Chi-Square Test
Last Updated :
03 May, 2024
Chi-squared test indicates that there is a relationship between two entities. Handling data often involves testing hypotheses to extract useful information. In categorical analysis, chi-square tests are used to determine if observed patterns are likely to be purely random. The present manuscript expands upon chi-squared exam concepts, definitions, and procedures for doing them correctly.
What Is a Chi-Square Test?
Chi-squared test, or χ² test, indicates that there is a relationship between two entities. For example, it can be demonstrated when we look out for people’s favourite colours and their preference for ice cream. The test is instrumental in telling whether these two variables are associated with each other. For instance, it is possible that individuals who prefer the colour blue also tend to be in favour of chocolate ice cream. This test checks whether or not observed data fits those that would be expected assuming that association is absent at all, where there is a huge deviation.
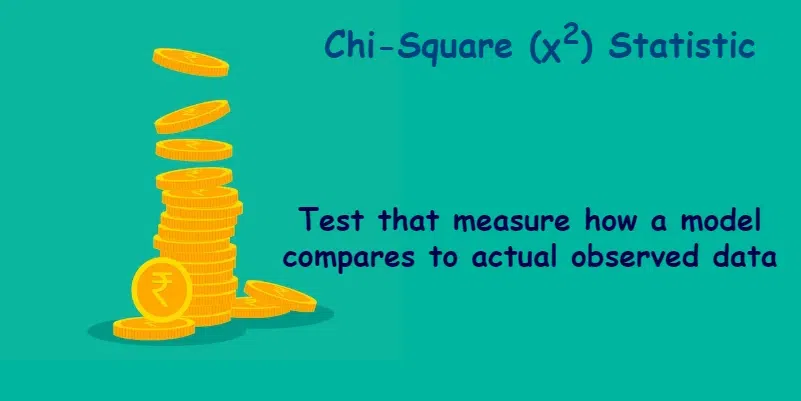
Chi-Square Test
When you toss a coin, you will expect to see heads or tails appearing in almost equal measure. For instance, in case you toss it several times and get many heads, then through the chi-square test we can conclude that the probability is less likely to be due to mere chance. Essentially, the chi-square test tackles two forms of figures which include observed frequencies (what you see happening) versus predicted frequencies(i.e., what should have occurred by chance). For simplicity, let us use an example of tossing coins where one would assume that getting either a head or a tail should occur on average fifty per cent of times each. It assists you with checking whether how the situation is playing out is a direct result of something genuine or simply irregular karma.
Why Chi-Square Tests Matter
Chi-square tests are important in various fields of study such as marketing, biology, medicine or even social sciences; that is why they are extremely valuable:
- Revealing Associations: Chi-square tests help researchers identify significant relationships between different categories, aiding in the understanding of causation and prediction.
- Validating Assumptions: Chi-square tests check if your observed data matches what you expected. This helps you know if your ideas are on track or if you need to reconsider them.
- Data-Driven Decisions: Chi-square tests validate our beliefs based on empirical evidence and boost confidence in our inferences.
(Oi – Ei)² / Ei = χ²
Symbols are broken down as follows:
- Σ (sigma): The symbol means sum, so each cell of your contingency table must be computed.
- Oi: This shorthand captures the idea of actual number of observations in a given cell of a contingency table, or what was actually counted.
- Ei: The number of times you would expect to see a particular result under conditions where we assume the hypothesis of no association (null hypothesis) is called as the expected frequency i.e. Ei.
- (Oi – Ei): The difference between the expected and actual frequencies is computed in this section of the formula.
Steps for Chi-Square Test
Various steps for chi-square test are added below:
Step 1: Define Hypothesis
- Null Hypothesis (H₀): The relationship between categorical variables is determined by the use of statistical analysis. This means the researcher assumes that there is no relationship between the two variables under study no matter the differences or patterns identified are as a result of random chance. Observing this hypothesis helps us protect our analysis from possible prejudices hence ensuring it is just.
- Alternative Hypothesis (H₁): The hypothesis suggests that there is a relation between the two categorical independent variables which are under study, therefore showing that there is an actual relationship instead of mere coincidence.
Step 2: Gather and Organize Data
Gather Information about the Two Category Variables:
Before performing a chi-square test, you should have on hand information about two categorical variables you wish to observe. As an example, in case one wishes look into how sex influences which type of ice-cream a person will choose- it would mean knowing the specific choice they would go for whether it is chocolate or strawberry among others besides their gender which implies both pieces of data have been collected already.
- Before conducting a chi-square test, it is necessary to get data on two categorical variables you want to analyze. For instance, if you are interested in exploring the relationship between gender and preferred ice cream flavors, then you must collect details on people’s sex (male or female) and their best flavors (e.g., chocolate, vanilla, strawberry).
- Once this information is collected, it can be inserted into a contingency table.
- When one is investigating the use of two related variables, it is necessary to use a contingency table to capture all combinations they can possibly be combined in. In this table, the values of one variable show up in the columns across, while values of another variable show up in rows. For instance, one can use it to determine how many females liked diet coke/vanilla flavored ice cream.
The hypothesis is that men prefer vanilla while women prefer chocolate. So we need to record how many have chosen vanilla among all male respondents versus the number who chose chocolate out of all female respondents.
Here’s an example of what a contingency table might look like:
|
Chocolate
|
Vanilla
|
Strawberry
|
Male
|
20
|
15
|
10
|
Female
|
25
|
20
|
30
|
In this table:
- Table contains two dimensions which are gender and ice cream flavors. The row headings are male and female categories respectively whereas column headings represent chocolate, vanilla and strawberry flavors. Each cell contains numerical counts for every combination of category. Conduct a chi-square test on this table to examine association between these two categorical variables.
Step 3: Calculate Expected Frequencies
- Get Predicted Frequency:In any specific cell, the expected frequency can be described as the number of occurrences which are expected if the two variables were independent.
- Expected Frequency Calculation: To compute the anticipated frequency of individual cells, one must use a method of comparison. This involves multiplying the sums of rows and columns in proportion, then dividing by the total number of observations in a table.
Step 4: Perform Chi-Square Test
Use Chi-Square Formula:
χ² = Σ (Oi – Ei)² / Ei
Step 5: Determine Degrees of Freedom (df)
df = (number of rows – 1) × (number of columns – 1)
Step 6: Find p-value
- One can use a chi-squar table to get the p-value for a particular chi-square statistic (χ²) with certain degrees of freedom (df) which was calculated. This table has chances of various values of the chi-square statistic in different degrees of freedom.
- If null hypothesis is correct then chi-square with its validity will be observed as p value. If it is assumed there is no correlation between the variables then the probability of this data set occurring given what we have seen becomes cleare
Step 7: Interpret Results
- If the p-value is less than a certain significance level (e.g., 0.05) then we reject the null hypothesis, which is commonly denoted by α. Thus it means that category variables highly correlate each other.
- When a p-value is above α it implies that we cannot reject the null hypothesis hence there is insufficient evidence for establishing the relationship between these variables.
Addressing Assumptions and Considerations
- Chi-square tests suppose that the observations are independent from one another; they are distinct.
- Each cell in the table should have a minimum of five values in it for better results. Otherwise, think about the Fisher’s exact test as an alternative measure if a table cell has less than five numbers in it.
- Chi-square tests do not indicate a causal relationship but they identify association between variables.
What are Categorical Variables?
- Categorical variables are like sorting things into different groups. But instead of using numbers, we’re talking about categories or labels. For example, colors, types of fruit, or types of cars are all categorical variables.
- They’re termed as “categorical” simply because bit by bit they segment things like “red,” “green” or “blue” into separate clusters. Unlike height or weight whose measurements are contiguous, categorical data has definite options without numerical order between them. That is why if you ask whether someone prefers apples to oranges then it means that the person is discussing categorical data
Characteristics of Categorical Variables
- Distinct Groups: Categorical variables put things into different groups that don’t overlap. For example, when we talk about hair color, someone can be a redhead, black-haired, blonde, or brunette. Each person falls into just one of these groups.
- Non-Numerical:There is no hierarchy in categorical terms for these are just names and not actions hence its futility to compare like blondes are better than brunettes based on it; referring blondes as bad women due to their hair types will be unfair as it may not make any sense referring such attributions with regards to colours either feminist perspective could be used but the simplest explanation remains that ’they are merely dissimilar.
- Limited Options: Categorical variables are characterized by a fixed number of possibilities. One may have such choices as red, blonde, brown, black hair color. The number of categories may fluctuate, but they all remain distinct and bounded in scope.
Goodness-Of-Fit
A goodness-of-fit test is used to determine whether or not a model or hypothesis being utilized is consistent with collected data type. Suppose you were to come up with a hypothesis such as: ‘It is likely that humans who live in urban areas are taller than those from rural areas’. After collecting data on the heights of people and comparing it with your hypothesis’ prediction, if there is close agreement between the two then one has grounds for believing that these predictions are correct. But if such agreement does not exist, then perhaps one has to rethink on his/her hypothesis. Thus, the goodness of fit test helps us.
Key Aspects of a Goodness-of-Fit Test
1. Purpose: The aim is to check if a guessed distribution fits well with the data we have.
2. Data Requirements: It can be used with both continuous and categorical data, among other forms of data.
3. Common Applications:
- The aim of this procedure is to examine if a set of figures is taken from a standard random distribution.
- In addition to this, it will juxtapose the actual occurrence of stuff with what we expect more times than not – a good example is making comparisons using chi-square test.
- It evaluates the degree of correspondence between a series of points displayed as dots on a graph, with one straight line or curved shape – this happens during regression analysis.
4. Benefits:
- Gives us a way to check if our ideas match up with real data.
- Helps us spot any weird or unusual data that might cause problems.
5. Limitations:
- Various tests are more effective in various situations.
- These results can be altered by the test type and volume of data points as well.
Types of Goodness-of-Fit Tests
- Chi-Square Test: It is mainly used for categorical data and helps in comparing the observed frequencies of classes with their expected frequencies based on a theoretical model.
- Kolmogorov-Smirnov Test: Anderson-Darling test checks how things are distributed (cumulatively) against an expected model’s distribution without having any assumptions about that model’s form. It applies to discrete as well as continuous data types. You can think of it as a way to tell if a cake recipe was followed or not from how the cake spreads out as it cooks. This makes it important in social science research, as we often lack grounded informations on which alternative hypotheses may be preferred or disconfirmed.
- Anderson-Darling Test: A nonparametric test that is specifically concerned with the weighted absolute differences between cumulative observed and calculated distributions. When we are talking about the discrepancies in the tails of distributions, it usually is more sensitive than Kolmogorov-Smirnov’s test. It examines the extent of these disparities as renewed emphasis on tails. This is much more efficient at finding outages in the tails punansa suuntaajiin.
Solved Examples on Chi-Square Test
Example 1: A study investigates the relationship between eye color (blue, brown, green) and hair color (blonde, brunette, Redhead) . The following data is collected:
Eye Color
|
Blonde
|
Brunette
|
Redhead
|
Total
|
Blue
|
35
|
52.5
|
12.5
|
100
|
Brown
|
28.1
|
42.1
|
9.8
|
80
|
Green
|
6.9
|
10.4
|
2.7
|
20
|
Solution:
Calculate the chi-square value for each cell in the contingency table using the formula
χ² = (Oi – Ei)² / Ei
For instance, consider someone with brown hair and blue eyes:
χ² = (15 – 28.1)² / 28.1 ≈ 6.07.
To complete the total chi-square statistic, find each cell’s chi-squared value and sum them up across all the nine cells in the table.
Degrees of Freedom (df):
df = (number of rows – 1) × (number of columns – 1)
df = (3 – 1) × (3 – 1)
df = 2 × 2 = 4
Finding p-value:
You may reference a chi-square distribution table to get an estimated chi-square stat of (χ²) using the appropriate degrees of freedom. Look for the closest value and its corresponding p-value since most tables do not show precise numbers.
If your Chi-square value was 20.5, you would observe that the nearest number in the table for df = 4 is 14.88 with a p-value in 0.005; an illustration is.
Interpreting Results:
- Selecting a level of significance (α = 0.05 is common)or than if the null hypothesis holds, the probability of either rejecting it at all is limited (Type I error).
- Compare the alpha value and p-value.
- When the p-value is less than the significance level, which in this case is written as p-value < 0.05, we can reject the null hypothesis. There is sufficient evidence to say that hair and eye color are related in one direction according to statistical terms. If the p-value is greater than the significance level it means that we cannot reject the null hypothesis therefore p-value > 0.05.
- Based on the data at hand, we cannot say that there is a statistically significant correlation between eye and hair colors.
Example 2: 100 flips of a coin are performed. The coin is fair, with an equal chance of heads and tails, according to the null hypothesis. 55 heads and 45 tails are the observed findings.
Solution:
Let’s imagine a coin. this coin has two sides, one which has tails and the other that has heads on them, when flipping this coin there is a 50/50 chance of obtaining either outcome.
This is why most of us would like characteristic information about it because then they predict the result based on their prior knowledge or experiences even before actually doing so- such things include whether the person who tossing has been motivated enough as well as what he/she hopes will happen next if head or tail shows up. However, there are times when people make different decisions in a hurry without thinking about future consequences and that could be possible when dealing with rare coin.
Afterwards, the anticipated values will be juxtaposed with the ones from making several flips at the dice case. Dissimilar results from those that would be attributable to mere chance may perhaps indicate that this might otherwise.
FAQs on Chi-Square Test
What is a chi-square test used for?
Chi-square test is a statistical test used to compare observed results with expected results.
What is p-value in a chi-square test?
P-value is the area under the density curve of this chi-square distribution to the right of the value of the test statistic.
What are limitations of chi-square tests?
Chi-square tests can only be applied with categorical variables. They need a large enough sample to get accurate results. If the cell numbers are below 5, findings can be unreliable. Independent observation is presumed for chi-square tests. Chi-square tests do not show how strong the association is or in what direction it goes. When dealing with relationships involving continuous variables, they find it unsuitable for themselves, so they opt out of it instead.
What if expected frequencies are low?
If you have a small group or expect only a few things to happen, think about using Fisher’s exact test.
How to choose the appropriate level of significance (α)?
Choice of α is based on the tradeoff between minimizing Type I error (rejecting a true null hypothesis) and Type II error (failing to reject a false null hypothesis). Here are some rules of thumb: Typical choices include α = 0.05 (5%) or α = 0.01 (1%). The consequence of having a lower α is that researchers need a stronger statistical signal in order to reject the null hypothesis, which makes it more serious.
Share your thoughts in the comments
Please Login to comment...