It goes without saying that in general a search engine responds to a given query with a ranked list of relevant documents.The purpose of this article is to describe a first approach to finding relevant documents with respect to a given query. In the Vector Space Model (VSM), each document or query is a N-dimensional vector where N is the number of distinct terms over all the documents and queries.The i-th index of a vector contains the score of the i-th term for that vector.
The main score functions are based on: Term-Frequency (tf) and Inverse-Document-Frequency(idf).
Term-Frequency and Inverse-Document Frequency –
The Term-Frequency (
) is computed with respect to the i-th term and j-th document :

where

are the occurrences of the i-th term in the j-th document.
The idea is that if a document has multiple receptions of given terms, it will probably deals with that argument.
The Inverse-Document-Frequency (

) takes into consideration the i-th terms and all the documents in the collection :
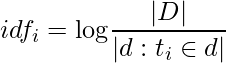
The intuition is that rare terms are more important that common ones : if a term is present only in a document it can mean that term characterizes that document.
The final score

for the i-th term in the j-th document consists of a simple multiplication :

. Since a document/query contains only a subset of all the distinct terms in the collection, the term frequency can be zero for a big number of terms : this means a sparse vector representation is needed to optimize the space requirements.
Cosine Similarity – In order to compute the similarity between two vectors : a, b (document/query but also document/document), the cosine similarity is used :
(1) 
This formula computes the cosine of the angle described by the two normalized vectors : if the vectors are close, the angle is small and the relevance is high.
It can be shown the cosine similarity is the same of the Euclidean distance under the assumption of vector normalization.
Improvements – There is a subtle problem with the vector normalization: short document that talks about a single topic can be favored at the expenses of long document that deals with more topics because the normalization does not take into consideration the length of a document.
The idea of pivoted normalization is to make document shorter than an empirical value ( pivoted length :

) less relevant and document longer more relevant as shown in the following image: Pivoted Normalization
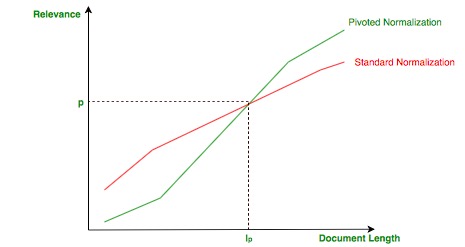
A big issue that it is not taken into consideration in the VSM are the synonyms : there is no semantic relatedness between terms since it is not captured neither by the term frequency nor the inverse document frequency. In order to solve this problems the Generalized Vector Space Model(GVSM) has been introduced.
The Vector Space Model (VSM) is a widely used information retrieval model that represents documents as vectors in a high-dimensional space, where each dimension corresponds to a term in the vocabulary. The VSM is based on the assumption that the meaning of a document can be inferred from the distribution of its terms, and that documents with similar content will have similar term distributions.
To apply the VSM, first a collection of documents is preprocessed by tokenizing, stemming, and removing stop words. Then, a term-document matrix is constructed, where each row represents a term and each column represents a document. The matrix contains the frequency of each term in each document, or some variant of it (e.g., term frequency-inverse document frequency, TF-IDF).
The query is also preprocessed and represented as a vector in the same space as the documents. Then, a similarity score is computed between the query vector and each document vector using a cosine similarity measure. Documents are ranked based on their similarity score to the query, and the top-ranked documents are returned as the search results.
The VSM has many advantages, such as its simplicity, effectiveness, and ability to handle large collections of documents. However, it also has some limitations, such as the “bag of words” assumption, which ignores word order and context, and the problem of term sparsity, where many terms occur in only a few documents. These limitations can be addressed using more sophisticated models, such as probabilistic models or neural models, that take into account the semantic relationships between words and documents.
Advantages:
Access to vast amounts of information: WIR provides access to a vast amount of information available on the internet, making it a valuable resource for research, decision-making, and entertainment.
Easy to use: WIR is user-friendly, with simple and intuitive search interfaces that allow users to enter keywords and retrieve relevant information quickly.
Customizable: WIR allows users to customize their search results by using filters, sorting options, and other features to refine their search criteria.
Speed: WIR provides rapid search results, with most queries being answered in seconds or less.
Disadvantages:
Quality of information: The quality of information retrieved by WIR can vary greatly, with some sources being unreliable, outdated, or biased.
Privacy concerns: WIR raises privacy concerns, as search engines and websites may collect personal information about users, such as their search history and online behavior.
Over-reliance on algorithms: WIR relies heavily on algorithms, which may not always produce accurate results or may be susceptible to manipulation.
Search overload: With the vast amount of information available on the internet, WIR can be overwhelming, leading to information overload and difficulty in finding the most relevant information.
Share your thoughts in the comments
Please Login to comment...