Causation is indicating that X and Y have a cause-and-effect connection with one another. It tells X causes Y. Causation is also understood as a basis. Firstly, causation indicates that two possibilities occur at the same time or one after the other. And secondly, it tells these two variables not only occur jointly, the presence of one drives the other to display.
Correlation is a word in statistics that directs to the extent of the connection between two unexpected variables. So the correlation between two data groups is the part to which they compare one another. If X and Y grow to be observed at the exact period, it is suggesting a correlation between X and Y. It is not suggesting A rationales B or vice versa. It just tells when X is followed, Y is observed. They proceed jointly or display at the exact time.
Difference between correlation and causation
Comprehending the difference between correlation and causation can make a huge difference – particularly when someone is establishing a decision on something that may be wrong. Say, someone is wondering whether the previous month’s in monthly vibrant users has been generated by the current App Store optimization actions, it makes reason to try this in directive to say for sure whether it’s a correlation or causation.
Correlation represents an alliance between variables: when one variable is different, so do the other. A correlation is a statistical hand of the connection between variables. These variables vary jointly: they covary. But this covariation isn’t necessarily due to an immediate or avoiding causal connection.
Causation means that changes in one variable bring about changes in the other; there is a cause-and-effect relationship between variables. The two variables are associated with each other and there is also a causal connection between them. A correlation doesn’t indicate causation, but causation always indicates correlation.
Types of Correlation
- Positive correlation: An optimistic correlation is when you watch X increasing and Y increasing as well. Or if X drops, Y likewise drops.
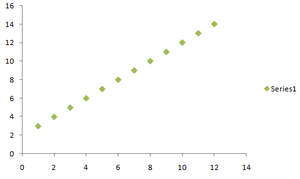
- Negative correlation: A negative correlation is when an increase in X directs to a decrease in Y or vice versa.
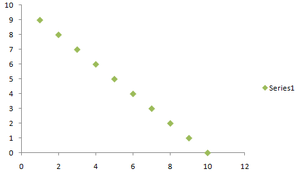
- No correlation: No correlation is when two variables are totally irrelevant and a difference in X shows no differences in Y or vice versa.
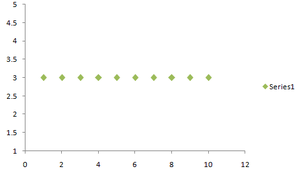
Correlation Vs Causation: How to Tell if Something’s a Coincidence or a Causality
So how does someone try the data so one can make bulletproof assertions about causation? There are five methods to go about this – technically they are called the method of investigations. (Document them from the most powerful technique to the most helpless).
- Randomized and Experimental Study
Circumstances people like to test the unique shopping coach in their eCommerce app. The opinion is that there are multiple phases before a user can even review and expand for their thing and that this situation is a controversial topic that prevents them from buying more often. So they have rebuilt the shopping carriage in their app and like to visit if this will increase the chances of users buying goods.
The most convenient way to establish causation is to organize randomized research. This is where one randomly assigns people to try the testing organization.
In the practical method, there is a managing enterprise and a practical group, both with matching conditions but with one independent variable being tested. By assigning someone randomly to try the testing corporation, you avoid trying appreciation, where detailed outcomes are chosen over others.
In the sample, one would randomly assign users to try the new shopping carriage one has prototyped in one’s app, while the administration group would be appointed to use the existing (old) shopping cart.
After the testing time, look at the data and see if the new cart guides to more investments. If it does, one can assert a true causal connection: one’s old cart was restricting users from completing a purchase. The outcomes will have the most facts to both interior stakeholders and other individuals beyond one’s association whom one decides to share it with, specifically because of the randomization.
But what occurs when someone can’t randomize the method of assigning users to take the analysis? This is a quasi-experimental method. There are six kinds of quasi-experimental methods, each with different applications. The issue with this technique is, without randomization, statistical difficulties become pointless. People cannot be absolutely sure the outcomes are due to the variable or to problem variables carried about by the lack of randomization.
Quasi-experimental analyses will typically require more advanced statistical procedures to get the necessary insight. Researchers may use surveys, interviews, and observational notes as well – all complicating the data analysis process.
Let’s say people are testing whether the user experience in your latest app version is less confusing than the old UX. And people are specifically using their closed group of app beta testers. The beta test group wasn’t randomly selected since they all raised their hand to gain access to the latest features. So, establishing correlation Vs causation – or in this instance, UX compelling disorder– isn’t as detailed as when using a randomized testing analysis.
While scientists may ignore the effects from these analyses as untrustworthy, the information people collect may even give you a good understanding (think trends).
A correlational study is when people attempt to resolve whether two variables are connected or not. If X grows and Y likewise grows, that is a correlation. Just determinate that correlation doesn’t indicate causation and it will be okay.
For instance, people choose to desire to try whether a softer UX has a powerful favorable correlation with more useful app stock ratings. After observation, people see that when one rises, the other does too. They are not telling X (smooth UX) causes Y (sounder ratings), They are telling X is deeply associated with Y, and maybe energy even anticipates it. That’s a correlation.
The single-subject method is more frequently used in psychology and teaching, as it is involved with a unique topic. Rather of control and testing the company, the issue acts as his or her own authority. The investigator is concerned about trying to adjust the person’s behavior or thought.
In mobile marketing, a single-subject analysis might take the form of requesting one distinct user to try the usability of new app quality. People can have them do one measure for several duration on the existing app, then have them try the duplicate measure on the new app performance. Organize the data and see if the activity is done quickly on the old or new app.
Apparently, this method is using data from one user. Their knowledge cannot be generalized to all their users no issue how excellent a fit to the perfect buyer persona. That’s one cause why this type of analysis is infrequently used in commerce.
Anecdotes, sadly, are periodical all the evidence we have to demonstrate causation. Your energy comes through Support staff: “Customers believe the new user interface is hard to use. That’s why they’re uninstalling.”Customer X on Twitter: “We attempted to purchase a product on your app and it’s causing my phone craSH!”The issue here is: while they could have a valid misery point and might make it in an effective (And highly emotional) way, these stories do not confirm without a suspicion that X causes Y. They’re actually just rumors at this moment and carry less importance than the other choices overhead.
Correlation Vs Causation in Mobile Analytics
So what have we realized from all these correlation and causation instances? There are methods to try whether two variables generate one another or are positively correlated to one another. If they are severe about showing a causal connection, then they have got to use the testing process that shows their data and outcomes in the most facts. Begin with the unexpected experimental procedure and perform their course downwards. And consistently watch how they believe or even verbalize your forecasts.
There’s a Latin saying that goes: “Post hoc, ergo propter hoc,” which suggests: “After this, thus because of this.” The opinion is that by conveying one comment before the other, you indicate that the previous pushed the latter to occur. (“He rated my app zero stars. No one has downloaded my app.”) The truth is it could just be a correlation or a pure concurrency.
How to check Correlation Vs. Causation
As mobile marketers, we make judgments every day established on data. These conclusions guide users to maintain using the apps or uninstall them. This is why one must believe absolutely when encountering data and be careful with likely correlation vs causation problems.
A statistical phrase that guides to the extent of connections between 2 unexpected variables. So the correlation between 2 information sets is the amount to which they compare one another.
Sample Problems
Question 1: My father just complained to me: “While texting message, my phone keyboard freezes.” Is this problem related to causation or correlation?
Answer:
A short look at his smartphone affirmed my doubt: there are four-game apps open at the same time plus WhatsApp and YouTube. The action of attempting to send a text message wasn’t generating the freeze, the lack of RAM was. But my father instantly related it with the last step he was doing before the freeze.
Father was indicating causation where there was only a correlation.
Lack of RAM ⇢ Causation ⇢ Phone Freez ⇢ Correlation ⇢ Text Messaging won’t Work
Question 2: On daytime where Mrs. Roy goes jogging, Mrs. Roy witnesses more autos on the road.“Is this a cause-and-effect relationship is authorized or unauthorized?
Answer:
Mrs, Roy personally, not Compelling more autos to drive outdoors on the road when she goes jogging. It’s just that because she goes jogging outside, Mrs, Roy sees more autos than when she stays at home. This connection is not cause-and-effect because of neither the autos nor she affects each other.
Question 3: On days when Mr. chhabra drinks tea, Mr. chhabra sense more energetic“ Is this is cause-and-effect relationship is authorized or unauthorized?
Answer:
Mr, chhabra can sense more energy because of the tea, sure. But it can also be because Mr, chhabra goes to the tea shop to drink tea, and Mr, chhabra is more energetic at the tea shop than at home when there are a million distractions. This cause-and-effect IS NOT authorized.
Question 4: After Mr, Sagar’s exercise, Mr, Sagar feel physically exhausted. Is this cause-and-effect relationship is authorized or unauthorized?
Answer:
This is cause-and-effect because Mr, Sagar intentionally forces his body to physical tiredness when doing exercise. The strengths Mr, Sagar used to exercise are exhaustion (effect) after he exercise (cause). This cause-and-effect is authorized.
Question 5: When Mrs, Puja feeds her dog more than 3 treats a day, Her dog gets a little heavier, Is this is a cause-and-effect relationship?
Answer:
Mrs, Puja’s dog gets heavier because she is feeding it more. This is a cause and effect. Mrs, Puja feeding her dog treats is the cause and the effect is her dog becomes a little heavier.
Share your thoughts in the comments
Please Login to comment...