A Continuous Probability Distribution is a statistical concept that describes the probability distribution of a continuous random variable. It specifies the probabilities associated with various outcomes or values that the random variable can take within a specified range.
In this article, we’ll look into Real Life Applications of Continuous Probability Distribution.
What is Continuous Probability Distribution?
A continuous distribution is a statistical distribution wherein the possible values of the random variable constitute a continuous range. This implies that the variable can be any value within the specified range and not necessarily restricted to the discrete individual values.
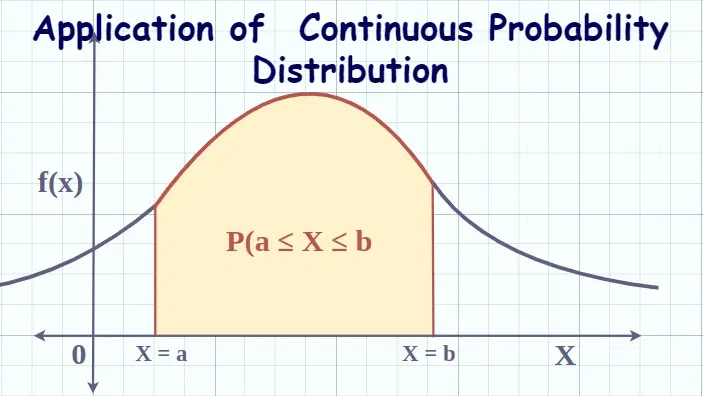
Distributions that are continuous are commonly defined by probability density functions (PDFs), which express the probability of the variable assuming a given value within its range.
Key Points
- Continuous Random Variable: In a continuous probability distribution, the random variable can take on any value within a specified interval or range. This means that the variable can theoretically assume an infinite number of values within that range.
- Probability Density Function (PDF): Persistent probabilities are frequently modeled using a probability density function (PDF), which is a function that shows the relative probability of the random variable obtaining different values across the domain of interest. The area under the curve of PDF of a distribution between the needed interval implies that a random variable has that value with the biggest probability.
- No Individual Probabilities: In contrast with discrete probability distribution that assigns a probability to each individual value, continuous probability distribution will specify the probability for each interval of the values. This is because there is no definite probability assigned to any single value of continuous random variable since as in the case of discrete probabilities, they assume infinite number of possible values.
Examples: Normal distribution, uniform distribution, exponential distribution, as well as the beta distribution are famous examples of continuous probability distributions, and it is definitely not an exhaustive list. It can be said that every distribution has its own PDF that shows how the probability density for each random variable would be distributed.
Continuous Probability Distribution Formulas
Some fundamental formulas used in probability theory and statistics to characterize continuous probability distributions and analyze their properties depending on the distribution being considered are:
1. Probability Density Function (PDF)
The probability density function f(x)describes the probability distribution of a continuous random variable X. It satisfies the following properties:
- f(x) ≥ 0 for all x in the range of X.
- ∫∞-∞ f(x)dx = 1 (total area under the curve equals 1).
Depending on the specific distribution, the PDF formula varies.
For example:
- Normal Distribution: f(x) = (1/√2πσ 2).[Tex]\bold{e^{-\frac{xμ^2}{2σ^2}}}[/Tex]
- Uniform distribution: f(x) = 1/(b-a) for a <= x <= b
- Exponential Distribution: f(x) = λe-λx for x >-= 0
- Beta Distribution: f(x) = xα-1 (1−x)β-1/B(α,β) for 0 <= x <= 1
2. Cumulative Distribution Function (CDF)
- Cumulative distribution function F(x)gives the probability that a random variable X takes on a value less than or equal to x.
- It is the integral of the PDF up to a certain value of x: F(x) = ∫x-∞ f(t)dt
- CDF is often provided as part of the definition of specific distributions.
3. Mean (Expected Value)
- Mean μ of a continuous random variable X is its average value, and it is given by: μ = ∫-∞∞x⋅f(x)dx
- Alternatively, for a function g(X), the mean is E[g(X)] = ∫-∞∞ g(x)⋅f(x)dx.
4. Variance
- Variance σ 2 of a continuous random variable X measures the spread of its distribution, and it is given by:
- σ2 = ∫-∞∞ (x−μ)2⋅f(x)dx
- Standard deviation σ is the square root of the variance.
Applications of Continuous Probability Distribution
Continuous Probability Distribution functions have various applications in real life that includes:
From finance to physics, engineering to health care, as we focus on continuous probability distributions, we begin to uncover its contributions across a wide variety of fields.
- Finance and Economics
- Physics and Engineering
- Demography and Social Sciences
- Healthcare and Biology
- Quality Control and Manufacturing
Finance and Economics
Continuous probability distributions play a significant role in finance and economics, providing essential tools for modeling uncertainty, risk, and various financial phenomena. Here are some key applications:
In Stock Prices and Returns
- Relative variability of both stocks prices and stock returns is typically depicted by a log-normal distribution or, hence, continuous distributions. Log-normal distribution is indeed the best tool for capturing the volatility of pricing in financial markets and has the feature of being both continuous and volatile.
- Models of the type of the Black-Scholes model for option pricing where the stock prices follows a log-normal distribution that is a widely use assumptions for the pricing of the options and then the evaluation the strategy of investments.
In Interest Rate Modeling
- Continuous probability distributions are normally employed to formulate models of interest rates over time, when time is considered as a variable. For example, Vasicek model or Cox-Ingersoll-Ross (CIR) model are today well-known in the field of interest rate modeling.
- In these models the interest rates are described as stochastic continuous processes, which makes possible their simulation from the viewpoint of economists and financial experts. These analyses will allow to investigate and predict interest rate scenarios and assess their effect to bond prices, derivative securities and other financial instruments.
In Portfolio Management and Risk Analysis
- Continuous distributions are key tool in portfolio management and it’s risk analysis as they can be used to approximate the distribution of portfolio returns and evaluate overall portfolio risk.
- Techniques like Value-at-Risk (VaR) and Conditional Value-at-Risk (CVaR) provide confidence limits with which the future probability distributions are based in order to estimate the potential losses of a portfolio through changing confidence levels.
In Financial Derivatives Pricing
- Continuous probability distributions are a general case that’s extensively applied to price financial derivatives, including options, futures, and swaps. Derivative pricing models like Black-Scholes model that take specific continuous distribution do the calculation of reasonable underpricing of these instruments.
- Such a model encourages investors and financial institutions to trade in derivatives successfully with higher transaction efficiency, therefore providing an opportunity for risk management and corporate hedging strategy.
In Credit Risk Modeling
- An assessment of the risk of default and the estimation of loss due to credit portfolio is facilitated by the use of continuous distributions in credit risk modeling.
- In their turn the Merton model and the continuous probability credit risk model are implemented in order to characterize the borrowers’ credit value and the probability of default occurrence.
Physics and Engineering
Continuous probability distributions find extensive applications in physics and engineering. Here are some key areas where they are applied:
In Signal Processing
For use in signal processes, continuous probability distributions are used to model the noise in signals. The Gaussian distribution, which has a wide usage as a basement for the random noise features of electrical signals, is a popular choice for that. The filter model engineered by the engineers employ this model so heavily in reacting noises and designing the best signal filtering algorithms and processing techniques.
In Measurement and Instrumentation
Among them, any continuous distributions as normal distributions are the best approach for modelling error in measurements obtained from instruments. In laboratory experiments or engineering environments, the dimensions become randomized by associated factors such as the instrument’s precision, ambient variables, and human error. Measurement errors arise mainly due to imperfect knowledge and carelessness on part of the experimenter, which requires to be understood in order to estimate error frames and improve the precision of the measurements.
In Reliability Engineering
Continuous probability distribution is one of the fundamental concepts in the reliability engineering area where engineers are tasked with the analysis of the system failure probability with an equal time elapse. The life times of mechanical and electrical components are built to follow an exponential distribution or Weibull distribution, which are the general distributions. With the fitting of these distributions to failure data, engineers are able to find key parameters including the mean time to failure; from such, they predict the reliability of systems.
In Control Systems
Control theory implicates continuous probability distributions to capture uncertainties present in the system dynamics together with the measurements obtained from sensors. For example such kinds of techniques as Kalman filtering and optimal control algorithms regularly deal with [real number] Gaussian distribution being preferable to describe the system [outputs] estimation error and measurement noise.
Therefore, engineers can develop sturdy control techniques that consider problems of uncertainties are numerous in the field of operations.
In Random Vibrations and Structural Analysis:
In structure engineering and mechanics, continuous probability distribution is applied to investigate the random vibration and load variations induced on structures. In this case, the job of the engineer is simplified by the use of distributions such as Gaussian, log-normal, among others, to describe the statistical behavior of variables like stress, displacement, and size of the load. Designers thus, will be able to determine structural reliability, evaluate fatigue life, and better design resilient structures.
In Thermodynamics and Statistical Mechanics:
Descriptions of molecular velocities, energies, and states of particle in the gas, liquids and solid phase are employed for continuous distribution, especially the cases of the Maxwell-Boltzmann distribution and the Bose-Einstein distribution. These distributions indicate the common occurrences in the processes such as heat exchange, diffusion, and phase changes, making us able to precisely comprehend and engineer thermal devices and materials.
Demography and Social Sciences
In demography and the social sciences, continuous probability distributions are essential for modeling various phenomena and making predictions. Here are some key applications:
In Population Growth Models
- Modeling of population growth rates over a period of time via the continuous distribution is done using an exponential distribution.
- These scenarios give demographers insight into how populations evolve in terms of their magnitude and structure, the data which is essential for the forecasting population changes in the future.
In Life Expectancy and Mortality Analysis
- Reverse distributions – say the Weibull distribution or the Gompertz distribution – are utilized to illustrate the lifespan distribution within populations.
- They act as research tools, which allow for an analysis of mortality rate and life expectancy, which are two key indicators of a population population’s health and well-being.
In Income Distribution Analysis
- Two types of distribution, namely, the Pareto distribution, and the log-normal distribution are all used to model within-population income distributions.
- Economists and social scientists learn to analyze income distribution patterns among their communities, such as existing social inequalities, different levels of impoverishment, and wealth disparities.
In Educational Achievement and Testing
- Standardized tests and academic attainment are frequently modelled by continuous distributions, such as the normal distribution.
- These types of models do the work of evaluating students’ performance, setting standards for success, and assessing the effectiveness of the educational interventions on educators and policymakers performance.
Healthcare and Biology
Continuous probability distributions play a significant role in healthcare and biology for modeling various biological processes, analyzing experimental data, and making predictions. Here are some specific applications:
In Drug Dosage and Pharmacokinetics
- It is continuous probability distributions, that model the pharmacokinetic parameters as drug absorption rates, distribution volumes and clearance rates. This information allows caregivers to tailor drug dosages for singular patients with relevant factors into consideration, such as age, weight, and those that are involved in body metabolism.
- Use of exponential distribution is wide spread in modeling drug removal rates, which help to forecast the time given for a drug to be eliminated from the body.
In Disease Risk Assessment and Epidemiology
- Recurring probability distributions are introduced with the aim of capturing and modelling the distribution of risk factor of diseases at the population level. An instance of this would be the normal distribution utilized to measure blood pressure or cholesterol levels population-wide.
- In epidemiologic investigations, when dealing with the distributions, the continuous ones are usually used to estimate the spread of diseases, their progression or disease onset times and the corresponding risk factors so as to assess the prevalence of the disease and the outcome.
In Biological Processes and Lifespan Distribution
- Intervals distribution forms the foundation that describes various phenomena of biological nature, e.g. population lifespan distribution. However, in other cases, as the Weibull distribution being based on that of the survival probabilities is used to model lifespan distributions of the certain demographic groups.
- While continuous distributions are also in place for growth process of organisms (height or weight of entities within same population) and so better appreciation is cultivated on growth and development as well as populations health.
In Genetics and Molecular Biology
- Use of continuous probability distributions in genetics can be observed for calculation of frequency of alleles among populations, this is a result of it being related to understanding of genetic diversity and inheritance paths.
- In molecular biology, typically used are continuous distributions, to represent the distribution of gene expression levels or the protein packet that is spread across cells or tissues, which in turn help analyze the regulation of genes and cell processes.
Quality Control and Manufacturing
Continuous probability distributions play a crucial role in quality control and manufacturing processes by helping to model and analyze various aspects of product quality. Here are some specific applications:
In Defect Analysis
- Continuous distributions are used to model characteristics such as material strength or surface finish quality.
- Through examining a product’s defect distribution, manufacturers can uncover repetitive defect patterns that will help them to address the problem areas. Such as, the article claims that defects may be along the line of a specific continuous distribution (the mathematical expression of which can be used to anticipate the defect rate and take preventive action in advance).
In Process Control
- During the routine process control of the manufacturing system, continuous probability distributions are used for the supervision and maintenance within the quality standard.
- Control Charts, like X−bar and R charts, examine variations of process outputs using continuous distributions that abide by the normal distribution as the basis of their assessment. Slightly different from the ideal cases may provide an indication that the production is sub-optimal and should be adjusted accordingly.
Reliability and Failure Analysis
- Continuous distributions aides with reliability and failure rates of various manufactured parts or products.
- Engineers respond with distributions like exponential distribution, which delivers the time the components fail. Through the failure data analysis, manufacturers can calculate reliability indicators, including MTBF and the maintenance approach which is dedicated to leading to the less possible downtime.
Conclusion
In conclusion, continuous probability densities are the tools used to model the behavior of continuous random variables. They do so through probability density functions (PDFs), which provide probability descriptions over the infinite range of values, without leaving gaps between them.
FAQs on Applications of Continuous Probability Distribution
What is a continuous probability distribution?
Continuous probability distribution is a statistical concept used to describe the likelihood of continuous random variables taking on various values within a specified range. It uses probability density functions (PDFs) to assign probabilities to intervals of values.
What are some examples of continuous probability distributions?
Examples include the normal distribution, uniform distribution, exponential distribution, and beta distribution. Each distribution has its own PDF that characterizes the probability distribution of the associated random variable.
How are continuous probability distributions applied in real life?
Continuous probability distributions find applications in various fields such as finance, physics, demography, healthcare, and manufacturing. They are used for tasks such as modeling stock prices, analyzing structural integrity, predicting population growth, and controlling manufacturing processes.
What are the key characteristics of continuous probability distributions?
Key characteristics include infinite possible values within a specified range, the use of probability density functions (PDFs), absence of gaps between values, determination of probabilities through integration, and well-defined mean and variance.
Why are continuous probability distributions important?
Continuous probability distributions are important because they provide a framework for understanding and predicting the behavior of continuous random variables. They are used extensively in statistical analysis, modeling real-world phenomena, and making informed decisions across various domains.
Share your thoughts in the comments
Please Login to comment...