared to simple random sampling.
- Potential Bias: If there is heterogeneity within clusters, it might introduce bias in the sample.
- Complex Analysis: Analyzing data from cluster samples can be more complex than analyzing data from simple random samples. Special statistical techniques, such as accounting for cluster correlation, may be required.
- Cluster Size Variation: In some cases, clusters may vary in size leading to unequal representation. Larger clusters may contribute more significantly to the sample potentially skewing the results.
Conclusion
In conclusion, cluster random sampling is a valuable technique when dealing with large, geographically dispersed populations or naturally occurring groupings. This method offers cost and time efficiency making it logistically convenient, particularly in studies involving administrative units.
Read More,
Solved Examples on Cluster Random SamplingÂ
Example 1: Given Total Population: 800 households, Number of Clusters: 40 and Average Cluster Size (ACS) is 20, then determine the sample size using cluster random sampling.
Solution:
As we know, n = ACS × Number of clusters
⇒ n = 20 × 40
⇒ n = 800
Thus, the required sample size is 800 households.
Example 2: Given Schools in District: 60, Average Cluster Size: 15, Desired Sample Size: 300 then determine how many clusters should be randomly selected?
Solution:
Number of Clusters =Â Desired Sample Size/ ACS
⇒ Number of Clusters = 300 / 15
⇒ Number of Clusters =20
Thus, 20 schools were selected per clusters for the survey.
Example 3: Given hospitals in region is 25, desired sample size is 150 and average Cluster Size is 10 then determine the total number of elements in the sample.
Solution:
Hospitals in Region = 25
Desired Sample Size = 150
Average Cluster Size = 10
Thus, Total Sample Size = Desired Sample Size × ACS
⇒ Total Sample Size = 150 × 10
⇒ Total Sample Size = 1500
Thus, the sample consists of 1500 healthcare elements.
FAQs on Cluster Random Sampling
What is Cluster Random Sampling?
Cluster random sampling is a sampling method where a population is divided into clusters and entire clusters are randomly selected for analysis making it different from simple random sampling.
How is Cluster Size Determined?
The average cluster size (ACS) is calculated by dividing the total number of elements by the total number of clusters. This ACS is then used in the formula to determine the sample size.
What are some Types of Cluster Sampling?
Some of the comon types of cluster samping include Single-Stage, Two-Stage, Systematic, Multi-stage, etc.
What is 2 Stange Cluster Sampling?
Two-stage cluster sampling is a sampling technique that involves two stages of sampling i.e.,
- Stage 1: Selection of Random Cluster.
- Stage 2: Selection of individual from selected cluster.
Write One Example for Cluster Sampling.
In a study of student performance, random schools (clusters) were selected from each district, and all students within those schools were surveyed.
What are the Advantages of Cluster Sampling?
Advantages of Cluster Sampling includes:
- Cost-effective for large populations.
- Simplifies logistics and data collection.
- Preserves randomness when individual sampling is impractical.
- Useful for geographically dispersed populations.
What are Some Disadvantags of Cluster Sampling?
Disadvantages of Cluster Sampling includes:
- Increased sampling error compared to simple random sampling.
- Potential for cluster variability.
- Complex analysis and design.
- May require larger sample sizes.
Share your thoughts in the comments
Please Login to comment...